Improved Chemical Reaction Optimization With Fitness-Based Quasi-Reflection Method for Scheduling in Hybrid Cloud-Fog Environment
IEEE TRANSACTIONS ON NETWORK AND SERVICE MANAGEMENT(2024)
摘要
With the advent of Internet of Things (IoT) applications, smart IoT devices are ubiquitous. Executing these devices on cloud data centers can lead to network congestion and transmission delay that causes failure instances in cloud architecture. Therefore, fog computing is derived to satisfy the low latency, location awareness, and mobility requirements of massive IoT applications near end users. Concerned with the high requirements of compute-intensive business applications, fog computing structure becomes complex due to limited computing capacity, and therefore these applications are offloaded to the cloud. Therefore, to overcome the offloading problem of workflow tasks in this work, a novel method named: Improved Chemical Reaction Optimization for Workflow Scheduling in a Hybrid Environment (ICRO-WSHE) has been proposed. The proposed algorithm aims at minimizing the execution cost of workflow applications under the defined deadline constraints. The algorithm is based on CRO, which has fewer parameters and the fastest convergence speed. However, the existing CRO has drawbacks in terms of getting stuck in the local optimum and having difficulty obtaining real optimal solutions. Therefore, the proposed mechanism modifies the existing CRO algorithm and includes the suitable features of PSO (Particle Swarm Optimization) and FQR (Fitness-based Quasi Reflection) methods to prevent the shortcomings of the CRO method. Further, a double-point synthesis operation is incorporated to increase the exploration rate and improve the working of the proposed algorithm. The result of the simulation experiment based on different types of workflows shows that the proposed method outperforms existing algorithms and proves to be a practical approach.
更多查看译文
关键词
Chemical reaction optimization,fitness-based quasi reflection method,opposition based learning,workflow scheduling,cloud computing,fog computing
AI 理解论文
溯源树
样例
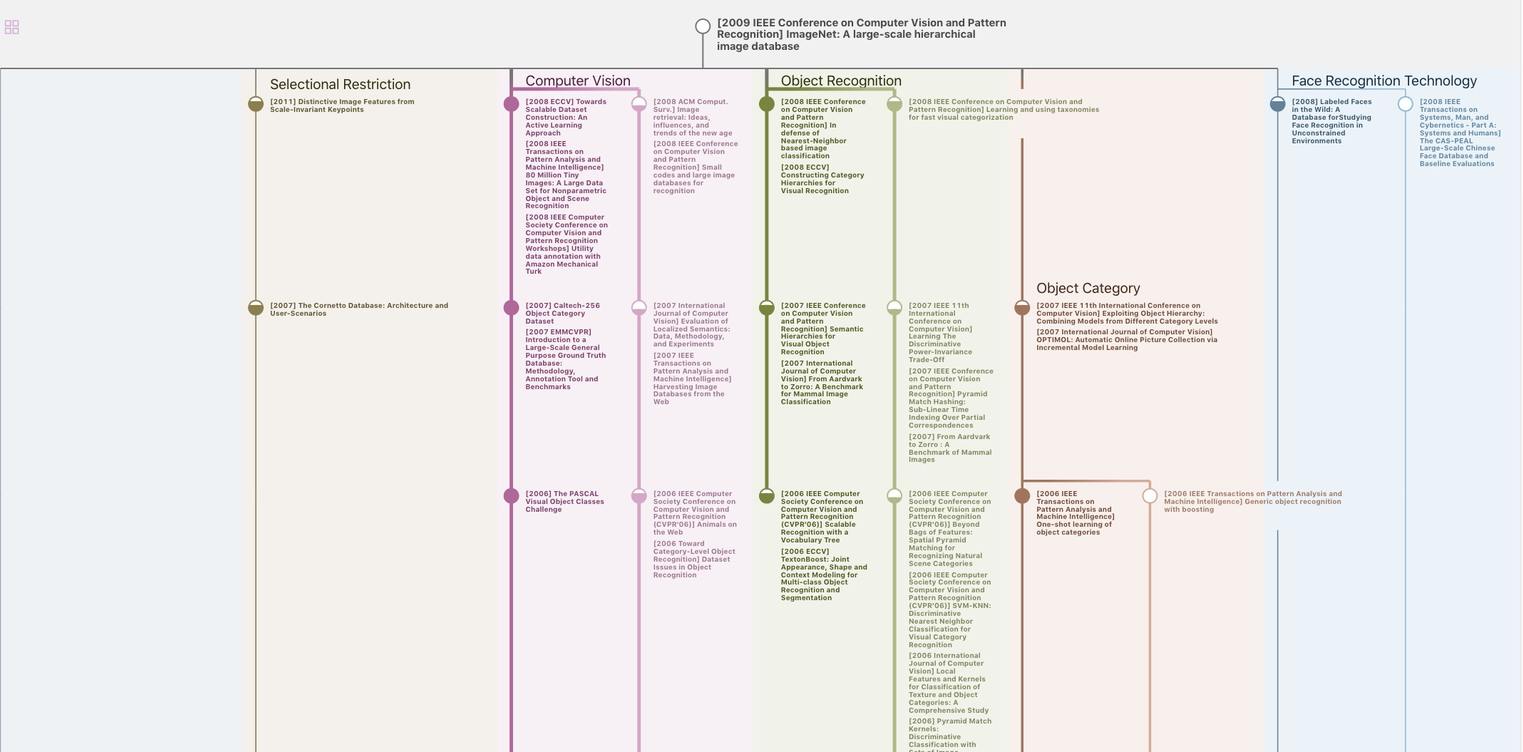
生成溯源树,研究论文发展脉络
Chat Paper
正在生成论文摘要