Data Collection Algorithms for Model Training in Internet of Vehicles.
IEEE Internet Things J.(2024)
摘要
In Internet of vehicles (IoVs), it is critical to collect sufficient data for model training, to support vehicular intelligent applications. However, the environment of IoV is highly dynamic due to the mobility of vehicles, making it challenging to efficiently allocate resources for data collection. Additionally, timely training of machine learning models with collected data is important for accurate representation in a constantly changing environment. This paper aims to improve the performance of model training by collecting sufficient data from vehicles in IoV. A system throughput maximization problem is formulated under the limited bandwidth, storage and computing resources, which is an NP-hard problem. To solve the problem, an iterative algorithm, namely IAMB, is proposed to preferentially collect data from the vehicles with sufficient data and reliable communication quality. Besides, a genetic algorithm, namely GAMB, is proposed to further improve the probability of superior individual by replacing operation. We also customize three greedy strategy based algorithms as the baselines. Extensive experimental results show that our proposed algorithms outperform the baseline algorithms for all cases. Specifically, IAMB and GAMB can improve the throughput by up to 5% and 8%, respectively, compared with baseline algorithms. In addition, the customized genetic algorithm is also superior to the iterative algorithm on performance of system throughput. Moreover, the customized genetic algorithm is more stable than the proposed iterative algorithm in terms of system throughput for model training in the dynamic network environment.
更多查看译文
关键词
Data Collection,Throughput Maximization,Internet of Vehicle
AI 理解论文
溯源树
样例
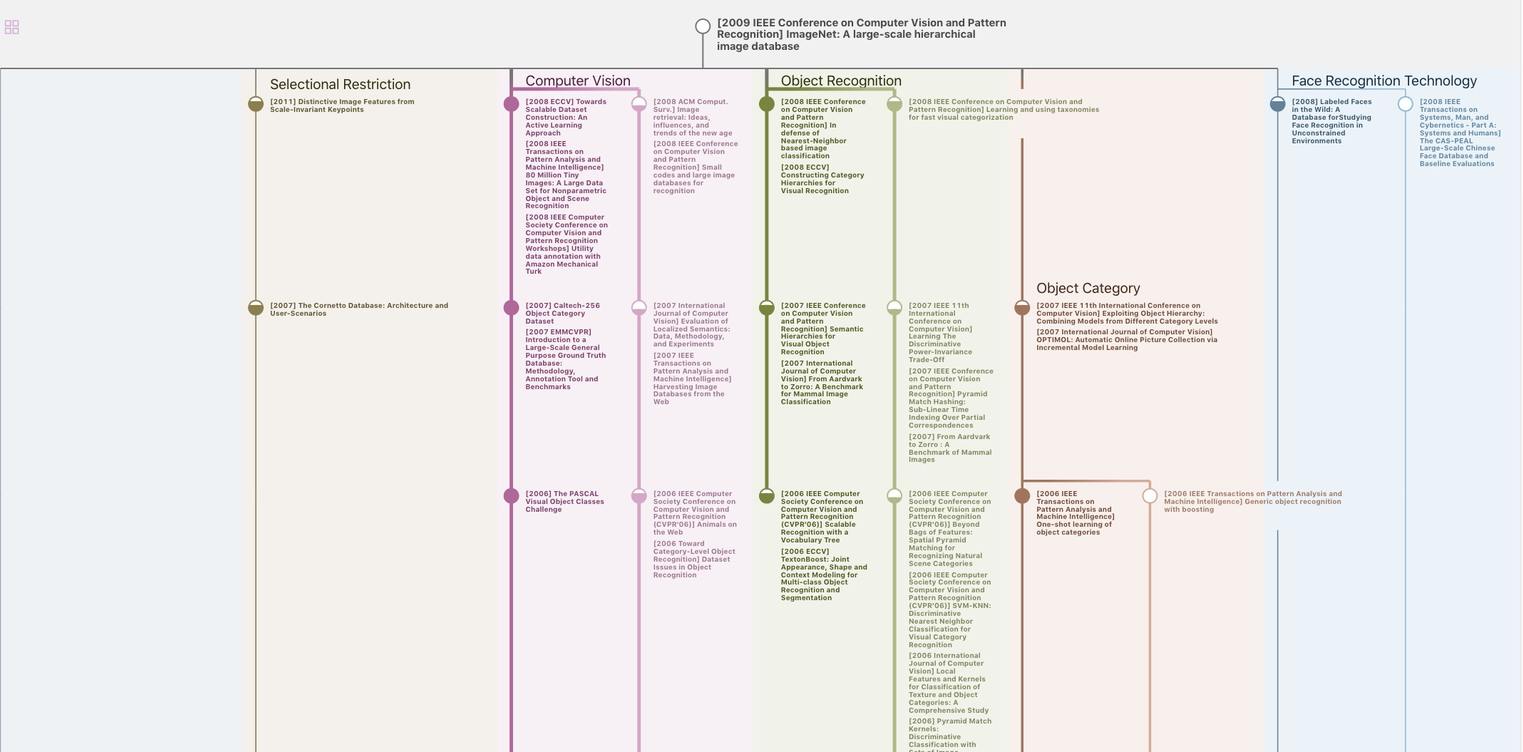
生成溯源树,研究论文发展脉络
Chat Paper
正在生成论文摘要