Free-Form Dynamic Load Model Synthesis With Symbolic Regression Based on Sparse Dictionary Learning
IEEE Transactions on Power Systems(2023)
摘要
With the increasing integration of distributed energy resources, significant efforts have been made to develop more accurate load models to mimic the dynamic response of power system load. Despite the prevalence of high-resolution measurement equipment and artificial intelligence algorithms, the increasing complexity in existing load models has restricted their accuracy, effectiveness, and capability in real applications. In this paper, a symbolic regression-based method combined with sparse dictionary learning is developed to automatically construct a free-form dynamic load model by synthesizing a large number of basic physics-driven mathematical functions. Firstly, the discontinuities or jumps of the dynamic load time-series data are automatically detected using a discrete wavelet transform. Then a sparse representation of the complete mathematical function set, including all possible physics-driven mathematical functions, is selected using a sparse dictionary learning algorithm. Furthermore, the formulated free-form load model parameters are initialized based on the data itself instead of being manually defined. Various tests are performed with different categories of faults at different locations to validate the performance of the proposed model.
更多查看译文
关键词
Dynamic load model,free-form,physics-driven,sparse dictionary learning,symbolic regression
AI 理解论文
溯源树
样例
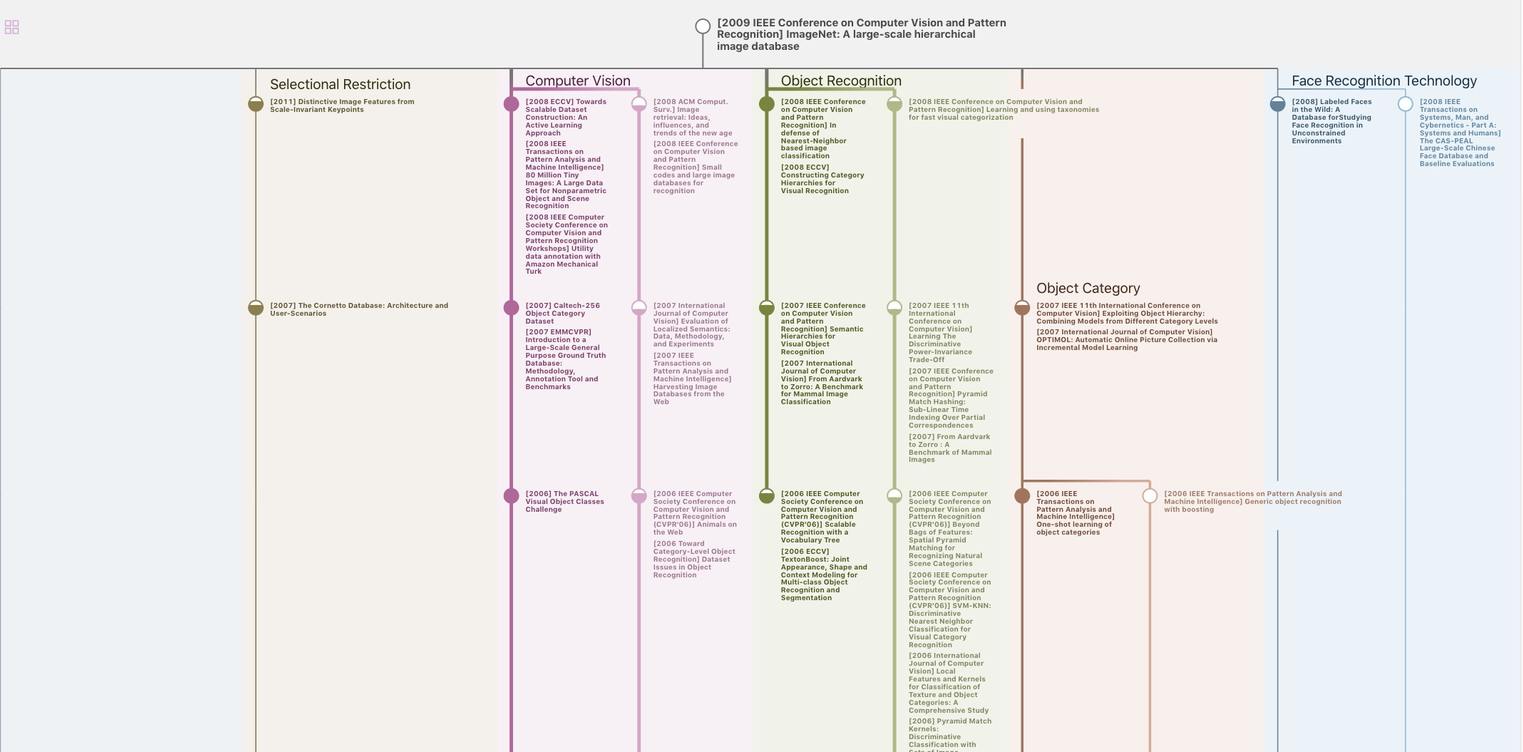
生成溯源树,研究论文发展脉络
Chat Paper
正在生成论文摘要