Geo-Perturbation for Task Allocation in 3-D Mobile Crowdsourcing: An A3C-Based Approach.
IEEE Internet of Things Journal(2024)
摘要
Location privacy protection (LPP) has become a key concern during mobile crowdsourcing (MCS) task allocation. Existing LPP mechanisms for MCS applications mainly focus on 2-D plane scenarios or directly apply 2-D techniques into 3-D space scenarios, leaving the height dimension of 3-D geolocation vulnerable to privacy breaches. To facilitate the LPP in 3-D MCS, we propose a learning-based geo-perturbation mechanism using 3-D geo-indistinguishability (3D-GI). In this mechanism, we first define an optimization objective to balance location privacy and MCS server profit, making it adaptable to different types of MCS applications. Then, we adopt the asynchronous advantage Actor–Critic (A3C) algorithm to design a reinforcement learning (RL)-based approach without knowing the accurate system and attack models. This approach enables us to derive the optimal perturbation policy in continuous policy space and accelerates the learning speed using asynchronous multithread training. Simulation results demonstrate that the proposed mechanism can better balance location privacy and server profit in 3-D MCS applications compared to existing benchmarks.
更多查看译文
关键词
Geo-indistinguishability,location privacy protection (LPP),mobile crowdsourcing (MCS),reinforcement learning (RL),3-D space
AI 理解论文
溯源树
样例
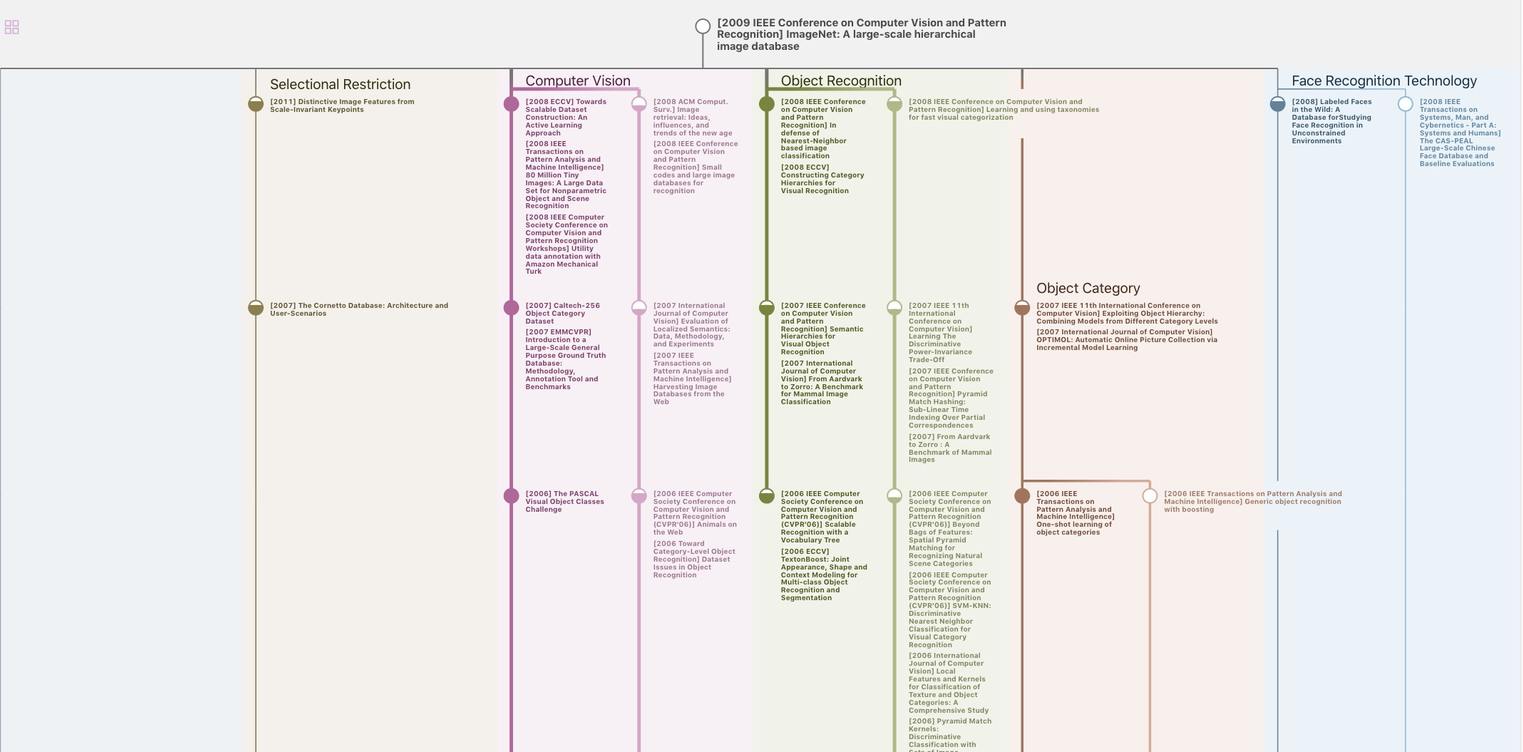
生成溯源树,研究论文发展脉络
Chat Paper
正在生成论文摘要