Robust Enhanced Collaborative Filtering Without Explicit Noise Filtering
Research Square (Research Square)(2023)
摘要
Abstract Graph convolutional neural networks have been successfully applied to collaborative filtering to capture high-quality user–item representations. Despite their remarkable performance, there are still limitations that hinder further improvement of recommender systems. Most existing recommender systems utilize implicit feedback data for model training; however, this approach inevitably introduces adversarial interaction noise. The conventional graph-based collaborative filtering method fails to effectively filter out this noise and, instead amplifies its impact, resulting in degraded model performance. To address this issue, we propose a robustness-enhanced collaborative filtering graph neural network model that does not rely on explicit noise filtering. Our approach involves simulating user–item interactions that do not exist in practice as adversarial interaction noise using random noise. To mitigate the impact of this noise in hidden feedback, we replace them with randomly selected partial nodes based on the principle of mutual information maximization. This approach not only improves model performance but also enhances the robustness of the model. Through experimental demonstrations on three benchmark datasets, our model exhibits significant improvement, thereby validating the effectiveness and interpretability of our proposed approach.
更多查看译文
关键词
robust enhanced collaborative filtering
AI 理解论文
溯源树
样例
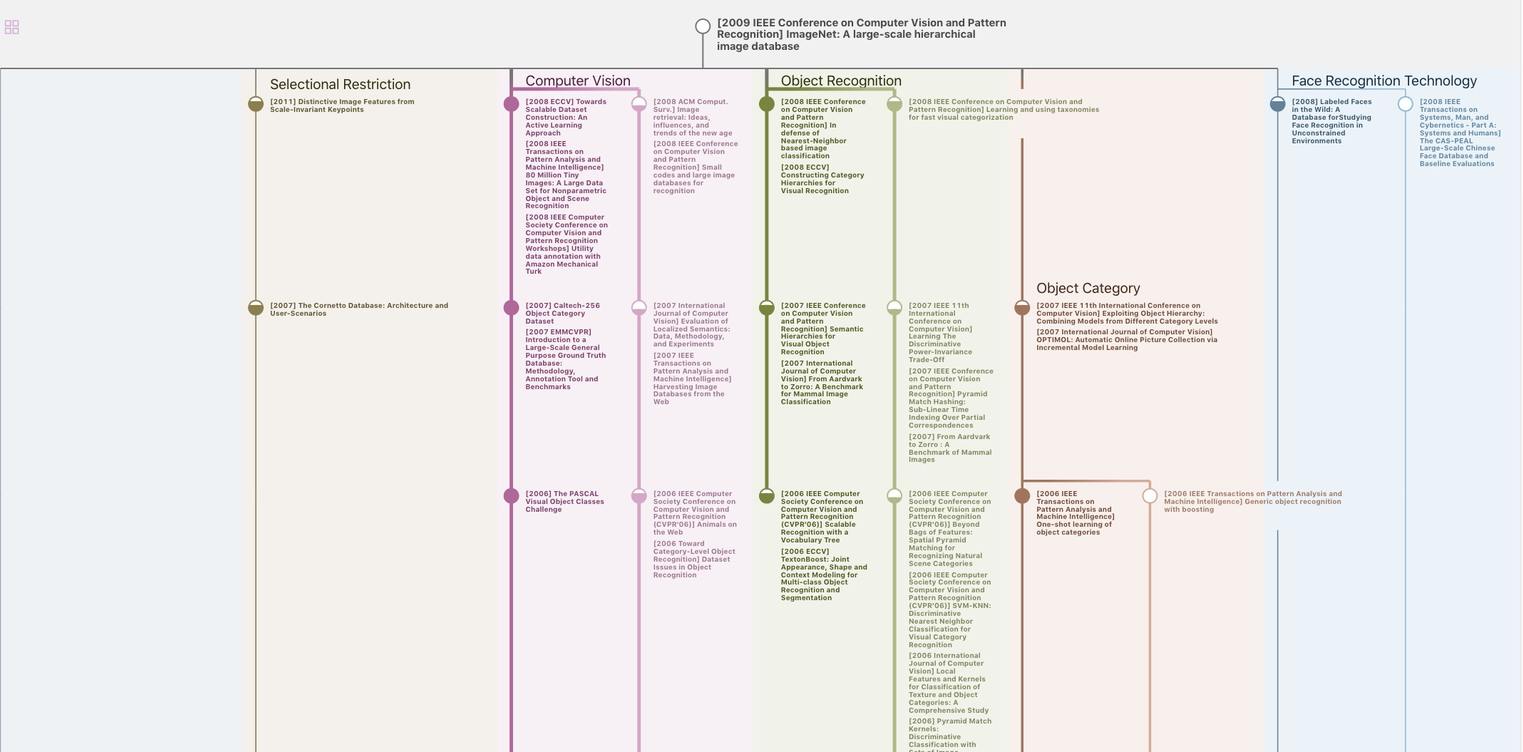
生成溯源树,研究论文发展脉络
Chat Paper
正在生成论文摘要