Blind Image Quality Assessment via Deep Response Feature Decomposition and Aggregation
IEEE JOURNAL OF SELECTED TOPICS IN SIGNAL PROCESSING(2023)
摘要
Image quality is related to image content and distortion information. Most learning-based image quality assessment (IQA) methods extract quality-oriented features with auxiliary tasks like detecting the distortion type and level. However, the perceptual quality degradation caused by the same distortion type and level varies substantially for different content in an image. To deal with this problem, in this article, we propose a blind IQA method based on Deep Response fEAture decoMposition and aggregation (DREAM), which considers two factors affecting the image quality simultaneously. First, we use a convolutional neural network (CNN) to extract the basic features from the input image. Second, several parallel fully connected (FC) layers are employed to decompose these basic features into response features related to the image content, distortion type, and distortion level. Third, the graph attention network (GAT) is leveraged to aggregate these response features corresponding to the visual quality. Finally, a regression network is used to predict the quality score. The success of our method lies in the feature decomposition to obtain the response features of different content to a specific distortion in the given distorted image and the quality-oriented features obtained by feature aggregation using the internal relation of these response features. Experimental results indicate that our proposed DREAM achieves state-of-the-art (SOTA) performance on both synthetic and authentic distortion IQA datasets.
更多查看译文
关键词
Blind image quality assessment,feature decomposition,feature aggregation,graph attention network,convolutional neural network
AI 理解论文
溯源树
样例
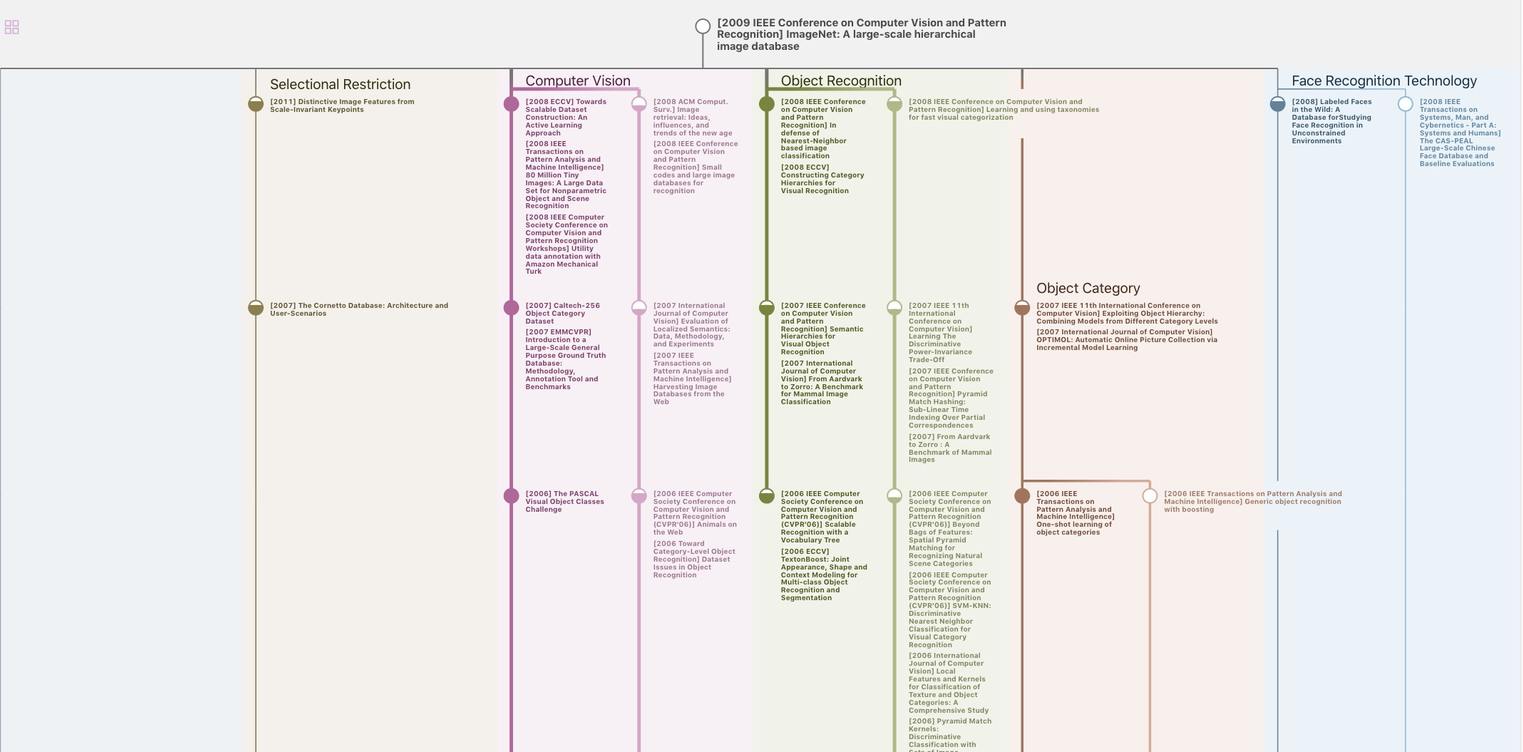
生成溯源树,研究论文发展脉络
Chat Paper
正在生成论文摘要