Intersection analysis using computer vision techniques with SUMO
Intelligent Transportation Infrastructure(2023)
摘要
Abstract This paper presents intersection analysis using computer vision techniques with Simulation of Urban MObility (SUMO). First, an efficient deep-visual tracking pipeline is proposed by using the off-the-shelf YOLO object detection architecture and cascading it with a discriminative correlation filter to produce reliable trajectories for traffic analysis of vehicles and pedestrians. While a variety of traffic measurements can be directly estimated from the extracted trajectories (e.g., speed, turning movement count), a method of incorporating turning movement count (TMC) within SUMO is proposed in order to mimic a realistic traffic flow for an observed intersection and its comprehensive analysis. Experimental evaluations on the developed tracking system implies that the YOLOv5 variant is the best for traffic cameras and, after appropriate fine-tuning using the University of Nevada, Las Vegas pedestrian data set, the YOLOv5 performance manifested a significant improvement with a recall value of 0.62. The tracking system is further employed for monitoring three other intersections in the downtown area of Las Vegas and turning movement counts were estimated for peak hours in the morning and evening of one day (7:00–9:00 and 16:00–18:00) at 15-min intervals. Finally, the intersection design, including traffic signals with estimated TMC, is used to calibrate SUMO to provide critical parameters (e.g., lane density, travel time, occupancy) for traffic signal performance evaluation and comprehensive intersection analysis. The signal design treatment demonstrates a significant improvement in travel times and simulation results indicate that the turning-left ratio is a crucial factor affecting the travel time of vehicles on each intersection leg.
更多查看译文
关键词
intersection,computer vision techniques,sumo
AI 理解论文
溯源树
样例
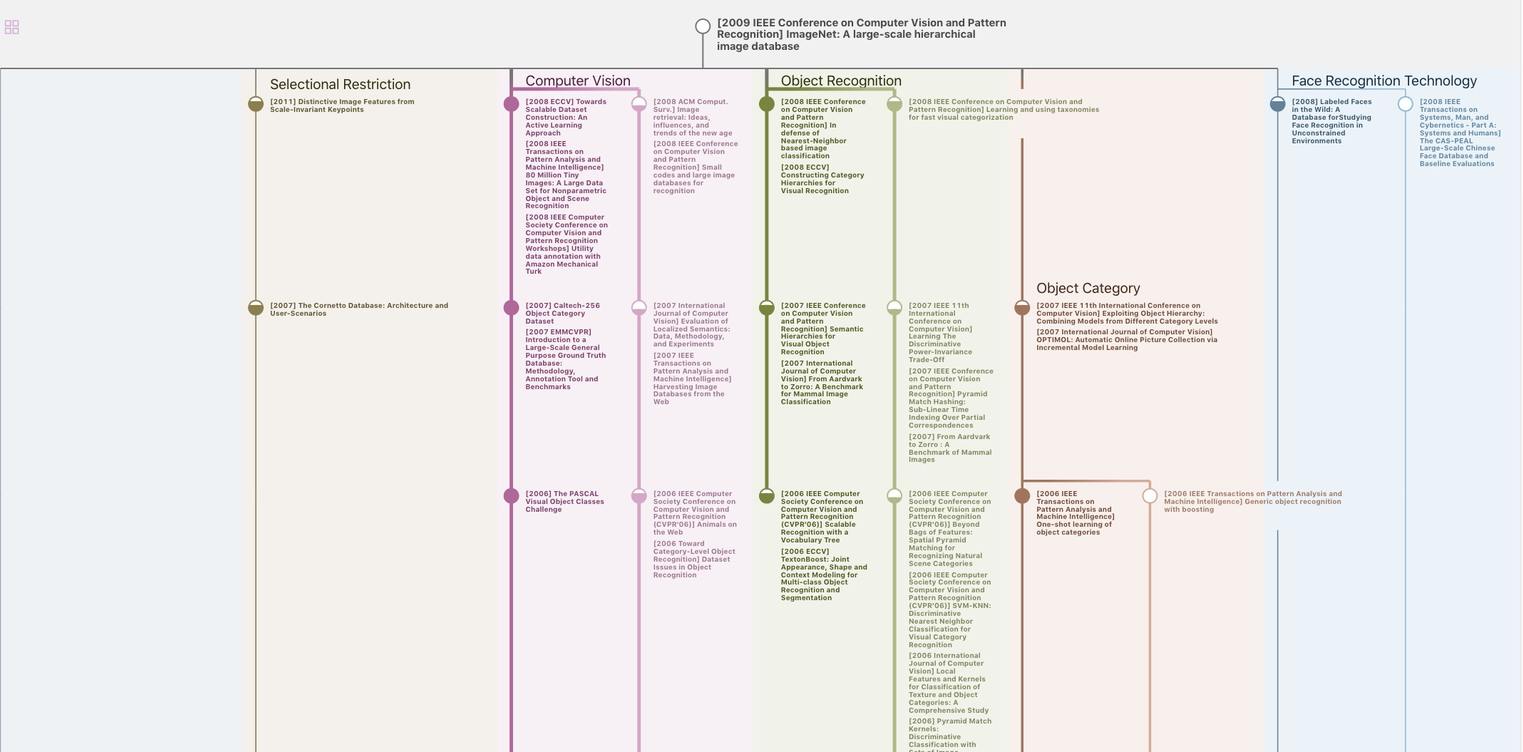
生成溯源树,研究论文发展脉络
Chat Paper
正在生成论文摘要