LSTAloc : A Driver-Oriented Incentive Mechanism for Mobility-on-Demand Vehicular Crowdsensing Market
IEEE Transactions on Mobile Computing(2023)
摘要
With the popularity of Mobility-on-Demand (MOD) vehicles, a new market called MOD-Vehicular-Crowdsensing (MOVE-CS) was introduced for drivers to earn more by collecting road data. Unfortunately, MOVE-CS failed after two years of operation. To identify the root cause, we survey 581 drivers and reveal its simple incentive model based on blindly competitive rewards. This model brings most drivers few yields, resulting in their withdrawals. In contrast, a similar market termed MOD-Human-Crowdsensing (MOMAN-CS) remains successful thanks to a complex model based on exclusively customized rewards. Hence, we wonder whether MOVE-CS can be resurrected by learning from MOMAN-CS. Despite considerable similarity, we can hardly apply the incentive model of MOMAN-CS to MOVE-CS, since MOD drivers are also concerned with passenger missions that dominate their earnings. To this end, we analyze a large-scale dataset of 12,493 MOD vehicles, finding that drivers have explicit preference for short-term, immediate gains as well as implicit rationality in pursuit of long-term, stable profits. Therefore, we design a novel driver-oriented incentive mechanism for MOVE-CS, called
LSTAloc
, at the heart of which lies a spatial-temporal differentiation-aware task allocation scheme empowered by submodular optimization. Applied to the dataset, our design would essentially benefit both the drivers and platform to incentivize MOD vehicular crowdsensing efficiently, thus possessing the potential to resurrect MOVE-CS.
更多查看译文
关键词
Incentive mechanism,submodular optimization,task allocation,vehicular crowdsensing
AI 理解论文
溯源树
样例
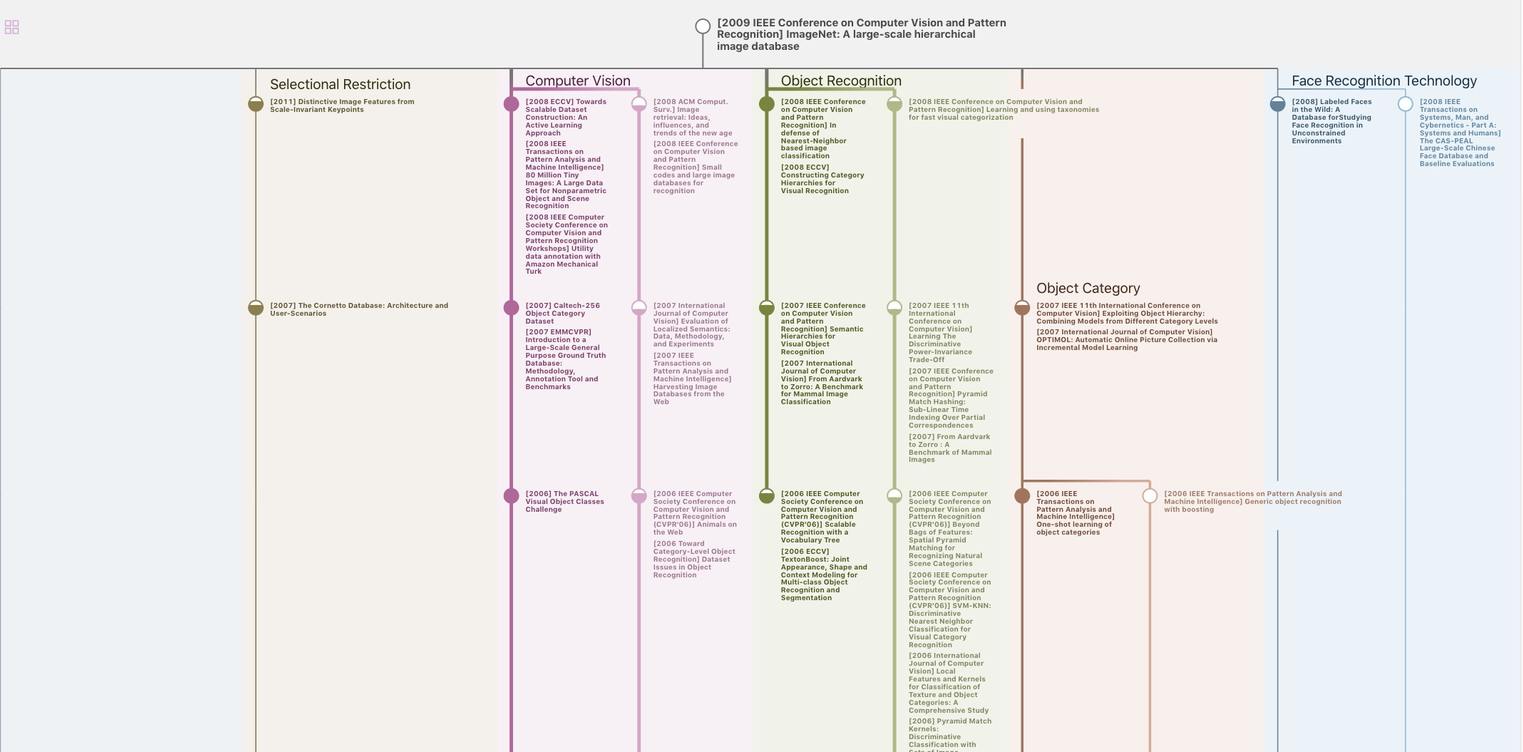
生成溯源树,研究论文发展脉络
Chat Paper
正在生成论文摘要