A Width Multi-Scale Adversarial Domain Adaptation Residual Network with AConvolutional Block Attention Module
Research Square (Research Square)(2023)
摘要
Abstract Although the fault diagnosis methods based on deep learning have attracted widespread attention in the academic field in recent years, such methods still face many challenges, including complex and variable working conditions, insufficient ability to extract key features, and large differences in sample data. To address these problems, a width multi-scale adversarial domain adaptation residual network with a convolutional block attention module (WMSRCIDANN) is proposed in this paper, which consists of a feature extraction network, a domain discriminant network, and a label classification network. In the feature extraction network, an improved width multi-scale residual network combined with a convolutional block attention module (WMSRC) is used as the feature extractor to achieve a weighted fusion of multi-depth features.In the domain discriminative network, the fully-connected network is replaced by a four-layer convolutional structure, which can further reduce the difference in feature distribution and improve the cross-domain invariance of deep features. In the label classification network, the classifier uses the extracted domain-invariant features to perform cross-domain fault identification. The experimental results show that WMSRCIDANN is effective in cross-domain bearing fault diagnosis.
更多查看译文
关键词
aconvolutional block attention module,adaptation,multi-scale
AI 理解论文
溯源树
样例
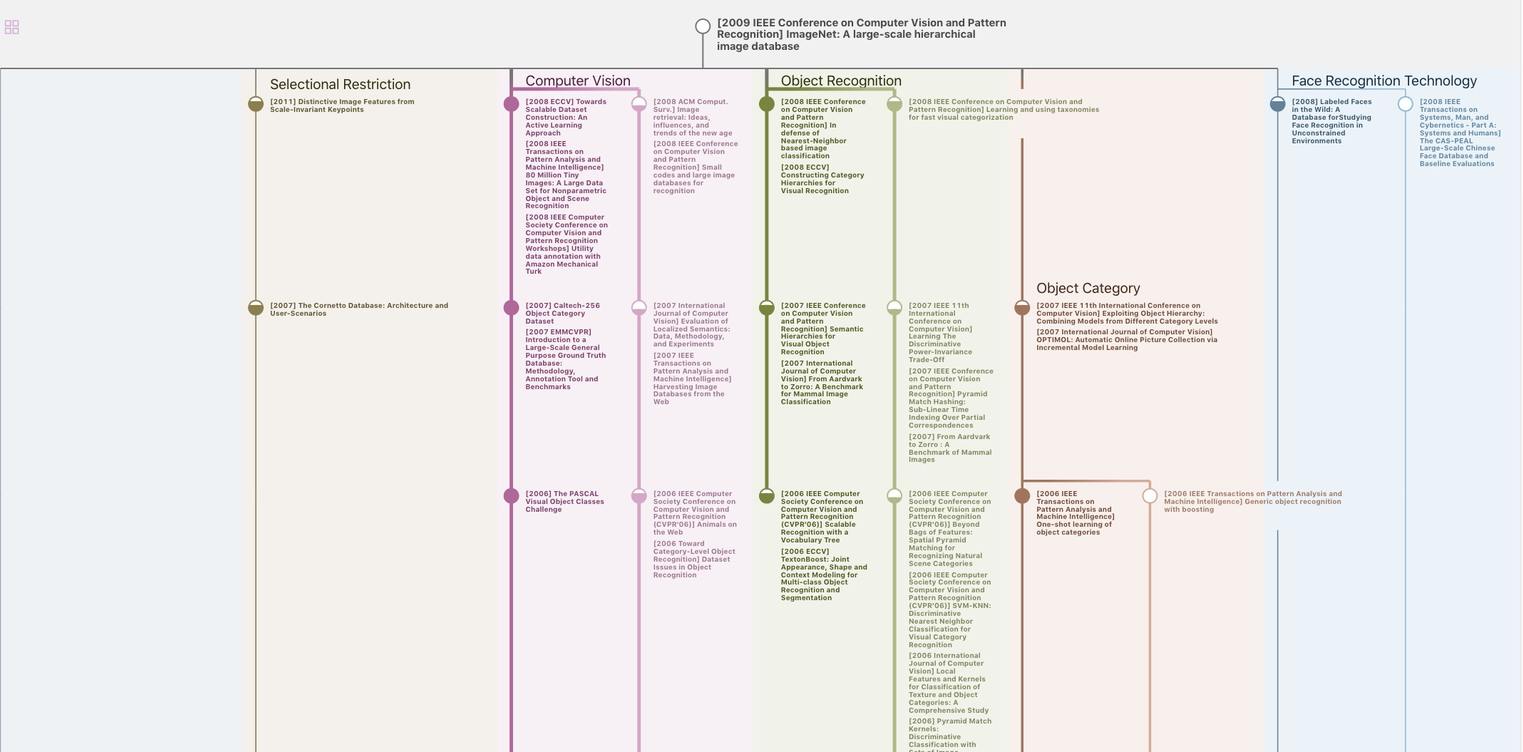
生成溯源树,研究论文发展脉络
Chat Paper
正在生成论文摘要