Sampling-based Nystr\"om Approximation and Kernel Quadrature
arXiv (Cornell University)(2023)
摘要
We analyze the Nystr\"om approximation of a positive definite kernel associated with a probability measure. We first prove an improved error bound for the conventional Nystr\"om approximation with i.i.d. sampling and singular-value decomposition in the continuous regime; the proof techniques are borrowed from statistical learning theory. We further introduce a refined selection of subspaces in Nystr\"om approximation with theoretical guarantees that is applicable to non-i.i.d. landmark points. Finally, we discuss their application to convex kernel quadrature and give novel theoretical guarantees as well as numerical observations.
更多查看译文
关键词
sampling-based
AI 理解论文
溯源树
样例
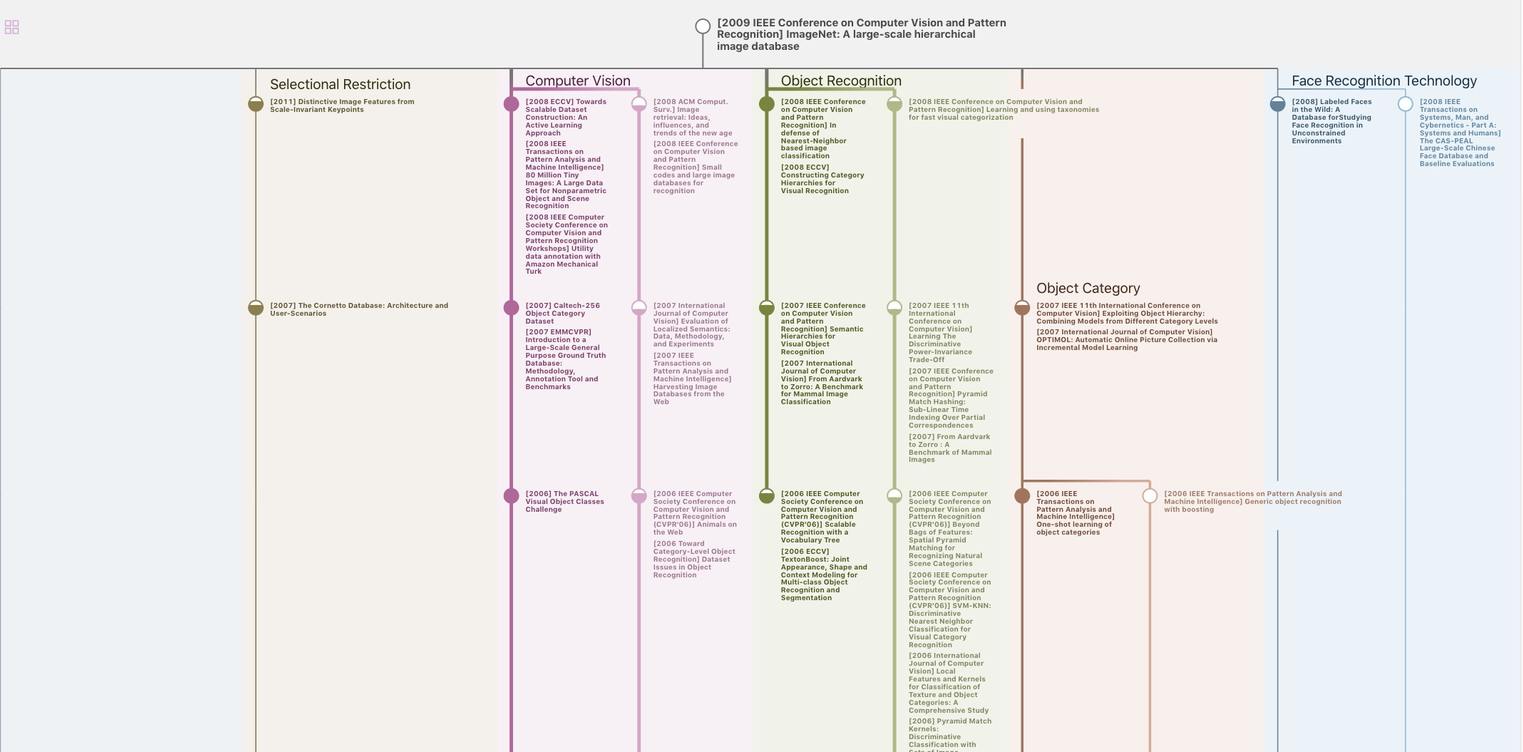
生成溯源树,研究论文发展脉络
Chat Paper
正在生成论文摘要