Hypervolume-Guided Decomposition for Parallel Expensive Multiobjective Optimization
IEEE Transactions on Evolutionary Computation(2023)
摘要
The hypervolume metric is widely used to guide the search in multiobjective optimization. However, in parallel expensive multiobjective optimization, the hypervolume-based multi-point expected improvement (EI) suffers from high computational overhead and scales poorly with the batch size. To address this issue, we integrate hypervolume-based EI with the MOEA/D framework and propose a novel EI, named the expected direction-based hypervolume improvement (DirHV-EI). The DirHV-EI only measures the hypervolume improvement within each axis-parallel box induced by the modified Tchebycheff scalarization. Thus, it has a simple analytical expression that can be easily computed. Theoretical analysis indicates that the maximization of our proposed improvement function can help to maximize both the weighted hypervolume and the Tchebycheff improvement metrics. Using DirHV-EI, we design a decomposition-based Bayesian optimization algorithm, called DirHV-EGO, for solving expensive multiobjective optimization problems. At each iteration, the MOEA/D is used to maximize the DirHV-EI values with respect to a number of direction vectors in a collaborative manner, and a number of candidate solutions can be obtained. Then, a submodularity-based greedy selection strategy is used to select multiple query points from the candidates. Experimental results on both benchmark instances and real-world problems show that our proposed algorithm is an efficient and effective method for parallel expensive multiobjective optimization.
更多查看译文
关键词
Decomposition,efficient global optimization,expected improvement,expensive multiobjective optimization,Hypervolume
AI 理解论文
溯源树
样例
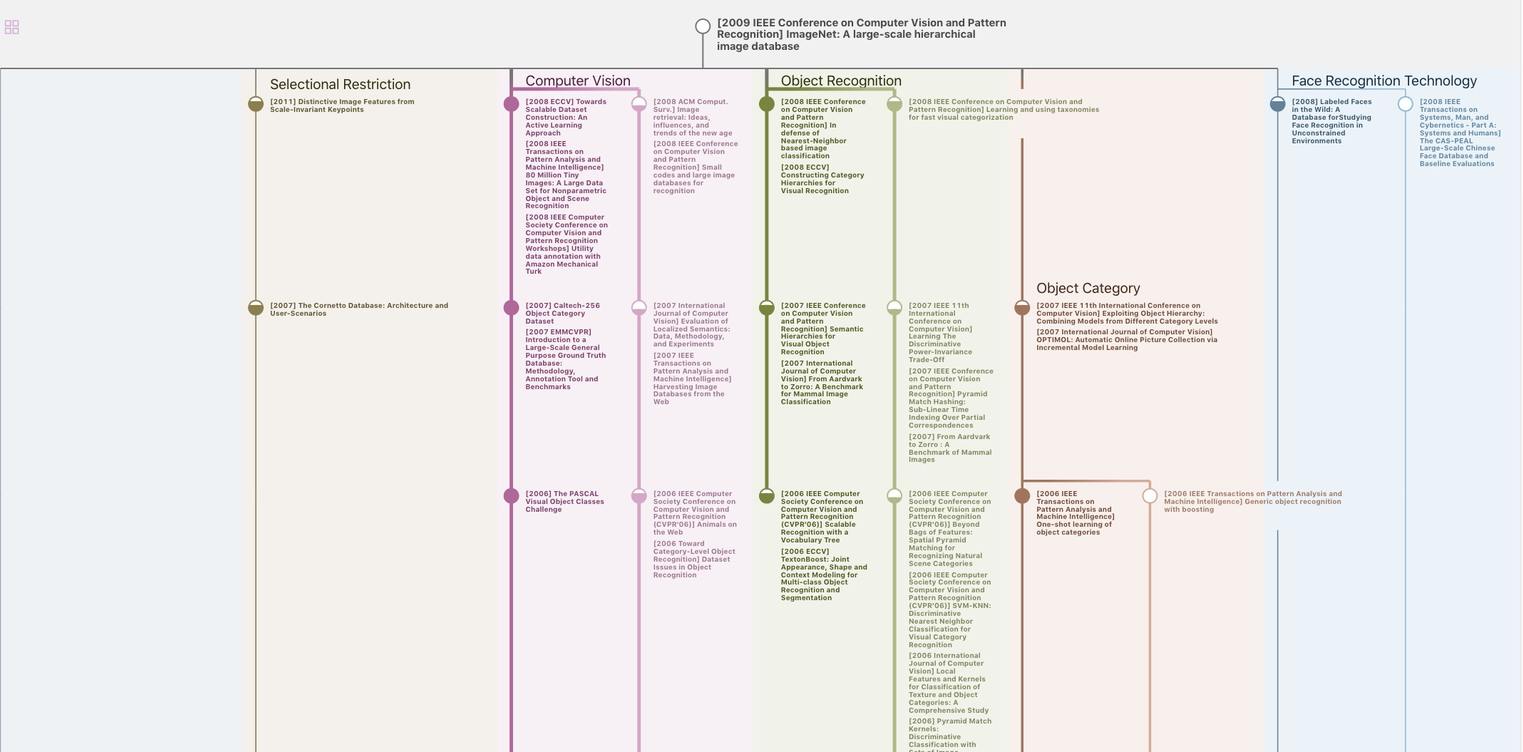
生成溯源树,研究论文发展脉络
Chat Paper
正在生成论文摘要