Performance of survival neural networks in predicting 10-year cardiovascular disease risk in UK and Chinese populations
European Heart Journal(2023)
摘要
Abstract Background Accurate risk assessment is essential for the primary prevention of cardiovascular disease (CVD). The commonly used models (e.g., WHO CVD risk charts and SCORE2) have been recalibrated using country-specific incidence to reduce the impact of population disparity. While some state-of-art machine learning algorithms have been developed to improve predictive accuracy, it is still unclear if they could accurately predict CVD risk in diverse populations. How to recalibrate these models for CVD risk prediction remains unknown. Purpose To adapt the recalibration method for survival neural network and assess its capability to predict 10-year CVD risk in UK and Chinese populations. Methods We selected adults aged 40-74 years without prior CVD from UK Biobank cohort and CHERRY cohort, an electronic health records-based Chinese cohort. 80% of participants of UKB cohort were used for model derivation, the rest were for internal validation, and the CHERRY cohort was for external validation. We used two different models of survival neural networks: ⑴ deepsurv which models the hazard function using Cox regression; ⑵ deephit which directly learns the distribution of survival time. Our models were based on predictors such as sex, age, smoking status, systolic blood pressure, total cholesterol, high-density lipoprotein cholesterol and history of diabetes. Model performance was evaluated in discrimination, calibration and reclassification compared with a Cox regression model. We recalibrated neural network models using the population-based incidence rate estimated from the CHERRY cohort. Results 347,206 participants from the UKB cohort (median[p25-p75] age:58[50-63]; women:54.7%) and 177,756 participants from the CHERRY cohort (median[p25-p75] age:55[48-62]; women:53.6%) were included for analyses. The 10-year Kaplan-Meier CVD rate was higher in CHERRY than in UKB (8.03%vs.3.66%). Using the same traditional CVD risk factors, compared to Cox regression, neural network models had higher C statistics in both UKB (0.732-0.734 vs. 0.731,P<0.001) and CHERRY cohorts (0.727-0.736 vs. 0.726,P<0.001). All models were well-calibrated in UKB, but underpredicted risk by around 60% (expected-observed ratio: 0.41-0.42) in CHERRY. Population-based recalibration yields substantial improvement (expected-observed ratio: 0.87-0.93). Moreover, recalibrated neural network models showed improved reclassification compared to Cox regression (the net reclassification improvements:1.89%-7.52% in UKB, 4.31%-5.17% in CHERRY), with a more distinct distribution of predicted risk. Conclusion Survival neural networks showed good discrimination in CVD risk prediction and consistently ranked low- and high-risk individuals across diverse populations. Like traditional regression, these models also need to be rescaled to adapt to the risk characteristics of the target population, and recalibration using population-based data could be a feasible method.Discrimination and calibrationRisk distribution and reclassification
更多查看译文
关键词
cardiovascular disease risk,cardiovascular disease,survival,neural networks
AI 理解论文
溯源树
样例
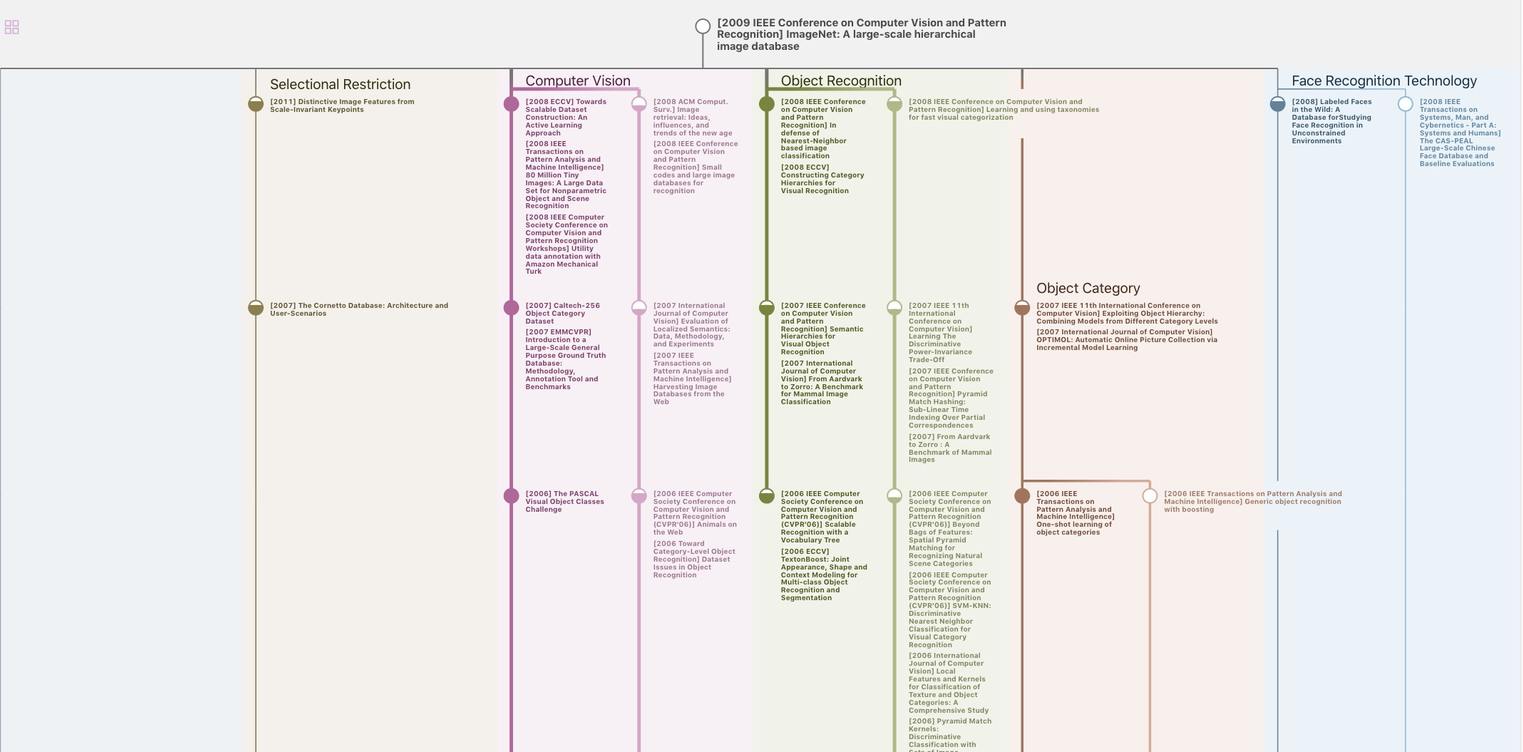
生成溯源树,研究论文发展脉络
Chat Paper
正在生成论文摘要