Light-YOLOv5: A Lightweight Drone Detector for Resource-Constrained Cameras
IEEE INTERNET OF THINGS JOURNAL(2024)
摘要
Critical infrastructures (CIs), such as military bases and airports, are putting a lot of attention into defending against attacks delivered via drones by deploying drone detection systems. However, the CI area might be very large, with no-fly zones extending to regions where it might not be possible to deploy a power line for resourceful cameras. To this aim, the CI might deploy an Internet of Things (IoT)-based surveillance camera system to capture drone images. However, these IoT cameras are resource-constrained devices that cannot support the currently available detectors. In this article, we propose Light-YOLOv5, a lightweight image-based drone detector for resource-constrained cameras. We make targeted improvements to YOLOv5, including the replacement of the backbone network, the introduction of the transformer module, and the design of a parallel mixed efficient attention module (PEAM). We show that our modifications allow for reduced network size while achieving better classification than other state-of-the-art solutions. To prove these claims, we expanded an already available data set of blurred drone images by adding clear images of aircraft and birds. Since airplanes and birds are easily confused as drones by image classifiers, our addition proves the effectiveness of our solution. Experiments show that Light-YOLOv5 can achieve a very good tradeoff between performance (74.8% mAP) and efficiency (170 FPS). Compared to YOLOv5, Light-YOLOv5 improves mAP by 4.1%, reduces the number of network parameters by 15.7%, can perform detection at 170 frames per second (FPS), and achieves an average accuracy rate of 93.8%.
更多查看译文
关键词
Resource-constrained cameras,unmanned aerial vehicle (UAV) detection,YOLOv5
AI 理解论文
溯源树
样例
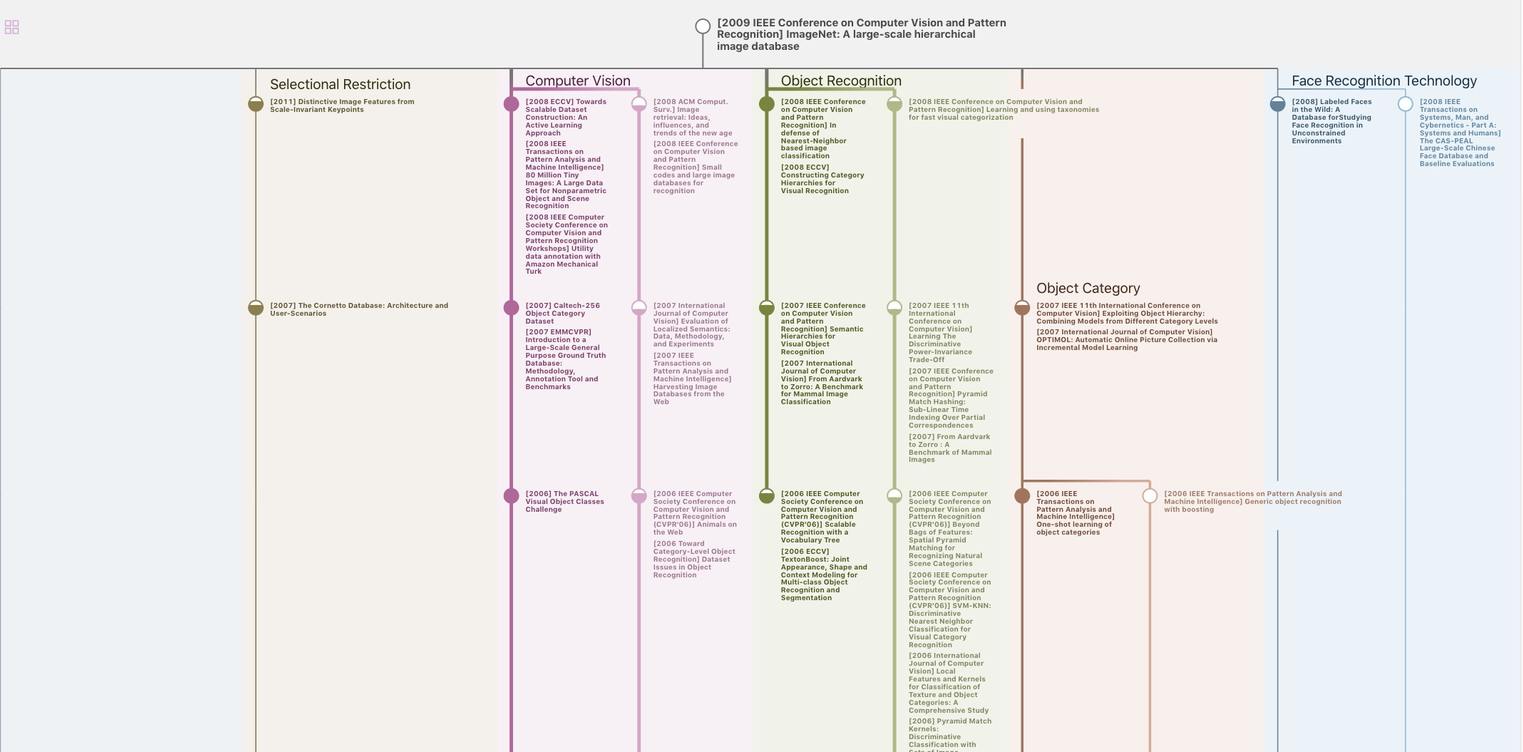
生成溯源树,研究论文发展脉络
Chat Paper
正在生成论文摘要