Towards Hierarchical Intent Disentanglement for Bundle Recommendation
IEEE Transactions on Knowledge and Data Engineering(2023)
摘要
Bundle recommendation aims to recommend a bundle of items for the user to purchase together, for which two scenarios (
i.e.
Next-bundle recommendation and Within-bundle recommendation) are explored to recommend a specific bundle of items for the user and a specific item to fill the user's current bundle, respectively. Previous works largely model the user's preference with a uniform intent, without considering the diversity of intents when adopting the items within the bundle. In the real scenario of bundle recommendation, user intents modeling actually needs to be considered from three hierarchical levels, for that: a user's intents may be naturally distributed in different bundles (user level), one bundle may contain multiple intents of a user (bundle level), and an item in different bundles may also present different user intents (item level). To this end, we develop a novel model,
H
ierarchical
I
ntent
D
isentangle
G
raph
N
etworks (HIDGN) for bundle recommendation. HIDGN is capable of capturing the diversity of the user's intent precisely and comprehensively from the hierarchical structure with an cross-task intent contrastive learning, which is unified with the supervised next-/within-bundle recommendation sub-tasks as a multi-task framework. Extensive experiments on three benchmark datasets demonstrate that HIDGN outperforms the state-of-the-art methods by 43.0%, 13.2%, and 73.3%, respectively.
更多查看译文
关键词
Bundle recommendation,contrastive learning,disentangled representation learning
AI 理解论文
溯源树
样例
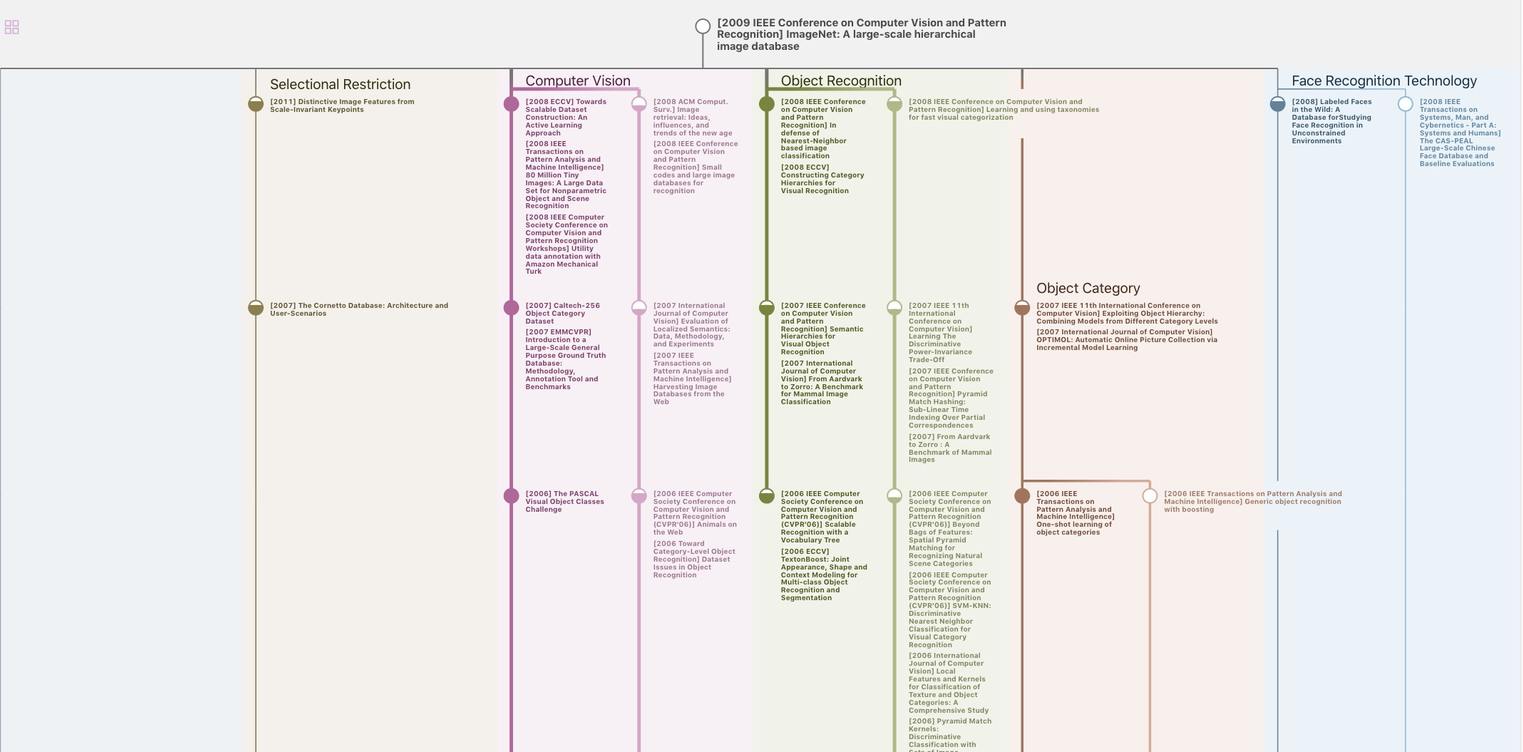
生成溯源树,研究论文发展脉络
Chat Paper
正在生成论文摘要