AI-Assisted E2E Network Slicing for Integrated Sensing and Communication in 6G Networks.
IEEE Internet Things J.(2024)
摘要
In the realm of modern wireless networks, the integration of wireless sensing and communication systems is pivotal, especially in the context of the forthcoming 6G Internet of Things (IoT) paradigm. The popularity of integrated sensing and communication (ISAC) stems from its potential to amplify the utilization of existing network infrastructures. This study introduces an innovative fusion of joint communication and sensing (JCAS), a framework of ISAC, combined with end-to-end (E2E) network slicing (NS) techniques. The aim is to meet user quality of service (QoS) expectations within the ambit of 6G IoT applications. The prime objective is to optimize resource allocation for communication and sensing services within a bespoke network slice tailored for 6G IoT scenarios. This is achieved by minimizing E2E system latency, essential for real-time decision-making in 6G IoT environments. The optimization challenge is tackled by using deep reinforcement learning (DRL) in the form of a deep Q network (DQN) algorithm, which is adept at addressing non-linear integer programming (NLIP) issues intrinsic to 6G IoT settings. Comprehensive simulations validate the approach, demonstrating its effectiveness in the context of 6G IoT networks. The amalgamation of ISAC with E2E network slicing emerges as a potent strategy for furnishing enhanced services customized for 6G IoT applications, successfully fulfilling consumers’ QoS requisites. This integrated approach holds substantial promise as a robust solution for addressing the exacting demands of forthcoming wireless networks, particularly those underpinned by the strides in 6G IoT technologies.
更多查看译文
关键词
AI,Deep Reinforcement Learning,Network Slicing,Integrated Sensing and Communication,Resource Optimization,IoT Network,6G Networks
AI 理解论文
溯源树
样例
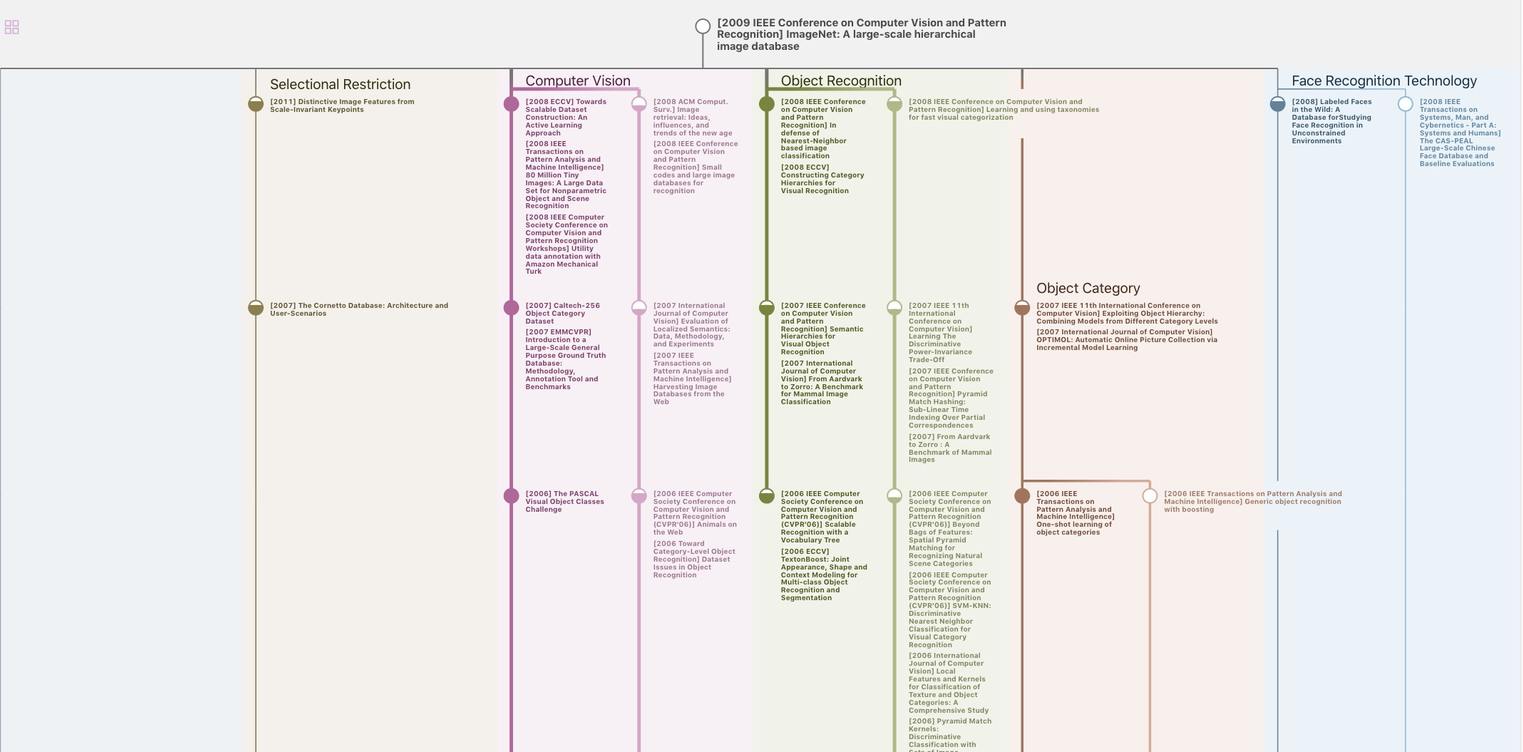
生成溯源树,研究论文发展脉络
Chat Paper
正在生成论文摘要