Economic and Energy-Efficient Wireless Federated Learning Based on Stackelberg Game.
IEEE Trans. Veh. Technol.(2024)
摘要
Wireless federated learning (FL) is a distributed learning framework, in which mobile devices (MDs) collaboratively train a model while protecting data privacy. Considering selfishness of MDs, they are unwilling to sacrifice their resources to assist model training without any reward, which may limit performance of FL. In this paper, we propose an incentive mechanism between a base station (BS) and several mobile devices (MDs) modeled as a Stackelberg game. During this game, BS first decides the total reward that can be distributed to MDs, and then each MD determines its number of local iterations according to its utility function. In addition, we derive the closed-form expressions of the optimal reward function of the BS and the number of local iterations of the MDs. Finally, the numerical results validate the efficacy of our proposed scheme.
更多查看译文
关键词
Federated learning,resource optimization,Stackelberg game,incentive mechanism
AI 理解论文
溯源树
样例
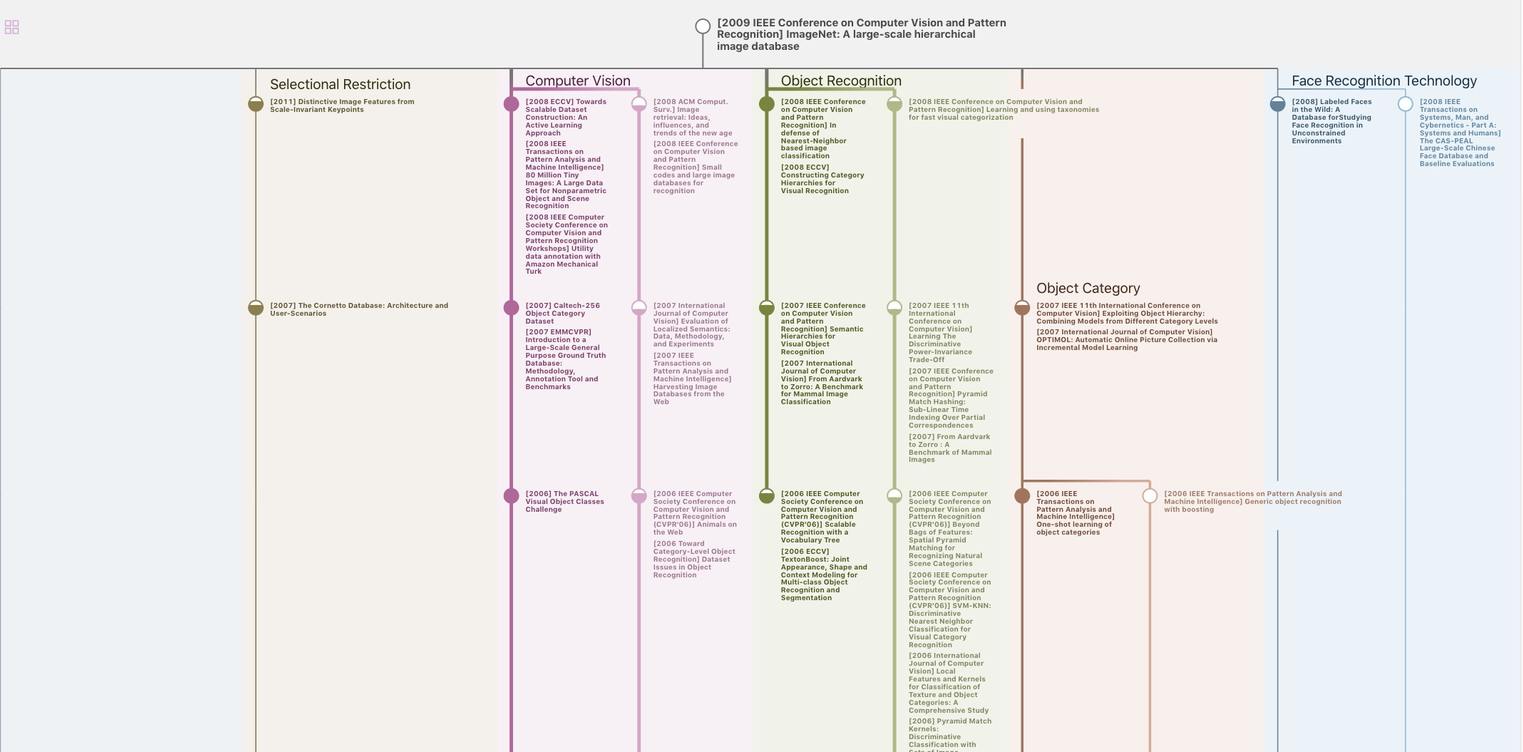
生成溯源树,研究论文发展脉络
Chat Paper
正在生成论文摘要