Decentralized Privacy-Preserving Electricity Theft Detection for Distribution System Operators
IEEE TRANSACTIONS ON SMART GRID(2024)
摘要
Distribution system operators (DSO) may benefit from sharing key information to detect electricity theft with data-driven methods. However, the privacy of electricity consumers must be preserved during training the detection model. To address this problem, we propose a decentralized federated learning framework to train the cross-DSO detection model under the premise of protecting the privacy of consumers. Firstly, a privacy-preserving protocol based on threshold homomorphic encryption is developed to provide parameters aggregation between DSO while a federated server is unnecessary. The dropout of DSO is allowed during model training. Then, based on the proposed framework, we design a decentralized federated extreme gradient boosting model to detect electricity theft. Encrypted gradient histograms are used to aggregate parameters and find the best split in the federated framework. Finally, the performance of the proposed model is verified on the dataset of Low Carbon London project. The results present that the proposed decentralized federated model has similar performance to the centralized model whether on imbalanced or non-independent identically distribution datasets.
更多查看译文
关键词
Data models,Federated learning,Data privacy,Privacy,Smart meters,Load modeling,Training,Electricity theft detection,decentralized federated learning,privacy protection,distribution system operators
AI 理解论文
溯源树
样例
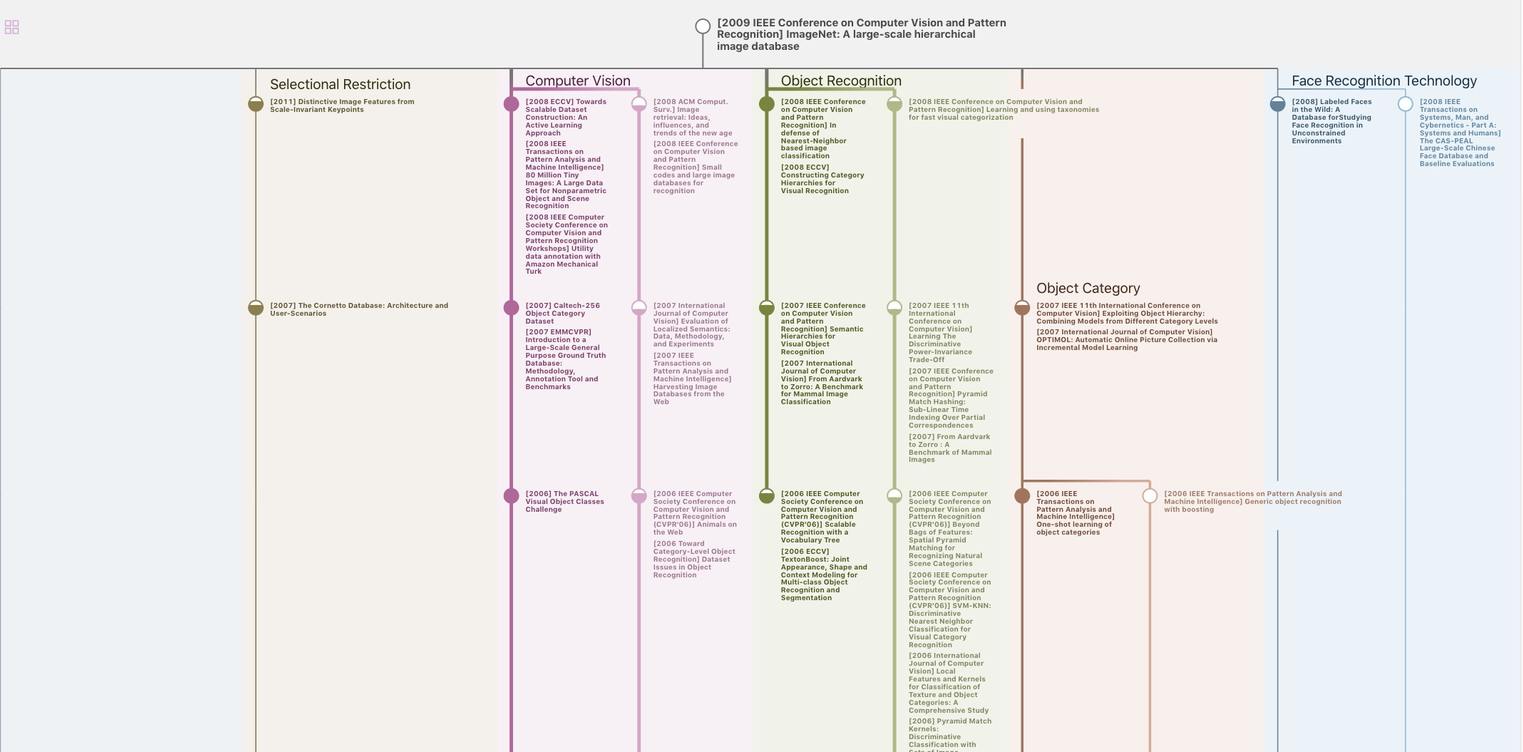
生成溯源树,研究论文发展脉络
Chat Paper
正在生成论文摘要