GPT-COPE: A Graph-Guided Point Transformer for Category-Level Object Pose Estimation
IEEE Transactions on Circuits and Systems for Video Technology(2023)
摘要
Category-level object pose estimation aims to predict the 6D pose and 3D metric size of objects from given categories. Due to significant intra-class shape variations among different instances, existing methods have mainly focused on estimating dense correspondences between observed point clouds and their canonical representations, i.e., normalized object coordinate space (NOCS). Subsequently, a similarity transformation is applied to recover the object pose and size. Despite these efforts, current approaches still cannot fully exploit the intrinsic geometric features to individual instances, thus limiting their ability to handle objects with complex structures (i.e., cameras). To overcome this issue, this paper introduces GPT-COPE, which leverages a graph-guided point transformer to explore distinctive geometric features from the observed point cloud. Specifically, our GPT-COPE employs a Graph-Guided Attention Encoder to extract multiscale geometric features in a local-to-global manner and utilizes an Iterative Non-Parametric Decoder to aggregate the multiscale geometric features from finer scales to coarser scales without learnable parameters. After obtaining the aggregated geometric features, the object NOCS coordinates and shape are regressed through the shape prior adaptation mechanism, and the object pose and size are obtained using the Umeyama algorithm. The multiscale network design enables perceiving the overall shape and structural information of the object, which is beneficial to handle objects with complex structures. Experimental results on the NOCS-REAL and NOCS-CAMERA datasets demonstrate that our GPT-COPE achieves state-of-the-art performance and significantly outperforms existing methods. Furthermore, our GPT-COPE shows superior generalization ability compared to existing methods on the large-scale in-the-wild dataset Wild6D and achieves better performance on the REDWOOD75 dataset, which involves objects with unconstrained orientations.
更多查看译文
关键词
object pose estimation,shape reconstruction,3D graph convolution,vision transformer
AI 理解论文
溯源树
样例
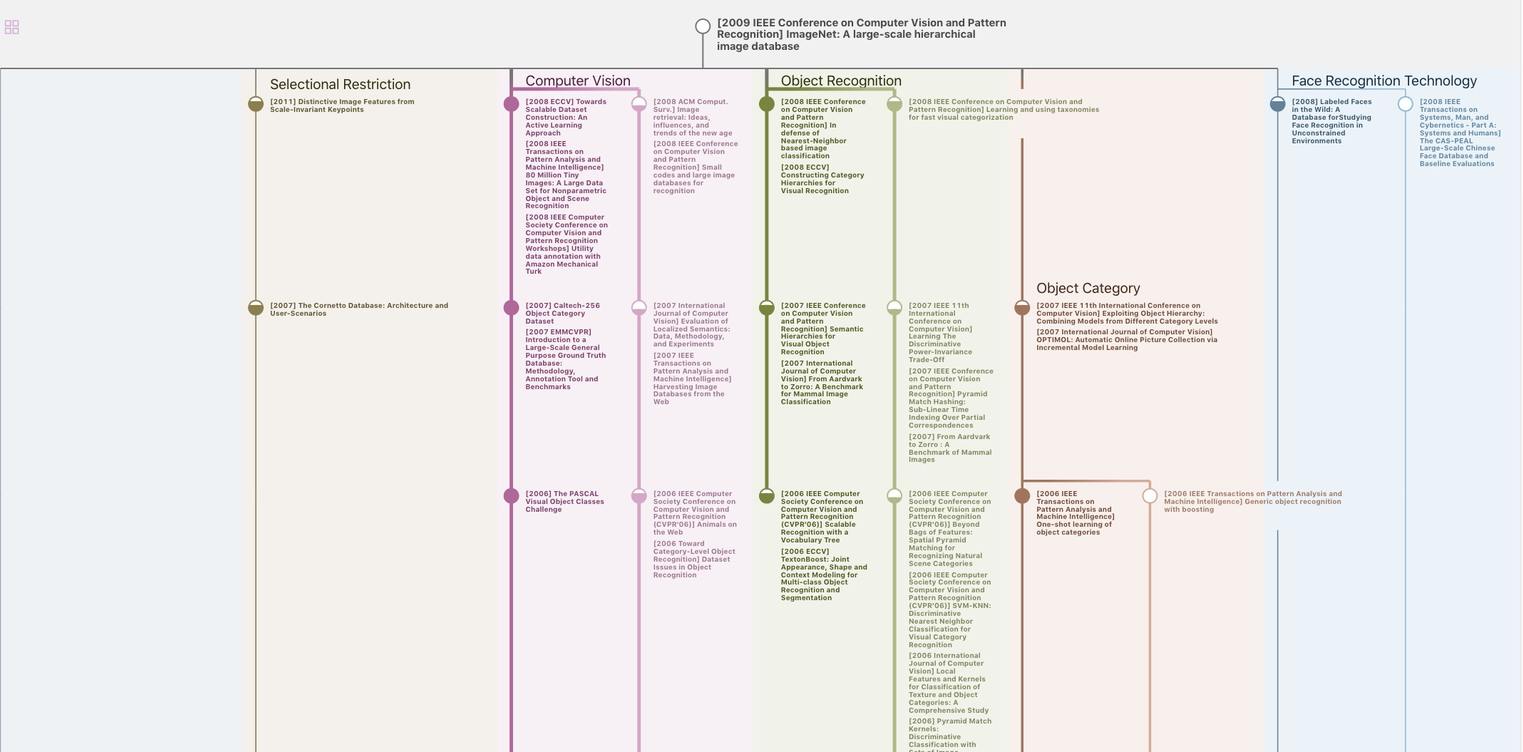
生成溯源树,研究论文发展脉络
Chat Paper
正在生成论文摘要