Multiobjective Deep Reinforcement Learning Assisted Resource Allocation for MEC-Caching-Coexist System.
IEEE Internet of Things Journal(2024)
摘要
In order to overcome the vicious competition between different high-volume services, we study the wireless resource sharing problem in the transmission process of the MEC-caching-coexist (MCCe) system with the capability of mmWave communications. The multi-objective Markov decision process (MOMDP) is introduced to model the task scheduling and resource allocation problem for the mmWave links, which aims to minimize the transmission delay and energy consumption simultaneously. Note that, for practical consideration, the exact channel information of all links are not known. We propose a novel multi-objective deep reinforcement learning with discrete-continuous hybrid action space (MODRL/HA) algorithm. In particular, the envelope updated design (EUD) is designed to realize the multi-objective optimization from the perspective of the Bellman operator. On the other hand, the parameterized network design (PND) is developed to deal with the hybrid action space of discrete task scheduling and continuous beamwidth and power variables. Our simulations show that, MODRL/HA algorithm can improve 22% performance in terms of the trade-off between delay and energy consumption compared with the benchmark schemes, which are original Deep Deterministic Policy Gradient (DDPG) and multi-objective DDPG (MODDPG) algorithms.
更多查看译文
关键词
MEC-caching-coexist system,wireless resource sharing,multi-objective optimization
AI 理解论文
溯源树
样例
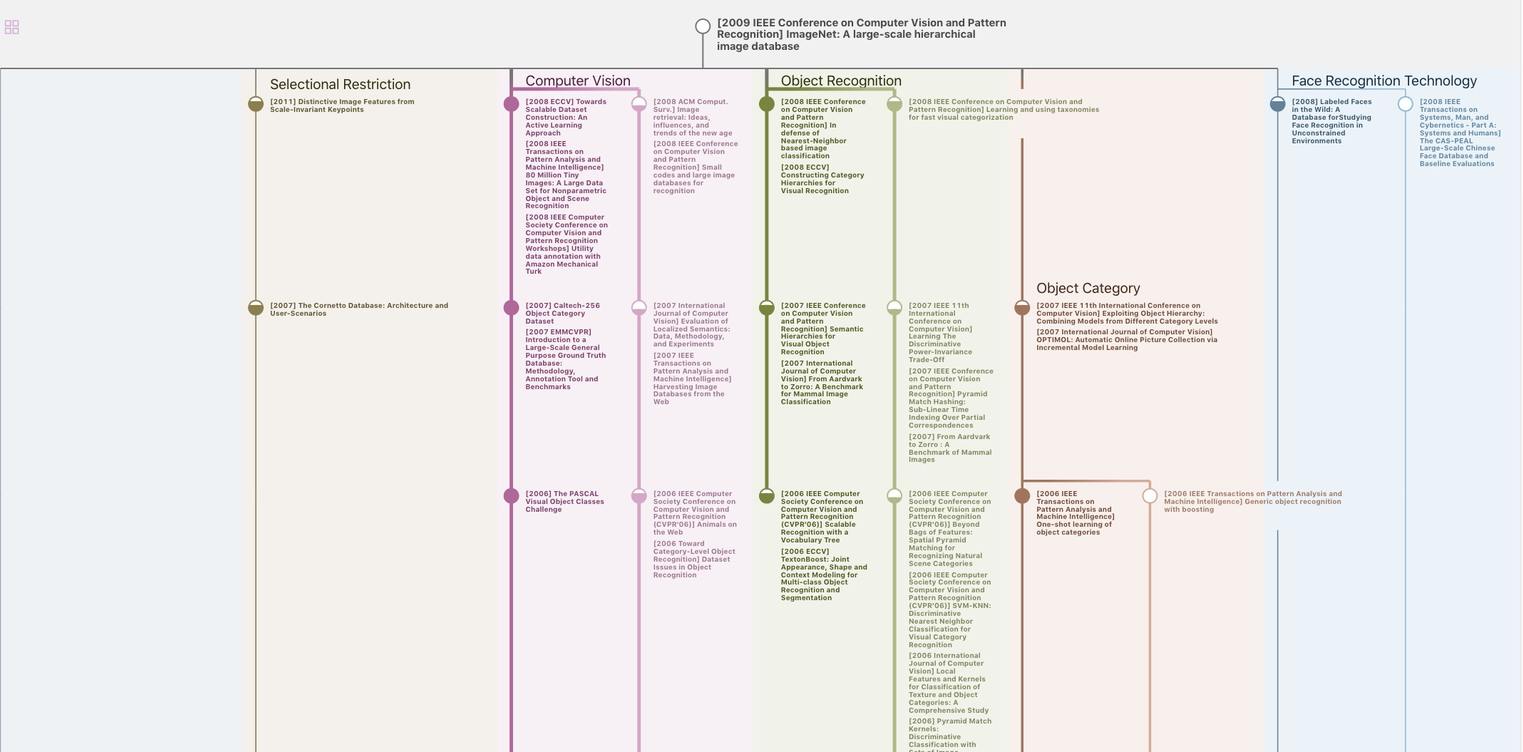
生成溯源树,研究论文发展脉络
Chat Paper
正在生成论文摘要