Classification and Concentration Predictions of Volatile Organic Compounds Using an Electronic Nose Based on XGBoost-Random Forest Algorithms
IEEE SENSORS JOURNAL(2024)
摘要
The electronic nose (E-nose) is widely used for quantitative monitoring of poisonous and harmful gases. However, its conventional gas sensing is generally carried out based on a single estimator, which lacks a large-scale training set to train the models, leading to a low classification and regression accuracy. In this regard, this work explores an E-nose based on a gas sensor array, which predicts both types and concentrations of gases accurately and efficiently. Classical ensemble machine learning algorithms, XGBoost, and random forest (RF) are selected as candidates for designing the classifiers and regressors of the E-nose. The classifier recognizes six different volatile organic compounds (VOCs), while the regressor predicts the concentration of each gas component. The fivefold cross-validation method is used to search for the best training parameters of each model. Different from the previous works that solely relied on a single model, such as back-propagation neural network and decision tree, this work constructs a model system based on XGBoost-RF algorithms, which demonstrates superior performance. The model system achieves an accuracy of 96.0% using the XGBoost classifier and an average R-2 score of 0.923 using the RF regressor in predicting six kinds of VOCs. Moreover, the novel model system has a high testing efficiency, with predicting time of 0.011 s in the classification process and 0.015 s in the regression process. This study provides a novel approach to design an accurate and efficient E-nose system for the quantitative detection of VOCs.
更多查看译文
关键词
Concentration prediction,electronic nose (E-nose),machine learning,sensor array,type recognition
AI 理解论文
溯源树
样例
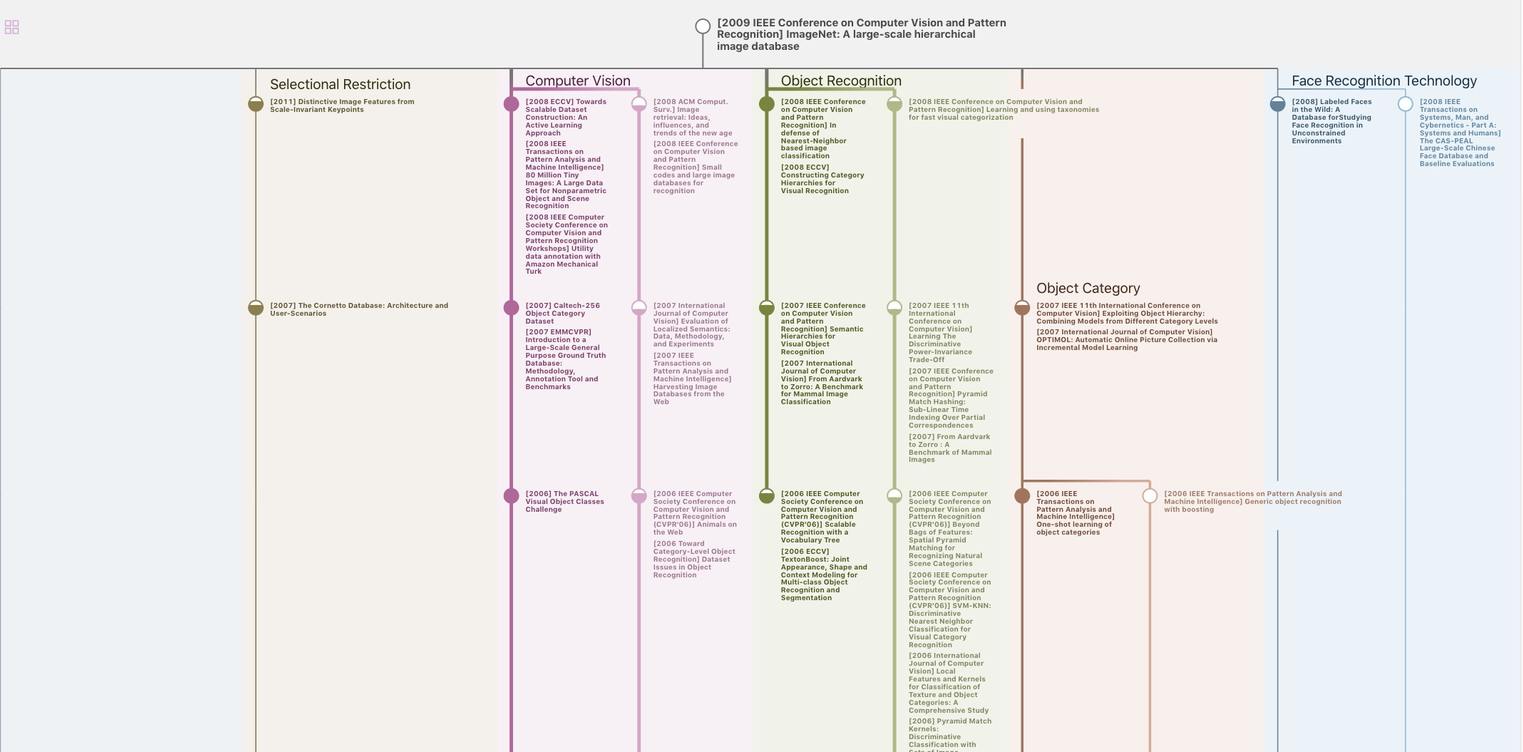
生成溯源树,研究论文发展脉络
Chat Paper
正在生成论文摘要