Simultaneous Denoising and Reconstruction of DAS Seismic Data via a Multi-Cascade Deep Learning Method
Geophysics(2023)
摘要
Distributed acoustic sensing (DAS) has been moderately used in seismic exploration as a consequence of its advantages, such as low cost, high resolution, high sensitivity, and strong resistance to high temperatures. Nonetheless, seismic data recorded by the DAS system often are seriously contaminated by a massive amount of background noise and recording gaps, which also decrease the quality of DAS seismic data. Building on its success in numerous fields of data processing, convolutional neural networks (CNNs) also have been applied to seismic data denoising as well as reconstruction and achieved good performance compared with traditional methods. However, relatively few CNN-based studies have been done on the simultaneous denoising and reconstruction of seismic data, especially for the complex DAS seismic data with a low signal-to-noise ratio. In this study, we have developed a multicascade network structure in combination with a channel attention mechanism (MCA-Net) to achieve the simultaneous denoising and reconstruction of DAS seismic data by only using a unified trained model. Particularly, our MCA-Net consists of three branches of cascade networks that can extract abundant features from DAS seismic data with different resolutions. Furthermore, we deploy some channel attention modules and aggressive blocks to MCA-Net to fuse these aforementioned multiresolution features and thus improving the feature extraction ability of the whole network. Meanwhile, a high-quality training data set composed of paired clean complete and noisy incomplete patches is used to train MCA-Net in a supervised fashion. Some synthetic and real DAS seismic data are used to demonstrate the effectiveness of MCA-Net, and our method exhibits better denoising and reconstruction performance than some traditional methods and two existing CNN architectures.
更多查看译文
关键词
das seismic data,deep learning,multi-cascade
AI 理解论文
溯源树
样例
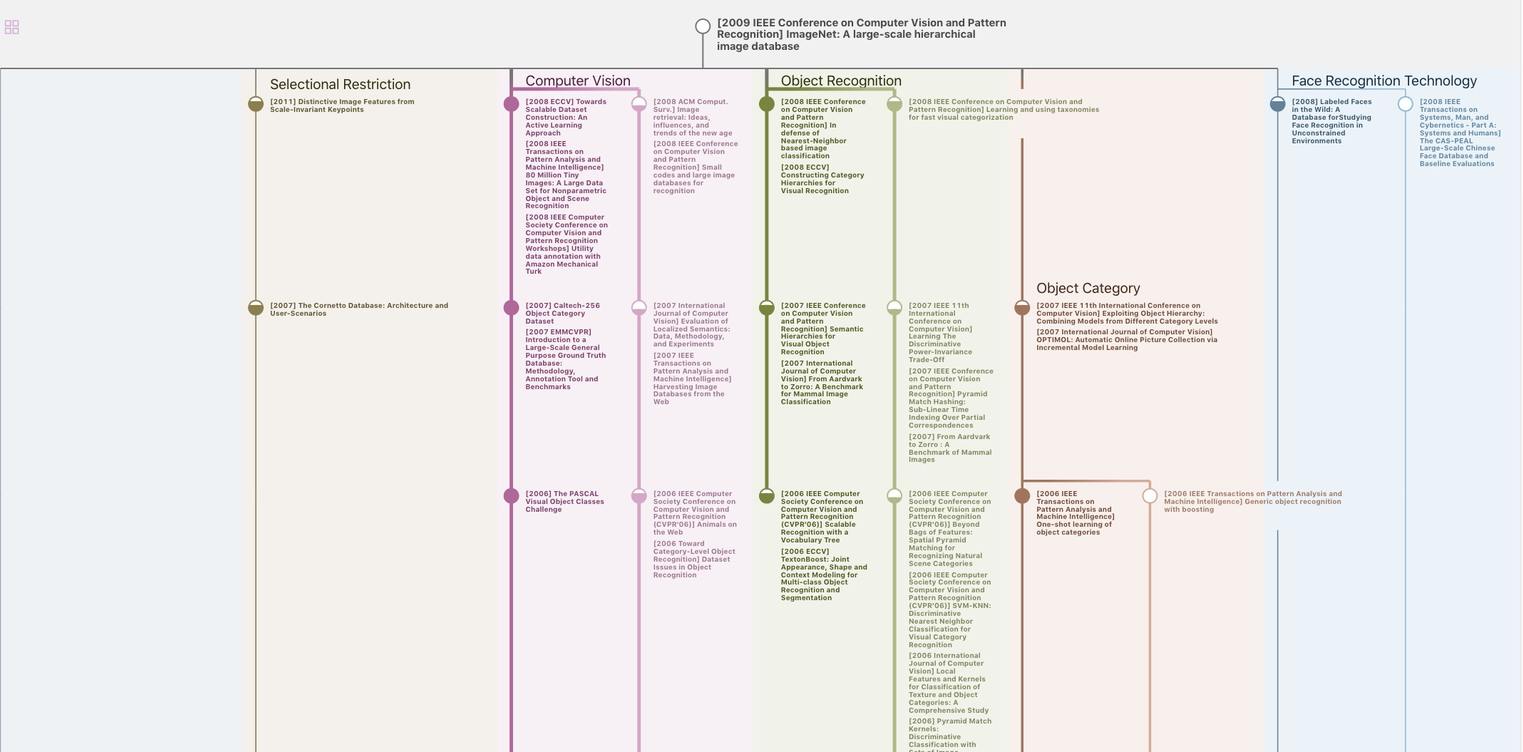
生成溯源树,研究论文发展脉络
Chat Paper
正在生成论文摘要