DDT: A Reinforcement Learning Approach to Dynamic Flow Timeout Assignment in Software Defined Networks
Journal of Network and Systems Management(2024)
摘要
OpenFlow-compliant commodity switches face challenges in efficiently managing flow rules due to the limited capacity of expensive high-speed memories used to store them. The accumulation of inactive flows can disrupt ongoing communication, necessitating an optimized approach to flow rule timeouts. This paper proposes Delayed Dynamic Timeout (DDT), a Reinforcement Learning-based approach to dynamically adjust flow rule timeouts and enhance the utilization of a switch’s flow table(s) for improved efficiency. Despite the dynamic nature of network traffic, our DDT algorithm leverages advancements in Reinforcement Learning algorithms to adapt and achieve flow-specific optimization objectives. The evaluation results demonstrate that DDT outperforms static timeout values in terms of both flow rule match rate and flow rule activity. By continuously adapting to changing network conditions, DDT showcases the potential of Reinforcement Learning algorithms to effectively optimize flow rule management. This research contributes to the advancement of flow rule optimization techniques and highlights the feasibility of applying Reinforcement Learning in the context of SDN.
更多查看译文
关键词
Software defined networking,SDN,OpenFlow,Timeout,Machine learning,Reinforcement learning
AI 理解论文
溯源树
样例
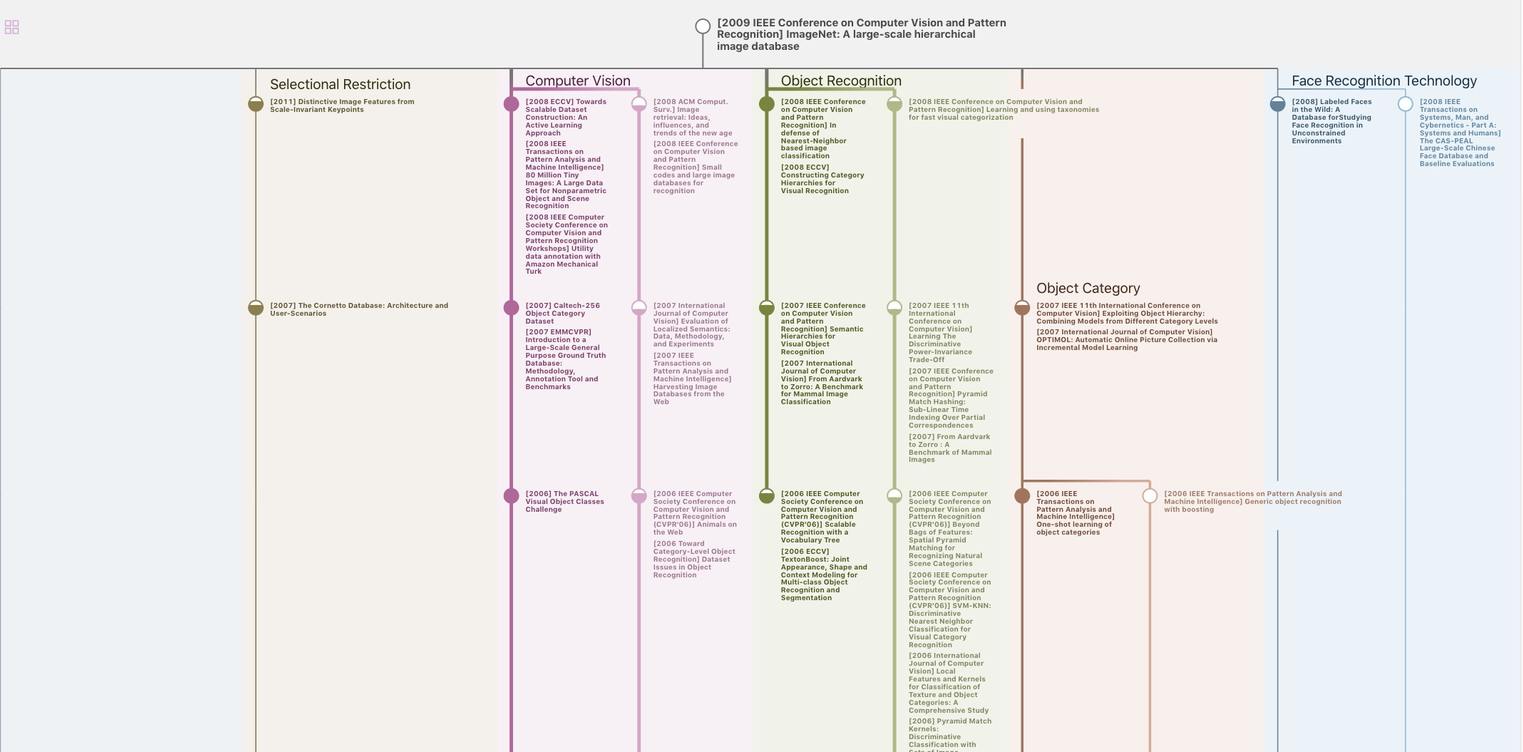
生成溯源树,研究论文发展脉络
Chat Paper
正在生成论文摘要