GaitFormer: Revisiting Intrinsic Periodicity for Gait Recognition
arXiv (Cornell University)(2023)
摘要
Gait recognition aims to distinguish different walking patterns by analyzing video-level human silhouettes, rather than relying on appearance information. Previous research on gait recognition has primarily focused on extracting local or global spatial-temporal representations, while overlooking the intrinsic periodic features of gait sequences, which, when fully utilized, can significantly enhance performance. In this work, we propose a plug-and-play strategy, called Temporal Periodic Alignment (TPA), which leverages the periodic nature and fine-grained temporal dependencies of gait patterns. The TPA strategy comprises two key components. The first component is Adaptive Fourier-transform Position Encoding (AFPE), which adaptively converts features and discrete-time signals into embeddings that are sensitive to periodic walking patterns. The second component is the Temporal Aggregation Module (TAM), which separates embeddings into trend and seasonal components, and extracts meaningful temporal correlations to identify primary components, while filtering out random noise. We present a simple and effective baseline method for gait recognition, based on the TPA strategy. Extensive experiments conducted on three popular public datasets (CASIA-B, OU-MVLP, and GREW) demonstrate that our proposed method achieves state-of-the-art performance on multiple benchmark tests.
更多查看译文
关键词
gaitformer recognition,intrinsic periodicity
AI 理解论文
溯源树
样例
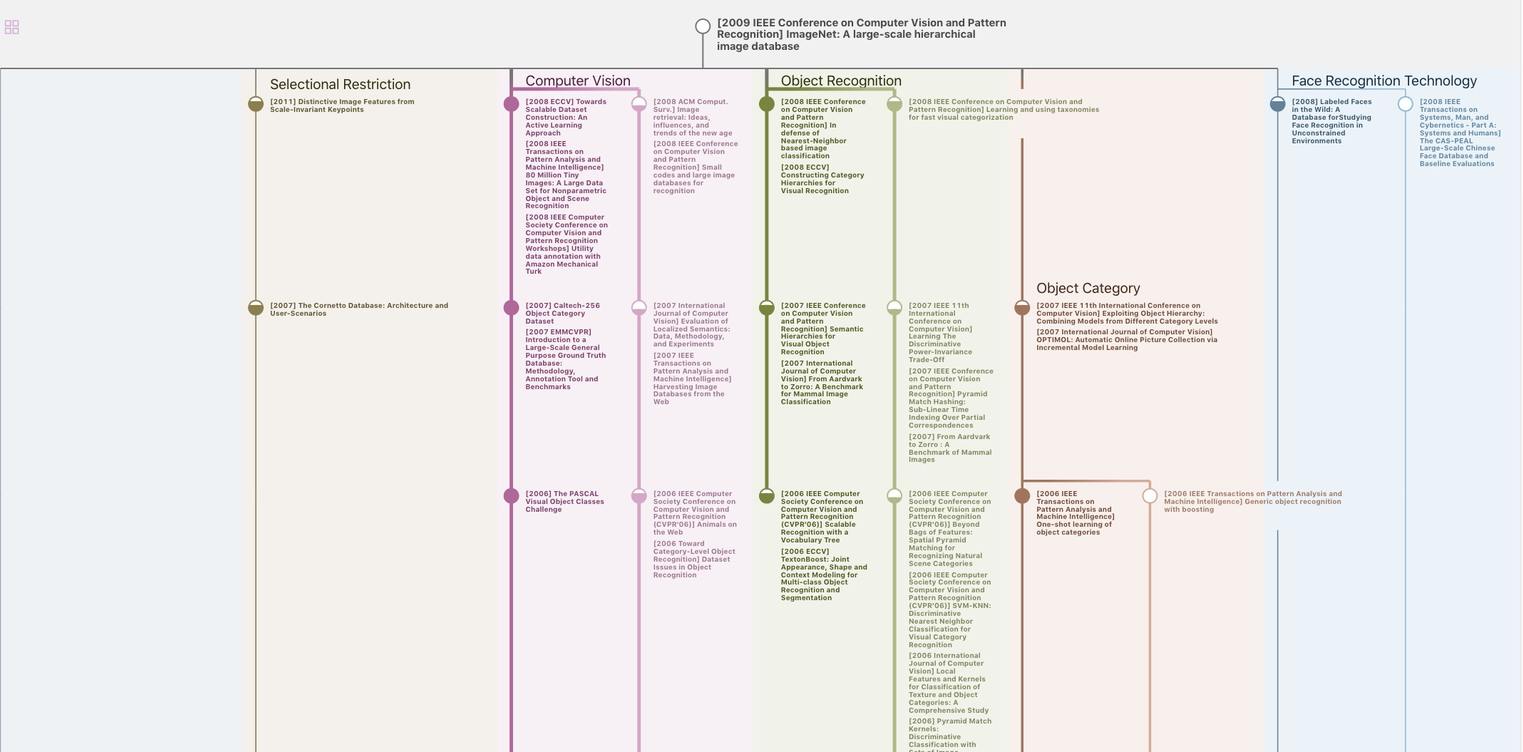
生成溯源树,研究论文发展脉络
Chat Paper
正在生成论文摘要