Non-Autoregressive Document-Level Machine Translation
arXiv (Cornell University)(2023)
摘要
Non-autoregressive translation (NAT) models achieve comparable performance and superior speed compared to auto-regressive translation (AT) models in the context of sentence-level machine translation (MT). However, their abilities are unexplored in document-level MT, hindering their usage in real scenarios. In this paper, we conduct a comprehensive examination of typical NAT models in the context of document-level MT and further propose a simple but effective design of sentence alignment between source and target. Experiments show that NAT models achieve high acceleration on documents, and sentence alignment significantly enhances their performance. However, current NAT models still have a significant performance gap compared to their AT counterparts. Further investigation reveals that NAT models suffer more from the multi-modality and misalignment issues in the context of document-level MT, and current NAT models struggle with exploiting document context and handling discourse phenomena. We delve into these challenges and provide our code at \url{https://github.com/baoguangsheng/nat-on-doc}.
更多查看译文
关键词
translation,non-autoregressive,document-level
AI 理解论文
溯源树
样例
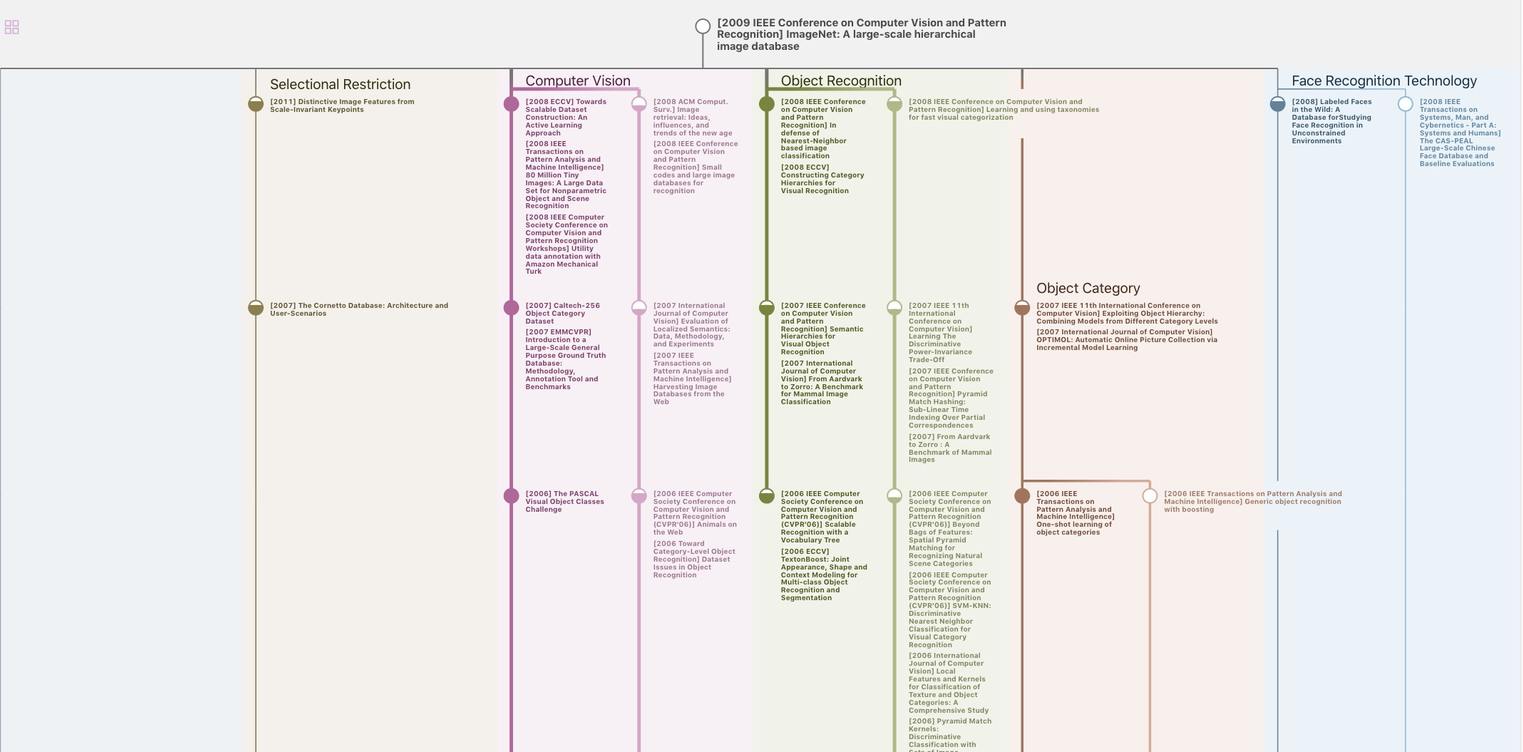
生成溯源树,研究论文发展脉络
Chat Paper
正在生成论文摘要