Supervised Feature Selection to Improve the Accuracy for Malware Detection
Research Square (Research Square)(2023)
摘要
Abstract Malware is becoming increasingly sophisticated and difficult to detect with traditional monitoring tools and antivirus software. As a result, machine learning has become a popular approach for classifying and detecting malware-related data. In this study, two distinct datasets, Malware-Exploratory and CIC-MalMem-2022, were subjected to a series of supervised and unsupervised learning procedures to gather information for observation. The developed model in this research uses three clustering algorithms for analysis, namely K-Means, Density-Based Spatial Clustering of Applications with Noise, and Gaussian Mixture Model, and seven classification algorithms for predicting malware, namely Decision Tree, Random Forest, Ada Boost, KNeighbors, Stochastic Gradient Descent, Extra Trees, and Gaussian Naïve Bayes. Results show that the Malware-Exploratory dataset achieved an accuracy score of 90%, while the CIC-MalMem-2022 dataset achieved a score of 99%. Additionally, both datasets demonstrated consistency across all three clustering algorithms, indicating that variables need not be highly correlated for successful malware detection. Future studies will determine the stability of the results against feature selection and genetic algorithms.
更多查看译文
关键词
feature selection,detection
AI 理解论文
溯源树
样例
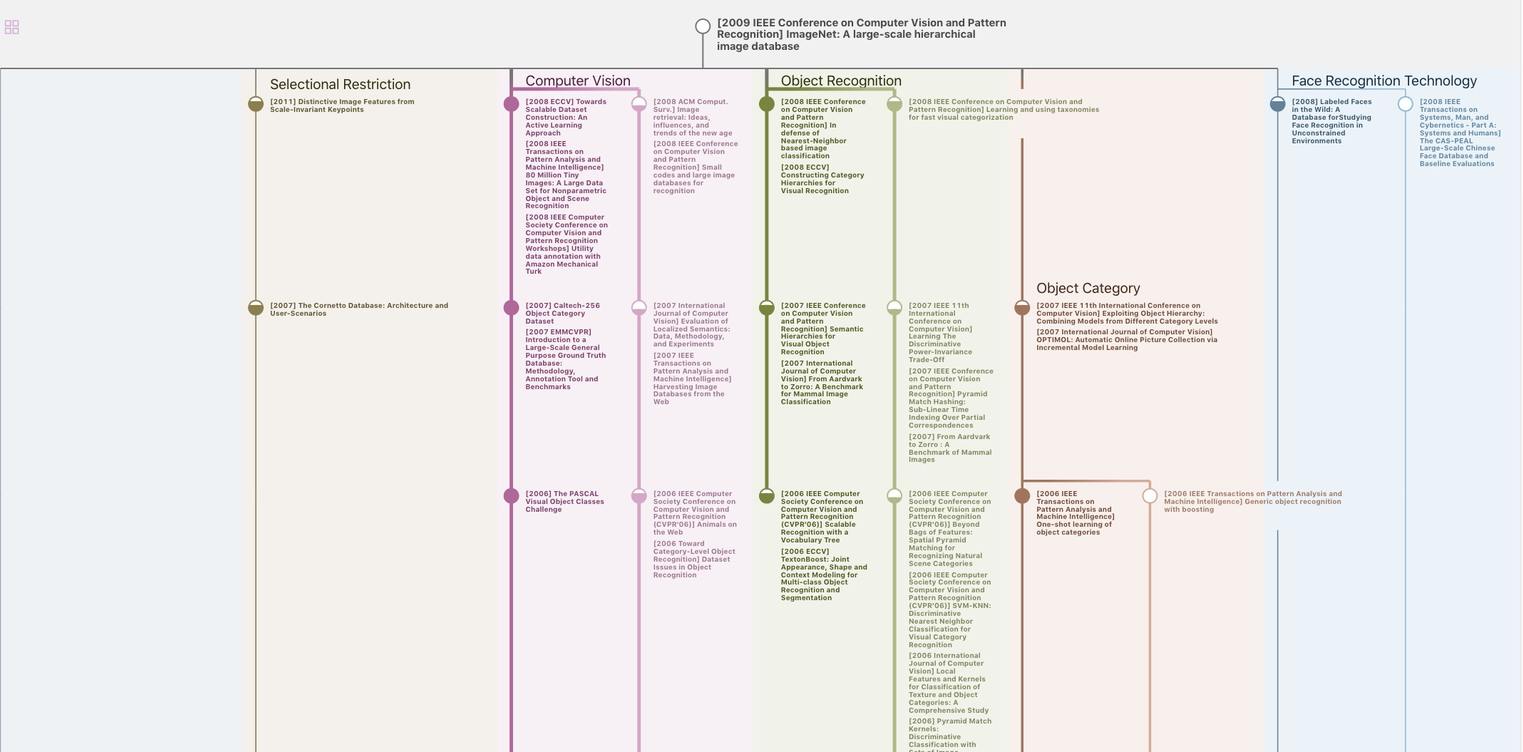
生成溯源树,研究论文发展脉络
Chat Paper
正在生成论文摘要