Adaptive-Mask Fusion Network for Segmentation of Drivable Road and Negative Obstacle With Untrustworthy Features
arXiv (Cornell University)(2023)
摘要
Segmentation of drivable roads and negative obstacles is critical to the safe driving of autonomous vehicles. Currently, many multi-modal fusion methods have been proposed to improve segmentation accuracy, such as fusing RGB and depth images. However, we find that when fusing two modals of data with untrustworthy features, the performance of multi-modal networks could be degraded, even lower than those using a single modality. In this paper, the untrustworthy features refer to those extracted from regions (e.g., far objects that are beyond the depth measurement range) with invalid depth data (i.e., 0 pixel value) in depth images. The untrustworthy features can confuse the segmentation results, and hence lead to inferior results. To provide a solution to this issue, we propose the Adaptive-Mask Fusion Network (AMFNet) by introducing adaptive-weight masks in the fusion module to fuse features from RGB and depth images with inconsistency. In addition, we release a large-scale RGB-depth dataset with manually-labeled ground truth based on the NPO dataset for drivable roads and negative obstacles segmentation. Extensive experimental results demonstrate that our network achieves state-of-the-art performance compared with other networks. Our code and dataset are available at: https://github.com/lab-sun/AMFNet.
更多查看译文
关键词
negative obstacle,drivable road,segmentation,adaptive-mask
AI 理解论文
溯源树
样例
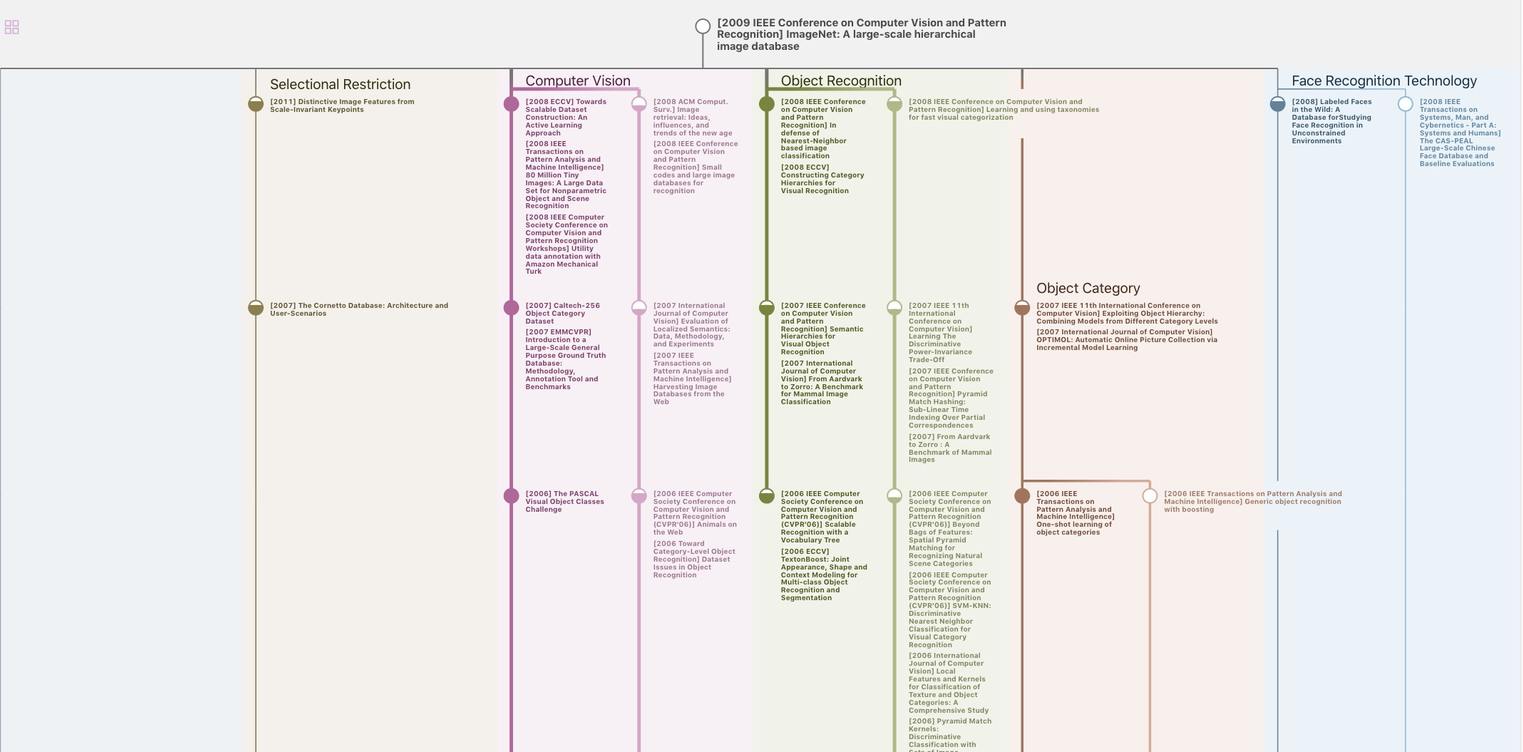
生成溯源树,研究论文发展脉络
Chat Paper
正在生成论文摘要