One Size Does Not Fit All
Chest(2023)
摘要
FOR RELATED ARTICLE, SEE PAGE 790COPD, a highly prevalent chronic pulmonary disease, is one of the most common reasons for hospital admissions and is associated with increased health care use, morbidity and death, and decreased quality of life.1Benady S. Canadian Thoracic SocietyThe human and economic burden of COPD: a leading cause of hospital admission in Canada. Canadian Thoracic Society, 2010Google Scholar Thus, identifying individuals at higher risk for exacerbations through a process called “risk stratification” is critical to guiding the management of COPD. FOR RELATED ARTICLE, SEE PAGE 790 A history of COPD exacerbations has been reported as the best single predictor of future exacerbations2Hurst J.R. Vestbo J. Anzueto A. et al.Susceptibility to exacerbation in chronic obstructive pulmonary disease.N Engl J Med. 2010; 363: 1128-1138Crossref PubMed Scopus (2123) Google Scholar,3Marott J.L. Colak Y. Ingebrigtsen T.S. Vestbo J. Nordestgaard B.G. Lange P. Exacerbation history, severity of dyspnoea and maintenance treatment predicts risk of future exacerbations in patients with COPD in the general population.Respir Med. 2022; 192106725Abstract Full Text Full Text PDF PubMed Scopus (7) Google Scholar and is emphasized by Global Initiative for Chronic Obstructive Lung Disease4Global Initiative for Chronic Obstructive Lung Disease. 2023 GOLD Report.https://goldcopd.org/2023-gold-report-2/Date accessed: February 4, 2023Google Scholar and by some COPD guidelines5Wedzicha J.A. Calverley P.M.A. Albert R.K. et al.Prevention of COPD exacerbations: a European Respiratory Society/American Thoracic Society guideline.Eur Respir J. 2017; 50: 1602265Crossref PubMed Scopus (118) Google Scholar to inform therapeutic choice. However, relying on COPD exacerbation history alone for risk stratification may be suboptimal, given growing evidence that suggests variability in yearly COPD exacerbation rates within individual patients caused by chance alone6Sadatsafavi M. McCormack J. Petkau J. Lynd L.D. Lee T.Y. Sin D.D. Should the number of acute exacerbations in the previous year be used to guide treatments in COPD?.Eur Respir J. 2021; 572002122Crossref Scopus (13) Google Scholar and high variability in exacerbation rates across the world, even among individuals with the same COPD exacerbation history at baseline.7Calverley P.M.A. Martinez F.J. Vestbo J. et al.International differences in the frequency of chronic obstructive pulmonary disease exacerbations reported in three clinical trials.Am J Respir Crit Care Med. 2022; 206: 25-33Crossref PubMed Scopus (7) Google Scholar Well-calibrated clinical prediction multivariable models that combine COPD exacerbation history and other individual characteristics can increase the accuracy of risk stratification over exacerbation history alone. However, predictive model performance may suffer from miscalibration if applied to clinical populations dissimilar to those used in their development. Prediction models can be validated externally in new populations of differing baseline risk in different settings; however, this is not commonly done, often because of the time and expense required.8Bellou V. Belbasis L. Konstantinidis A.K. Tzoulaki I. Evangelou E. Prognostic models for outcome prediction in patients with chronic obstructive pulmonary disease: systematic review and critical appraisal.BMJ. 2019; 367: l5358Crossref PubMed Scopus (71) Google Scholar This can result in low clinical usefulness of the prediction model.9Gulati G. Upshaw J. Wessler B.S. et al.Generalizability of cardiovascular disease clinical prediction models: 158 independent external validations of 104 unique models.Circ Cardiovasc Qual Outcomes. 2022; 15e008487Crossref Scopus (15) Google Scholar When rigorous further validation does not occur, can a prediction model be universally clinically applicable across different settings; if not, can they be revised (ie, recalibrated) with the use of background exacerbation risk to improve its clinical usefulness? The study by Ho et al10Ho J.K. Safari A. Adibi A. et al.Generalizability of risk stratification algorithms for exacerbations in COPD.Chest. 2023; 163: 790-798Abstract Full Text Full Text PDF PubMed Scopus (2) Google Scholar in this issue of CHEST tried to address these questions by comparing before and after recalibration “discrimination ability” (calculating the area under the curve [AUC]) and “clinical utility” (by net benefit calculations with the use of the decision curve analysis) of three risk stratification algorithms for the prediction of moderate (requiring treatment with systemic corticosteroids and/or antibiotics) and severe (resulting in ED visits or hospitalizations) exacerbations in the subsequent 12 months. Decision curve analysis calculates a clinical “net benefit” for prediction models compared with default strategies of treating all or no patients. A prediction model is considered of “clinical utility” if the net benefit of a model is greater than treating all and no patients. Alternatively, a prediction model is considered “harmful” at a given threshold if its net benefit is lower than treating all or no patients (strategies that do not require risk stratification). The three algorithms consisted of two multivariable prediction models and a history of COPD exacerbation. The multivariable prediction models were (1) the Acute COPD Exacerbation Prediction Tool (ACCEPT), that uses up to 13 predictors, which included a 12-month history of exacerbations, age, sex, smoking status, postbronchodilator FEV1 percent predicted, use of statins, oxygen therapy, BMI, the St. George’s Respiratory Questionnaire 19 score (or COPD Assessment Test 20), current use of inhaled long-acting muscarinic receptor antagonists, long-acting β2 agonists, and inhaled corticosteroids11Adibi A. Sin D.D. Safari A. et al.The acute COPD exacerbation prediction tool (ACCEPT): a modelling study.Lancet Respir Med. 2020; 8: 1013-1021Abstract Full Text Full Text PDF PubMed Scopus (40) Google Scholar and (2) a model by Bertens et al12Bertens L.C. Reitsma J.B. Moons K.G. et al.Development and validation of a model to predict the risk of exacerbations in chronic obstructive pulmonary disease.Int J Chron Obstruct Pulmon Dis. 2013; 8: 493-499Crossref PubMed Scopus (41) Google Scholar that uses a 12-month history of exacerbations, FEV1 expressed as percent predicted, smoking status, and a history of vascular disease. Well-characterized and studied databases from three randomized clinical trials that included clinical populations at three different levels of exacerbation risk were used: the Study to Understand Mortality and Morbidity in COPD (N= 2,421; annual risk of exacerbations = 0.22 [low risk]), the Long-term Oxygen Treatment Trial (N = 595; annual risk = 0.38 [medium risk]), and Towards a Revolution in COPD Health (N = 1,091; annual risk = 0.52 [high risk]). As expected, the prediction models generally had better discriminatory performance than COPD exacerbation history alone. Considering an AUC ≤0.75 as not clinically useful with an AUC value of 0.5 indicating no discriminative value,13Fan J. Upadhye S. Worster A. Understanding receiver operating characteristic (ROC) curves.CJEM. 2006; 8: 19-20Crossref PubMed Scopus (496) Google Scholar only the ACCEPT model of the 3 algorithms demonstrated clinically relevant discriminative ability among high-risk patients, but not in other settings. Exacerbation history alone had an AUC of 0.68, which indicates poor discriminative ability. The authors demonstrated that none of the algorithms had superior clinical utility over the others across all settings with different baseline risks. Before recalibration, the Bertens model performed the best in low exacerbation risk settings, while the ACCEPT model performed the best in high-risk settings. However, all three algorithms could be associated with the risk of harm in some scenarios (ie, providing lower net benefit than not using any risk stratification) and additionally can lead to over or under treatment compared with clinical guidelines. For example, in high exacerbation risk settings, the clinical utility of the use of COPD exacerbation history as a single predictor might be lower than providing all patients with therapies with low risk of adverse events and relatively inexpensive (eg, long-acting muscarinic receptor antagonist and long-acting β2 agonists therapy). The only clinical scenario in which COPD exacerbation history alone was found to be clinically useful (ie, providing higher net benefit than not using any risk stratification) was when therapies with high treatment thresholds associated with significant side effects, such as azithromycin or oral roflumilast, were considered. Next, the authors assessed the impact of recalibration based on the background exacerbation risk of the population being considered. After recalibration, both prediction models were no longer associated with risk of harm and were mostly superior to exacerbation history alone. As a strength, the study evaluated the performance of existing prediction models in populations with different background exacerbation risks with the use of well-characterized and studied databases. At the same time, the information presented in the article is complex, which may impact the understanding of implications in the general audience. Furthermore, the ACCEPT model is not easier to apply in clinical practice, given the number of variables. Further recalibration on baseline exacerbation risk further complicates the use of such tools in clinical care. Fortunately, wide implementation of Electronic Medical Records, natural language processing, and machine learning to incorporate both unstructured (narrative) clinical notes and structured electronic health records14Tsui F.R. Shi L. Ruiz V. et al.Natural language processing and machine learning of electronic health records for prediction of first-time suicide attempts.JAMIA Open. 2021; 4: ooab011Crossref PubMed Scopus (17) Google Scholar can help with such complexity but requires relevant knowledge. Another study limitation is that both multivariable prediction models incorporate cardiovascular risk factors into their risk prediction, which may reduce discrimination ability in Study to Understand Mortality and Morbidity in COPD patient population, given inclusion criteria. Importantly, only two prediction models were evaluated, which affected generalizability. For example, the article that the authors were inspired by tested more than 100 cardiovascular disease clinical prediction models’ performance, including clinical utility.9Gulati G. Upshaw J. Wessler B.S. et al.Generalizability of cardiovascular disease clinical prediction models: 158 independent external validations of 104 unique models.Circ Cardiovasc Qual Outcomes. 2022; 15e008487Crossref Scopus (15) Google Scholar Thus, Ho et al10Ho J.K. Safari A. Adibi A. et al.Generalizability of risk stratification algorithms for exacerbations in COPD.Chest. 2023; 163: 790-798Abstract Full Text Full Text PDF PubMed Scopus (2) Google Scholar have shown that one size does not fit all in prediction, and prediction models cannot be universally clinically applicable. Multivariable prediction models need to be flexible and adjustable, based on the overall exacerbation risk for optimal clinical implementation. COPD exacerbation history alone is not good enough to inform treatment choices in all clinical settings. Multivariable prediction models may have higher accuracy and superior predictive performance, but they require external validation and setting-specific recalibration to provide clinical utility before being deployed in a new clinical setting. Further studies are needed to examine more prediction models, to potentially develop a better prediction model than existing and strategies to further improve model predictive performance and generalizability. Finally, this is critical to implement prediction models clinically, considering machine learning and the use of electronic medical records systems. None declared. Generalizability of Risk Stratification Algorithms for Exacerbations in COPDCHESTVol. 163Issue 4PreviewExacerbation history alone is unlikely to provide clinical utility for predicting COPD exacerbations in all settings and could be associated with a risk of harm. Prediction models have superior predictive performance, but require setting-specific recalibration to confer higher clinical utility. Full-Text PDF
更多查看译文
关键词
size,one
AI 理解论文
溯源树
样例
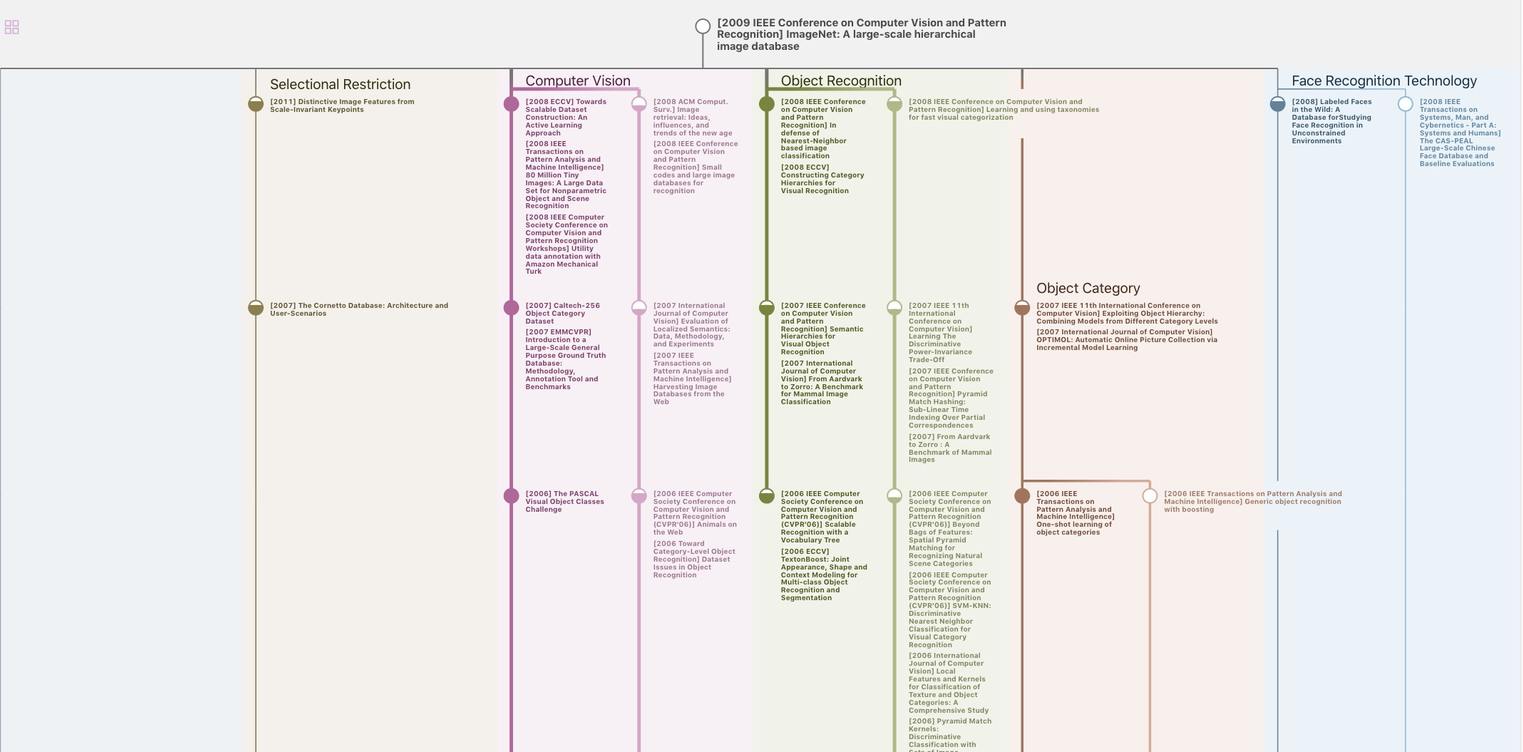
生成溯源树,研究论文发展脉络
Chat Paper
正在生成论文摘要