APPT : Asymmetric Parallel Point Transformer for 3D Point Cloud Understanding
arXiv (Cornell University)(2023)
摘要
Transformer-based networks have achieved impressive performance in 3D point cloud understanding. However, most of them concentrate on aggregating local features, but neglect to directly model global dependencies, which results in a limited effective receptive field. Besides, how to effectively incorporate local and global components also remains challenging. To tackle these problems, we propose Asymmetric Parallel Point Transformer (APPT). Specifically, we introduce Global Pivot Attention to extract global features and enlarge the effective receptive field. Moreover, we design the Asymmetric Parallel structure to effectively integrate local and global information. Combined with these designs, APPT is able to capture features globally throughout the entire network while focusing on local-detailed features. Extensive experiments show that our method outperforms the priors and achieves state-of-the-art on several benchmarks for 3D point cloud understanding, such as 3D semantic segmentation on S3DIS, 3D shape classification on ModelNet40, and 3D part segmentation on ShapeNet.
更多查看译文
关键词
asymmetric parallel point transformer,3d point cloud
AI 理解论文
溯源树
样例
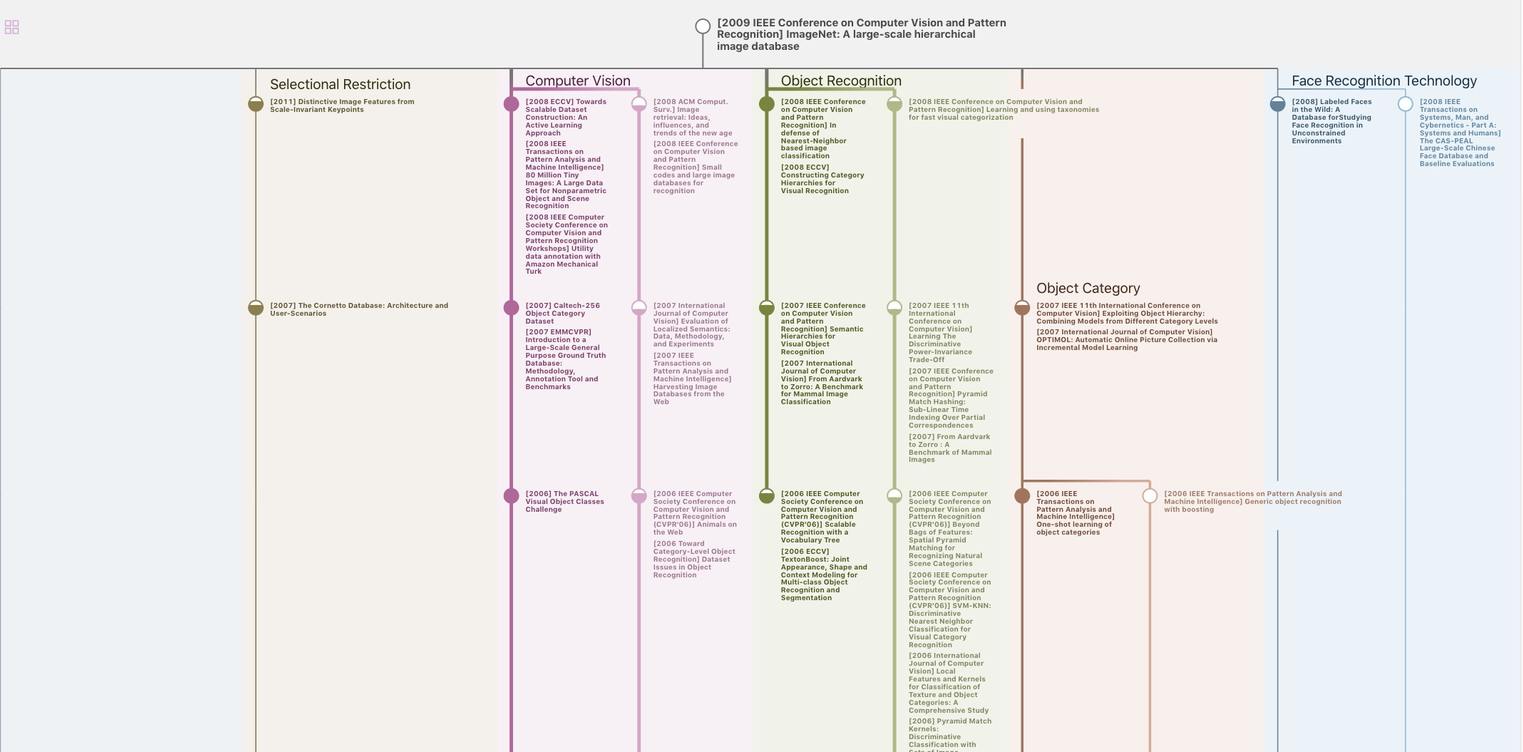
生成溯源树,研究论文发展脉络
Chat Paper
正在生成论文摘要