Federated Auto-weighted Domain Adaptation
arXiv (Cornell University)(2023)
摘要
Federated Domain Adaptation (FDA) describes the federated learning setting where a set of source clients work collaboratively to improve the performance of a target client where limited data is available. The domain shift between the source and target domains, coupled with sparse data in the target domain, makes FDA a challenging problem, e.g., common techniques such as FedAvg and fine-tuning, often fail with the presence of significant domain shift and data scarcity. To comprehensively understand the problem, we introduce metrics that characterize the FDA setting and put forth a theoretical framework for analyzing the performance of aggregation rules. We also propose a novel aggregation rule for FDA, Federated Gradient Projection ($\texttt{FedGP}$), used to aggregate the source gradients and target gradient during training. Importantly, our framework enables the development of an $\textit{auto-weighting scheme}$ that optimally combines the source and target gradients. This scheme improves both $\texttt{FedGP}$ and a simpler heuristic aggregation rule ($\texttt{FedDA}$). Experiments on synthetic and real-world datasets verify the theoretical insights and illustrate the effectiveness of the proposed method in practice.
更多查看译文
关键词
adaptation,domain,auto-weighted
AI 理解论文
溯源树
样例
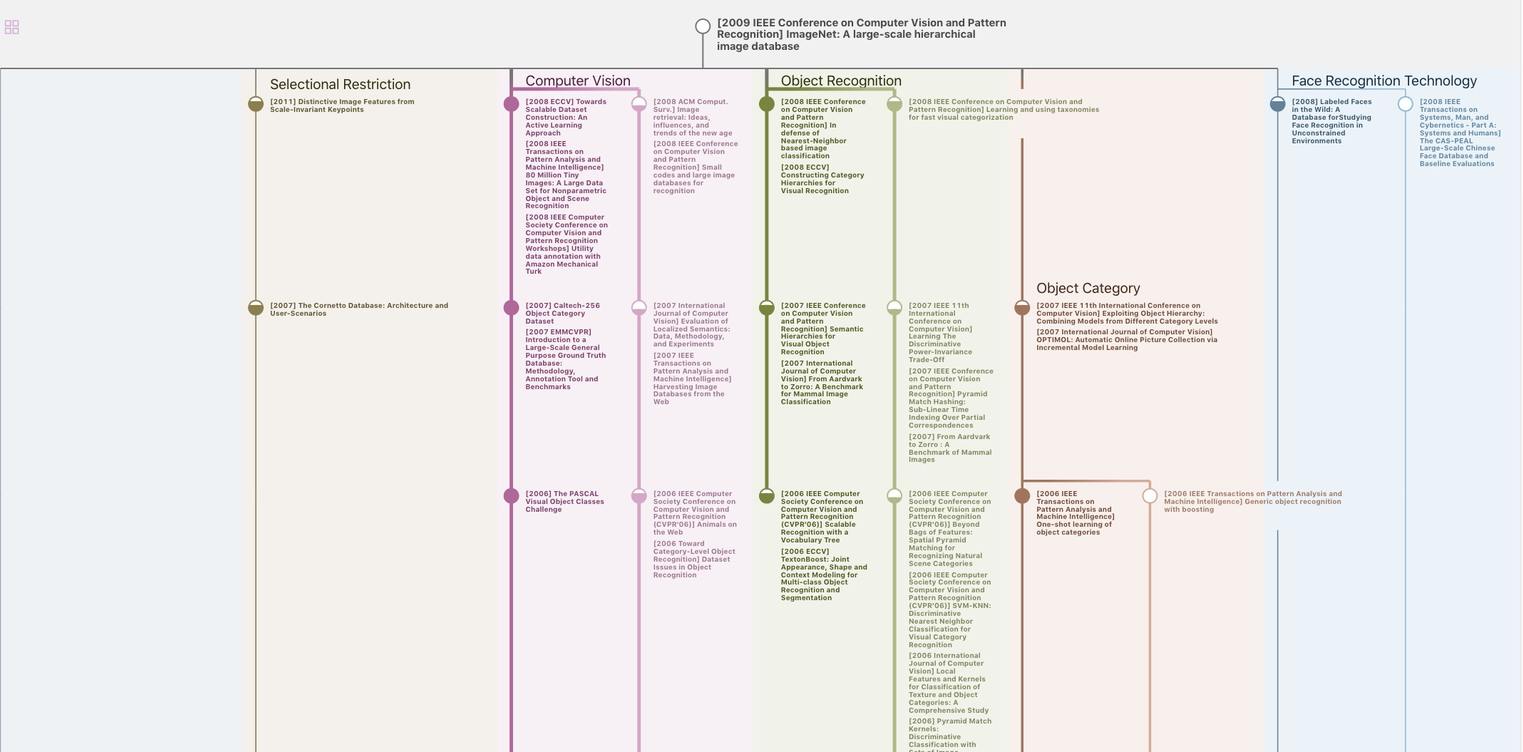
生成溯源树,研究论文发展脉络
Chat Paper
正在生成论文摘要