Convolutional Learning on Simplicial Complexes
arXiv (Cornell University)(2023)
摘要
We propose a simplicial complex convolutional neural network (SCCNN) to learn data representations on simplicial complexes. It performs convolutions based on the multi-hop simplicial adjacencies via common faces and cofaces independently and captures the inter-simplicial couplings, generalizing state-of-the-art. Upon studying symmetries of the simplicial domain and the data space, it is shown to be permutation and orientation equivariant, thus, incorporating such inductive biases. Based on the Hodge theory, we perform a spectral analysis to understand how SCCNNs regulate data in different frequencies, showing that the convolutions via faces and cofaces operate in two orthogonal data spaces. Lastly, we study the stability of SCCNNs to domain deformations and examine the effects of various factors. Empirical results show the benefits of higher-order convolutions and inter-simplicial couplings in simplex prediction and trajectory prediction.
更多查看译文
关键词
complexes,learning
AI 理解论文
溯源树
样例
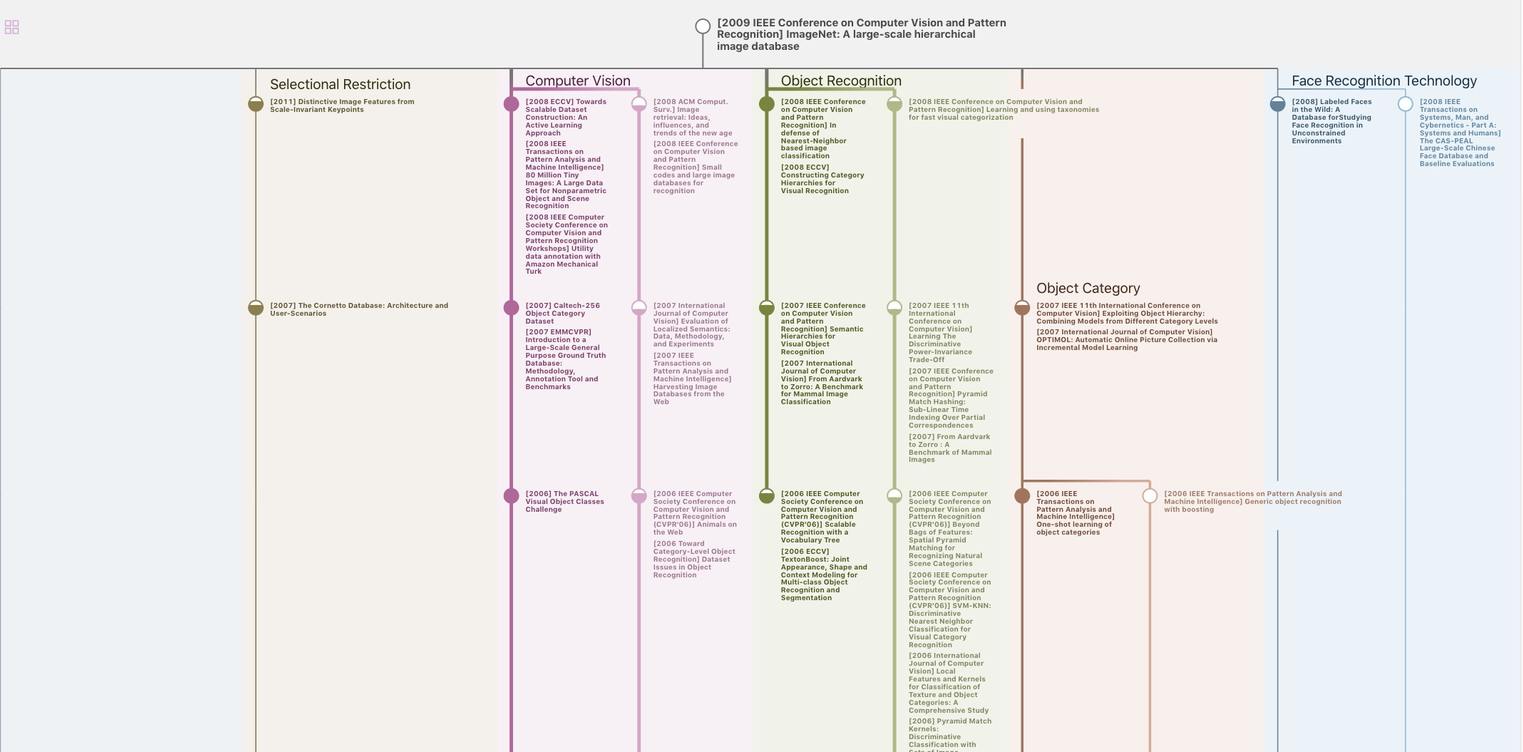
生成溯源树,研究论文发展脉络
Chat Paper
正在生成论文摘要