Modeling cross-cell typecis-regulatory patterns via hierarchical deep neural network and gene expression prediction
bioRxiv (Cold Spring Harbor Laboratory)(2023)
摘要
Abstract Linking cis -regulatory sequences to target genes has been a long-standing challenge. In this study, we introduce CREaTor, an attention-based deep neural network designed to model cis -regulatory patterns for genomic elements up to 2Mb from target genes. Coupled with a training strategy that predicts gene expression from flanking candidate cis -regulatory elements (cCREs), CREaTor can model cell type-specific cis -regulatory patterns in new cell types without prior knowledge of cCRE-gene interactions or additional training. The zero-shot modeling capability, combined with the use of RNA-seq and ChIP-seq data only, allows for the readily generalization of CREaTor to a broad range of cell types. Evaluation reveals that CREaTor outperforms existing methods in capturing cCRE-gene interactions across various distance ranges in held-out cell types. Further analysis indicates that the superior performance of CREaTor can be attributed to its capacity to model regulatory interactions at multiple levels, including the higher-order genome organizations that govern cCRE activities as well as cCRE-gene interactions. Collectively, our findings highlight CREaTor as a powerful tool for systematically investigating cis -regulatory programs across various cell types, both in normal developmental processes and disease-associated contexts.
更多查看译文
关键词
gene expression prediction,gene expression,hierarchical deep neural network,cross-cell
AI 理解论文
溯源树
样例
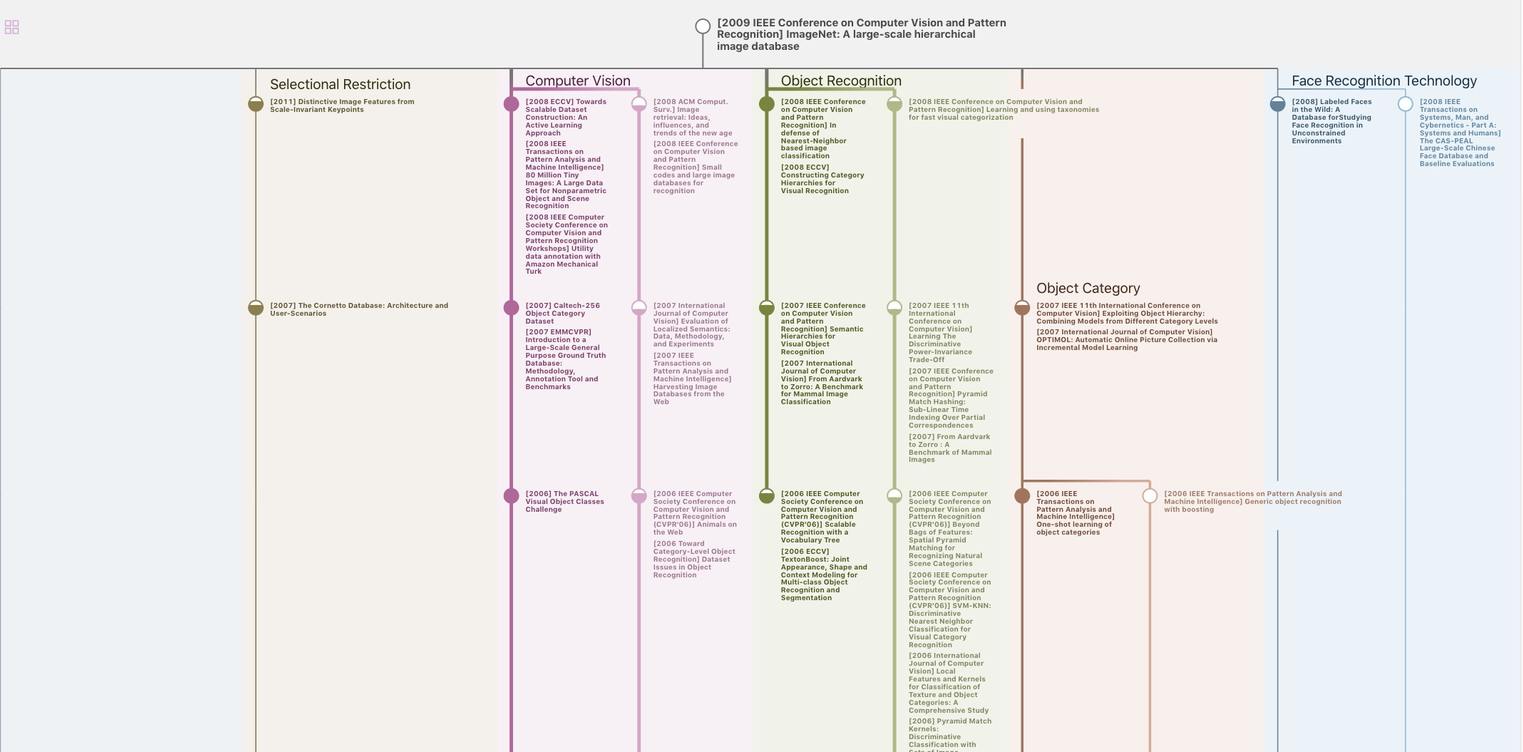
生成溯源树,研究论文发展脉络
Chat Paper
正在生成论文摘要