Model-Guided Generative Adversarial Networks for Unsupervised Fine-Grained Image Generation
IEEE TRANSACTIONS ON MULTIMEDIA(2024)
摘要
Unsupervised fine-grained image generation is a challenging issue in computer vision. Although many recent significant advances have improved performance, the ability to synthesize photo-realistic images in an unsupervised manner remains extremely difficult. The existing methods compose an image via complex three-stage generative adversarial networks and impose constraints between the latent codes. This pipeline focuses on the disentanglement and ignores the quality of generated images. In this article, we propose a novel two-stage approach for unsupervised fine-grained image generation, termed Model-Guided Generative Adversarial Networks (MG-GAN). We introduce an attention module for exploring the correlation between fine-grained latent codes and image features in the foreground generation stage. The attention module enables the network to automatically focus on the color details and semantic concepts of objects related to different fine-grained classes. Furthermore, we incorporate knowledge distillation strategy and design a simple but effective inverse background image generator as a teacher to guide the background image generation. With the help of knowledge learned in the pre-trained inverse background image generator, a comfortable canvas is synthesized and combined with foreground object more reasonably. Extensive experiments on three popularly fine-grained datasets demonstrate that our approach achieves state-of-the-art performance and is even competitive with semi-supervised method.
更多查看译文
关键词
Unsupervised fine-grained image generation,generative adversarial networks,attention mechanism,image inpainting
AI 理解论文
溯源树
样例
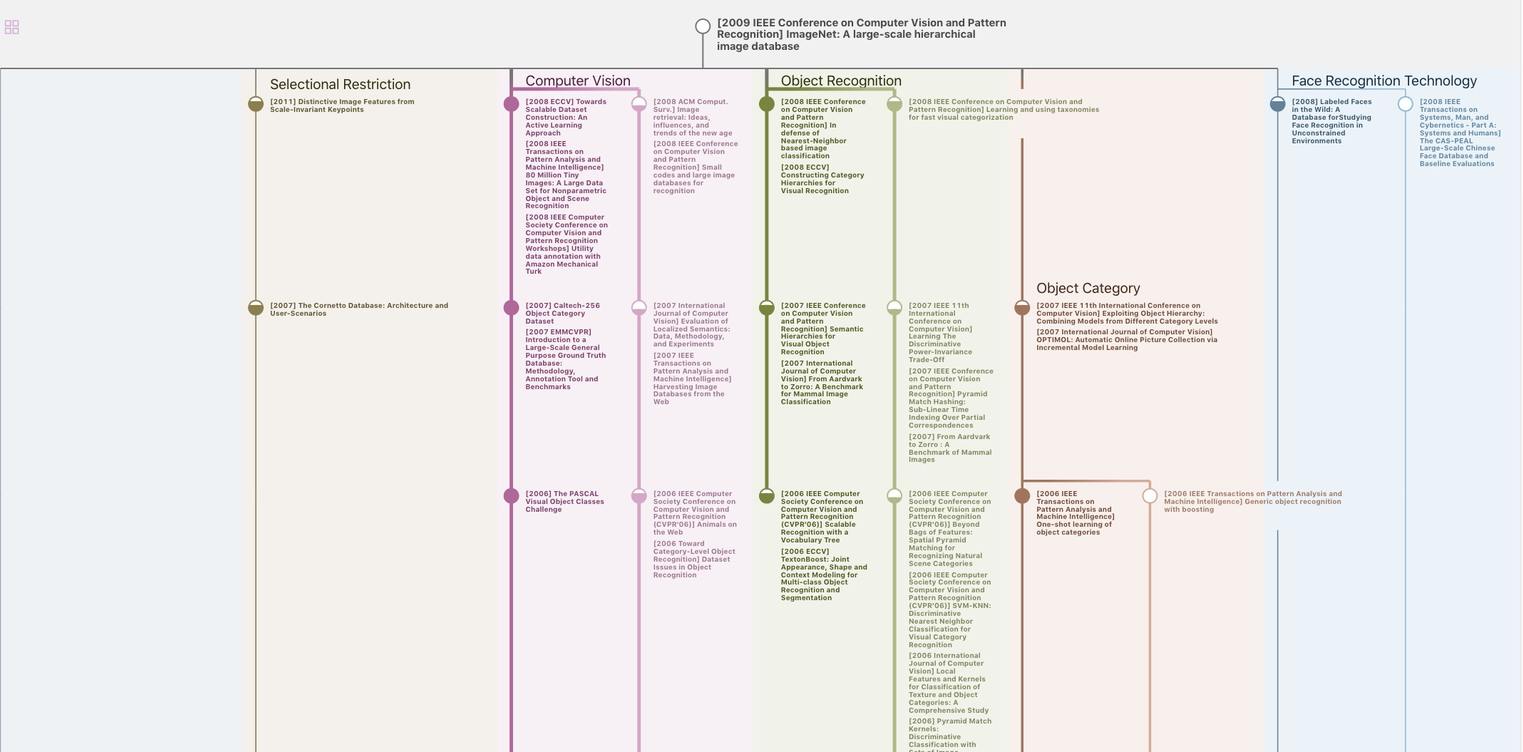
生成溯源树,研究论文发展脉络
Chat Paper
正在生成论文摘要