Abstract P6-01-18: Predicting response of triple negative breast cancer to neoadjuvant chemotherapy using a deep convolutional neural network-based artificial intelligence tool
Cancer Research(2023)
摘要
Abstract Background: Triple-negative breast cancer (TNBC) is commonly treated with neoadjuvant chemotherapy (NAC). Pathologic complete response (pCR) to NAC is associated with improved patient outcomes. The ability to predict which patients have high likelihood to achieve pCR has important clinical implications. We developed and validated a deep convolutional neural network (CNN)–based artificial intelligence (AI) model to extract morphometric features of TNBC to predict response to NAC. Methods: Whole-slide images (WSIs) of hematoxylin and eosin–stained core biopsies of 165 (pathologic complete response [pCR] in 60 and non-pCR in 105) and 78 (pCR in 31 and non-pCR in 47) TNBC patients, respectively, were used for training and validation of the model. The model extracted morphometric features from WSIs in an unsupervised way and transformed the image tiles from WSIs into high-dimensional vectors, generating clusters of morphologically similar patterns. Downstream ranking of clusters using neural networks provided regions of interest with high or low predictive value for NAC response. Morphometric scores combined with clinical TNM stage gave AI prediction scores; a low score close to 0 and high score close to 1, respectively, represented a high or low probability of pCR, respectively. Results: The predictive ability of the AI score for the entire cohort of 78 TNBC patients ascertained by receiver operating characteristic (ROC) analysis demonstrated area under the curve (AUC) of 75.5%. The AUC for stage I, II, and III disease was 88.1%, 73.7%, and 74.7% respectively. The performance of the AI scores was also analyzed based on their distribution into quartiles. Patients in the highest score quartile were predicted to not have pCR and those in the lowest score quartile were predicted to have pCR. Of the 20 patients in the lowest score quartile, 15 experienced pCR yielding a positive predictive value of the AI score for pCR of 75%. Of the 20 patients in the highest score quartile, 16 did not have pCR, yielding a negative predictive value of 80%. Conclusions: This is the first demonstration of using an AI tool to predict response to NAC in patients with TNBC. These results if validated in subsequent studies, could inform individualized decisions regarding intensity of NAC, including options to de-escalate NAC in patients with TNBC who are likely to achieve pCR. Citation Format: Savitri Krishnamurthy, Parag Jain, Debu Tripathy, Hassan Muhammad, Wei huang, Hua Yang, Shivaani Kummar, George Wilding, Rajat Roy, Ramandeep Randhawa. Predicting response of triple negative breast cancer to neoadjuvant chemotherapy using a deep convolutional neural network-based artificial intelligence tool [abstract]. In: Proceedings of the 2022 San Antonio Breast Cancer Symposium; 2022 Dec 6-10; San Antonio, TX. Philadelphia (PA): AACR; Cancer Res 2023;83(5 Suppl):Abstract nr P6-01-18.
更多查看译文
关键词
triple negative breast cancer,neoadjuvant chemotherapy,breast cancer,network-based
AI 理解论文
溯源树
样例
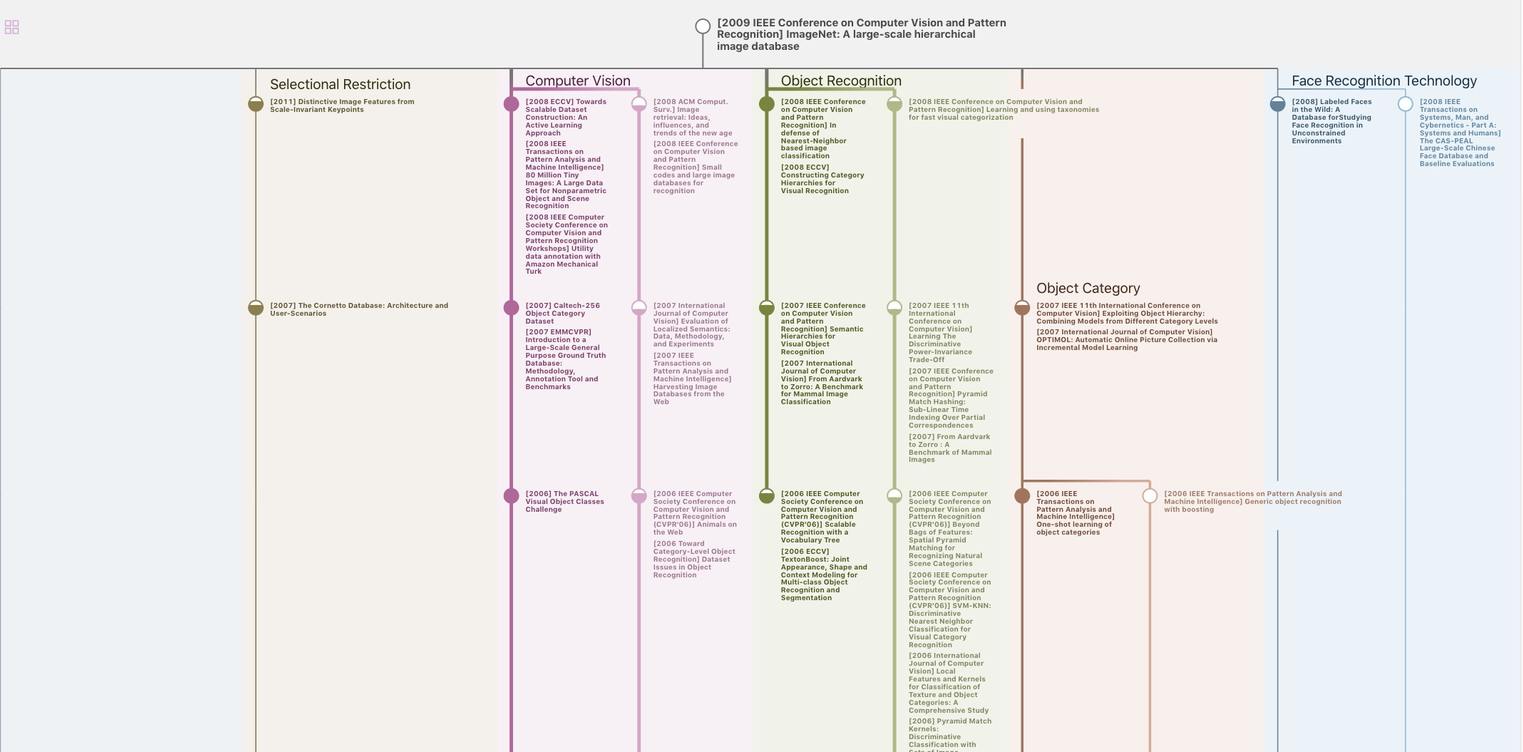
生成溯源树,研究论文发展脉络
Chat Paper
正在生成论文摘要