Task-Aware Adversarial Feature Perturbation for Cross-Domain Few-Shot Learning
ARTIFICIAL NEURAL NETWORKS AND MACHINE LEARNING, ICANN 2023, PT III(2023)
摘要
Currently, metric-based meta-learning methods have achie ved great success in few-shot learning (FSL). However, most works assume a high similarity between base classes and novel classes, and their performance can be greatly reduced when comes to domain-shift problem. As a result, cross-domain few-shot learning (CD-FSL) methods are proposed to tackle the domain-shift problem, which places a higher demand on the robustness of the meta-knowledge. To this end, we propose a feature augmentation method called Task-Aware Adversarial Feature Perturbation (TAAFP) to improve the generalization of the existing FSL models. Compared to the traditional adversarial training, our adversarial perturbations are generated from the feature space and contain more sample relationship information, which is discovered by the Task Attention Module. Task Attention Module is designed based on a transformer to capture more discriminative features in a task. Therefore, our perturbations can easily attack the extraction process for discriminative features, forcing the model to extract more robust discriminative features. In addition, a regularization loss is introduced to ensure the predictions of the adversarial augmented task remain similar to the original task. We conduct extensive classification experiments in five datasets under the setting of cross-domain few-shot classification. The result shows that our method can significantly improve the classification accuracy in both seen and unseen domains.
更多查看译文
关键词
Few-shot learning,Cross-domain few-shot learning,Task-Aware Adversarial Feature Perturbation
AI 理解论文
溯源树
样例
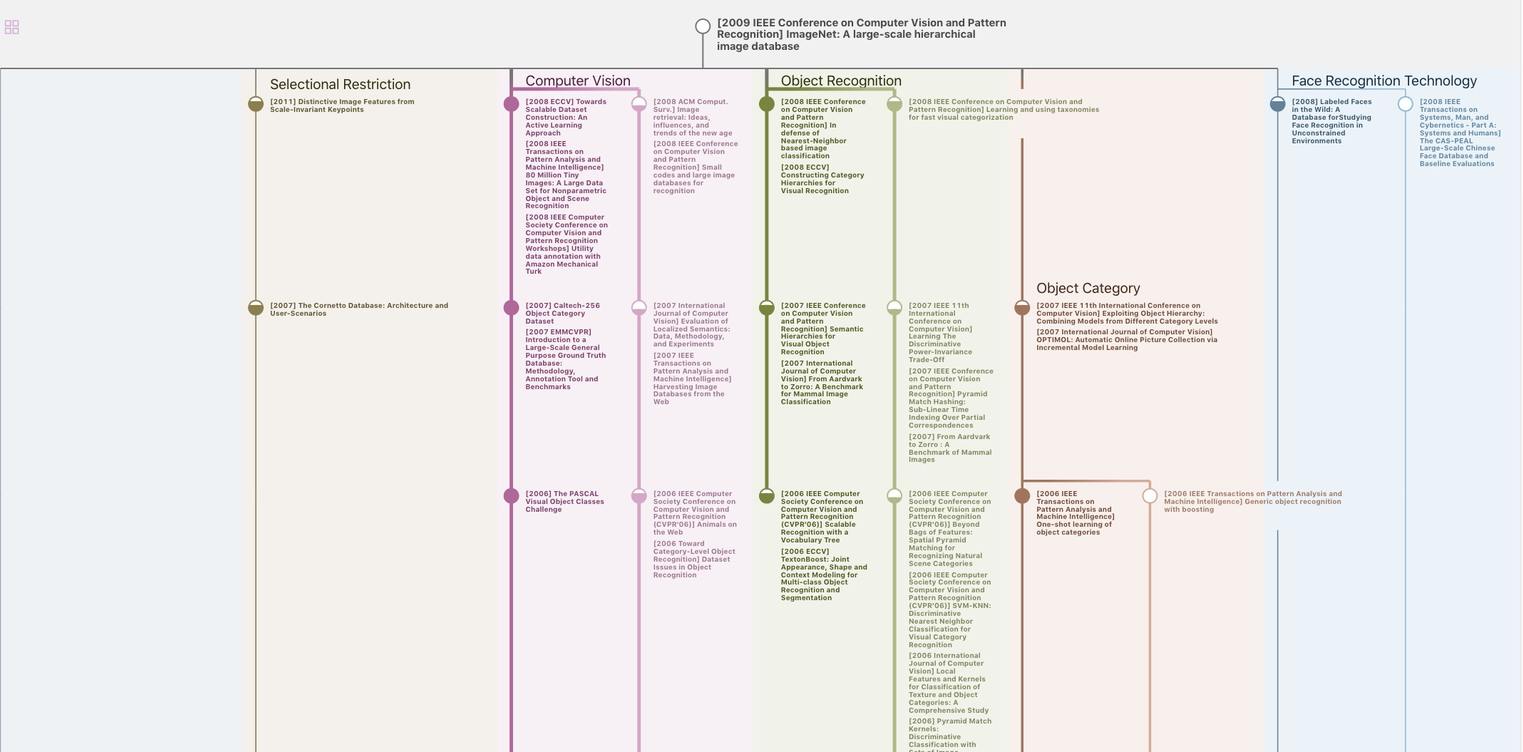
生成溯源树,研究论文发展脉络
Chat Paper
正在生成论文摘要