Traffic Flow Prediction Based on Multi-type Characteristic Hybrid Graph Neural Network
ARTIFICIAL NEURAL NETWORKS AND MACHINE LEARNING, ICANN 2023, PT V(2023)
摘要
In the traffic flow prediction task, how to obtain the complex spatial-temporal correlation in the traffic data is a hard nut to crack. This paper proposes a novel Multi-Type Characteristics Hybrid Graph Neural Network (MTCHGNN) to capture these complex dependencies. First, previous studies have shown that limited representations of given graph structures with incomplete relationships may restrict the performance of those models. So we construct the novel graph generation module to obtain a multi-type characteristic hybrid graph structure, which can not only describe the short-term spatial-temporal relationships between nodes in the traffic road network but also be effective for long-term dynamic changes with specific road conditions. Second, our spatial-temporal feature extraction architecture utilizes a multi-semantic graph attention network to capture features in every time step, which can discriminatively aggregate the information with multiple receptive fields according to the complex spatial-temporal dependencies between nodes. We evaluate our proposed approach on real-world datasets, and superior performance is achieved over state-of-the-art baselines.
更多查看译文
关键词
Spatial-Temporal,Graph Neural Network,Traffic forecasting
AI 理解论文
溯源树
样例
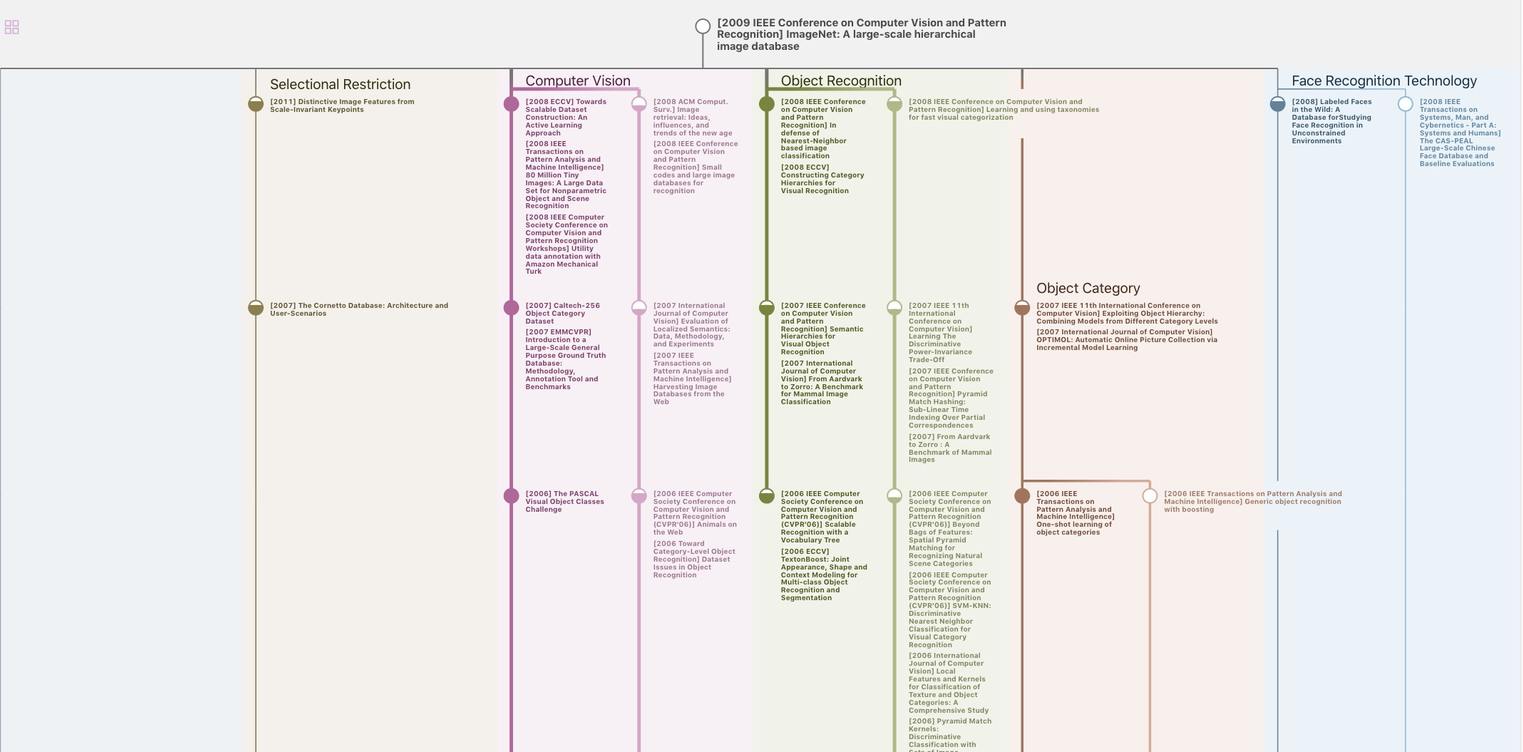
生成溯源树,研究论文发展脉络
Chat Paper
正在生成论文摘要