Label-Description Enhanced Network for Few-Shot Named Entity Recognition
ARTIFICIAL NEURAL NETWORKS AND MACHINE LEARNING, ICANN 2023, PT VIII(2023)
摘要
As one of the essential tasks in the context of natural language understanding, Few-shot Named Entity Recognition (NER) aims to identify and classify entities against limited samples. Recently, many works have attempted to enhance semantic representations by constructing prompt templates with text and label names. These methods, however, not only distract attention from the text, but also cause unnecessary enumerations. Furthermore, ambiguous label names always fail in delivering the intended meaning. To address the above issues, we present a Label-Description Enhanced Network (LaDEN) for few-shot named entity recognition, under which we propose a BERT-based Siamese network to incorporate fine-grained label descriptions as knowledge augmentation. The designed semantic attention mechanism captures label-specific textual representations, and the distance function matches similar token and label representations based on the nearest-neighbor criterion. Experimental results demonstrate that our model outperforms previous works in both few-shot and resource-rich settings, achieving state-of-the-art performance on five benchmarks. Our method is particularly efficient in low-resource scenarios, especially for cross-domain applications.
更多查看译文
关键词
Named Entity Recognition,Few-shot Setting,Domain Adaptation
AI 理解论文
溯源树
样例
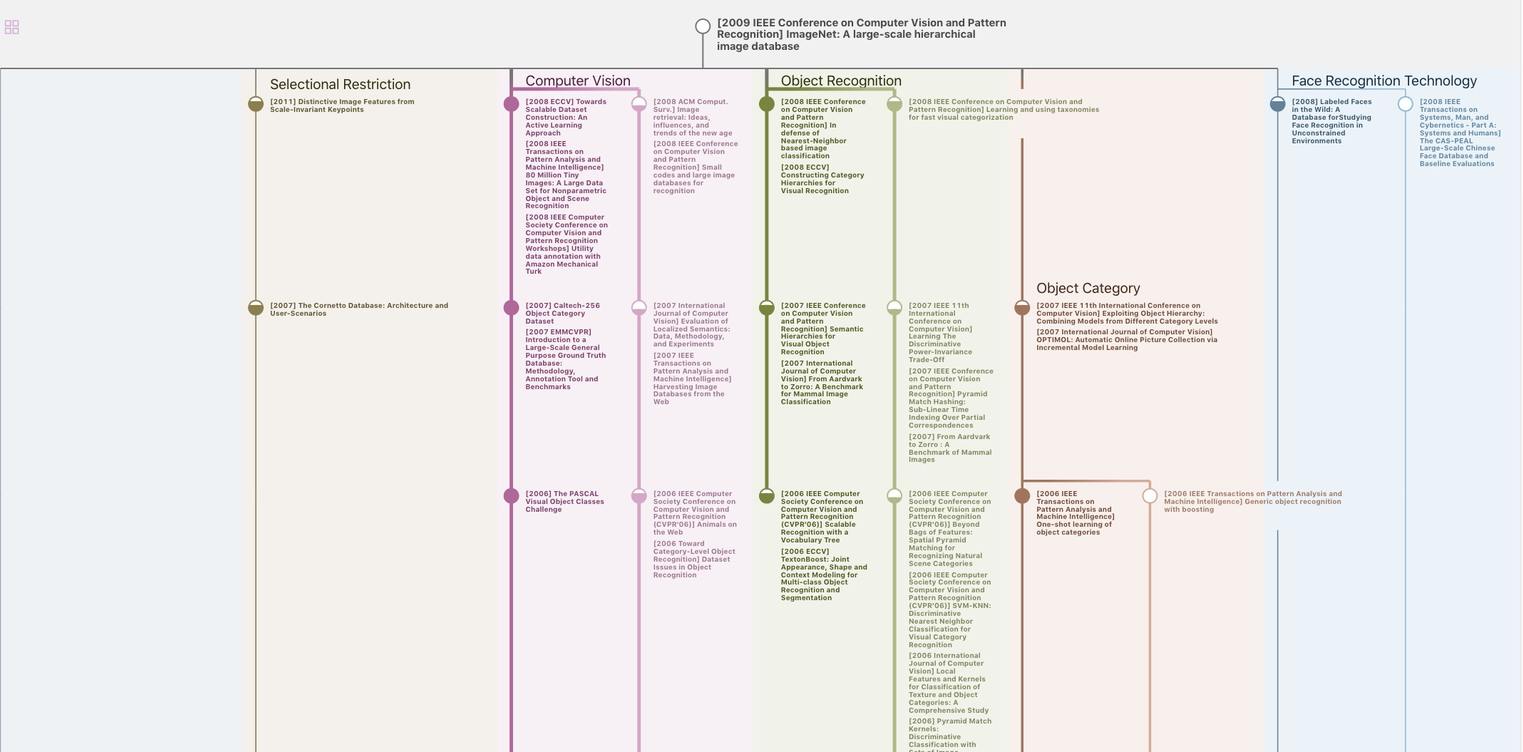
生成溯源树,研究论文发展脉络
Chat Paper
正在生成论文摘要