IEC-TPC: An Imputation Error Cluster-Based Approach for Energy Optimization in IoT Data Transmission Period Control.
IEEE Internet Things J.(2024)
摘要
In a variety of Internet of Things (IoT) applications, there is a growing need to constantly transmit large amounts of real-time data from IoT sensors to enable precise environmental monitoring. However, this constant transmission of data can result in high energy consumption of IoT sensors, which presents a significant challenge for IoT systems. Therefore, this paper presents a new approach for controlling the transmission period of IoT sensors called Imputation Error Cluster-based Transmission Period Control (IEC-TPC) framework, with the goal of reducing energy consumption while maintaining accurate data collection. In order to effectively balance energy consumption and data collection accuracy, the proposed approach uses an Imputation Error Centroid (IEC) model that forms clusters based on imputation error vectors and approximates the centroid of each cluster using a logistic function. Additionally, a new Imputation Error Cluster Prediction (IECP) model is designed using Long Short Term Memory (LSTM)-based encoding and Convolutional Neural Network (CNN) models to predict the label of each cluster in advance. By combining the IEC model with a numerically modeled energy consumption function, a min-max optimization problem is properly formulated to minimize the worst-case performance of transmission period control. To find the optimal solution, a Uniqueness Condition function is properly defined, and an Optimal Objective Value Searching Algorithm (O2VSA) leveraging a bisection search is proposed. Performance evaluation shows that the proposed method achieves similar data collection accuracy in CO2 and humidity datasets while reducing energy consumption by 12.92% and 35.83% than other baseline models, respectively. Moreover, the proposed algorithm outperforms the state-of-the-art method in the temperature dataset, with 13% lower energy consumption value. The proposed method also achieves a significant reduction in energy consumption of 95.21% compared to the uniform transmission period model in this dataset, while maintaining a low data reconstruction error of only 0.42%. Overall, the proposed method offers a promising approach for efficient and accurate transmission period control in IoT applications.
更多查看译文
关键词
Internet of Things,Energy consumption,Machine learning,LSTM,CNN,Min-max problem,Bisection search
AI 理解论文
溯源树
样例
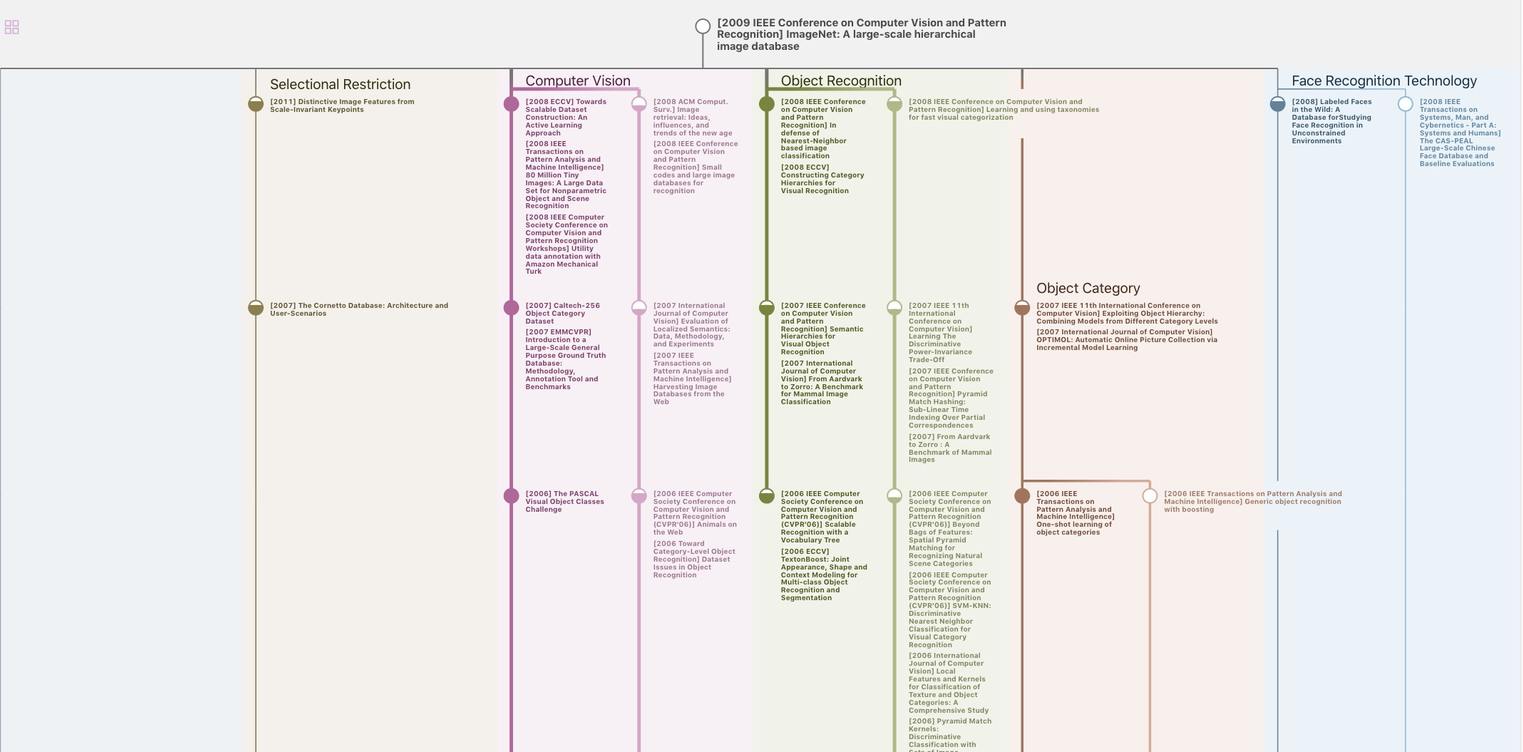
生成溯源树,研究论文发展脉络
Chat Paper
正在生成论文摘要