When Channel Correlation Meets Sparse Prior: Keeping Interpretability in Image Compressive Sensing
IEEE TRANSACTIONS ON MULTIMEDIA(2024)
摘要
Image compressive sensing (CS), recovering an unknown image by resorting to a small number of its measurements, has become an increasingly popular topic in multimedia technology and applications. For a better reconstruction, diverse priors, from the original sparse prior to the new deep prior, have been exploited. Despite the powerful learning capability and satisfactory reconstruction performance, the deep prior is known as a black box and loses clear interpretability. In this article, we first revisit image CS with different priors and observe that the method with hand-crafted sparse prior could still outperform state-of-art methods with deep prior or no prior when under the same settings, while the interpretability is well preserved. Then, towards a better performance of the sparse-prior-based method, we propose a Channel Adaptive Thresholding Network, namely CAT-Net. CAT-Net draws the support from channel correlation calculation to extend the single thresholding in the iterative soft thresholding algorithm (ISTA) into channel-wise thresholding. The channel adaptive thresholding conducts soft thresholding operation in each channel of the image features and can be adjusted adaptively to the inputs, which can reconstruct more precisely than a single static thresholding. The careful CAT operation can preserve patterns both in detail and holistically well. Experimental results demonstrate the proposed method outperforms the state-of-the-art image CS methods with both traditional and deep priors.
更多查看译文
关键词
Image reconstruction,Correlation,Transforms,Indexes,Thresholding (Imaging),Optimization,Iterative algorithms,Channel correlation,compressive sensing,deep prior,sparse prior
AI 理解论文
溯源树
样例
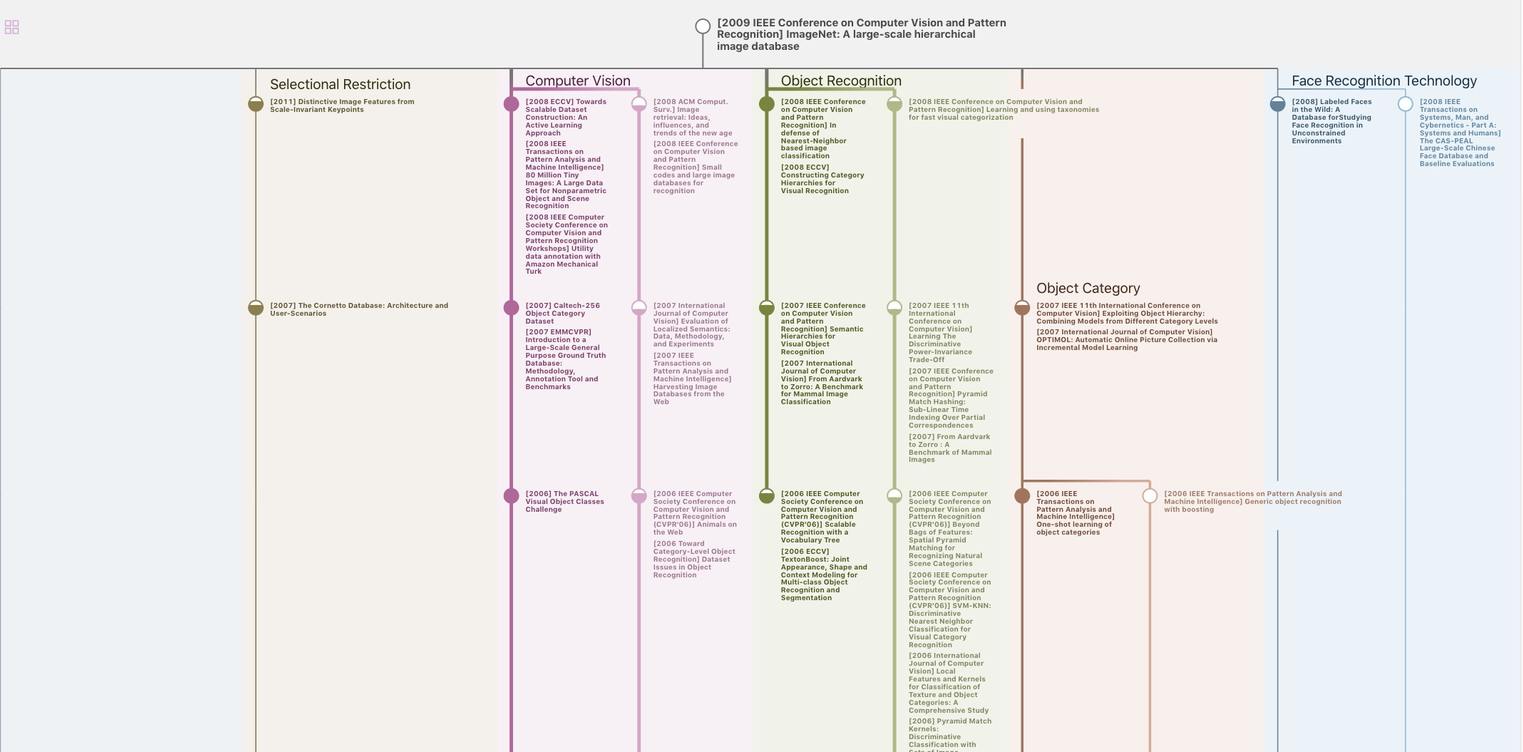
生成溯源树,研究论文发展脉络
Chat Paper
正在生成论文摘要