Structure Aware Multi-Graph Network for Multi-Modal Emotion Recognition in Conversations
IEEE TRANSACTIONS ON MULTIMEDIA(2024)
摘要
Multi-Modal Emotion Recognition in Conversations (MMERC) is an increasingly active research field that leverages multi-modal signals to understand the feelings behind each utterance. Modeling contextual interactions and multi-modal fusion lie at the heart of this field, with graph-based models recently being widely used for MMERC to capture global multi-modal contextual information. However, these models generally mix all modality representations in a single graph, and utterances in each modality are fully connected, potentially ignoring three problems: 1) the heterogeneity of the multi-modal context, 2) the redundancy of contextual information, and 3) over-smoothing of the graph networks. To address these problems, we propose a Structure Aware Multi-Graph Network (SAMGN) for MMERC. Specifically, we construct multiple modality-specific graphs to model the heterogeneity of the multi-modal context. Instead of fully connecting the utterances in each modality, we design a structure learning module that determines whether edges exist between the utterances. This module reduces redundancy by forcing each utterance to focus on the contextual ones that contribute to its emotion recognition, acting like a message propagating reducer to alleviate over-smoothing. Then, we develop the SAMGN via Dual-Stream Propagation (DSP), which contains two propagation streams, i.e., intra- and inter-modal, performed in parallel to aggregate the heterogeneous modality information from multi-graphs. DSP also contains a gating unit that adaptively integrates the co-occurrence information from the above two propagations for emotion recognition. Experiments on two popular MMERC datasets demonstrate that SAMGN achieves new State-Of-The-Art (SOTA) results.
更多查看译文
关键词
Emotion recognition,Context modeling,Feature extraction,Visualization,Acoustics,Oral communication,Transformers,Structure learning,multi-graph network,dual-stream propagations,multi-modal fusion,emotion recognition in conversations
AI 理解论文
溯源树
样例
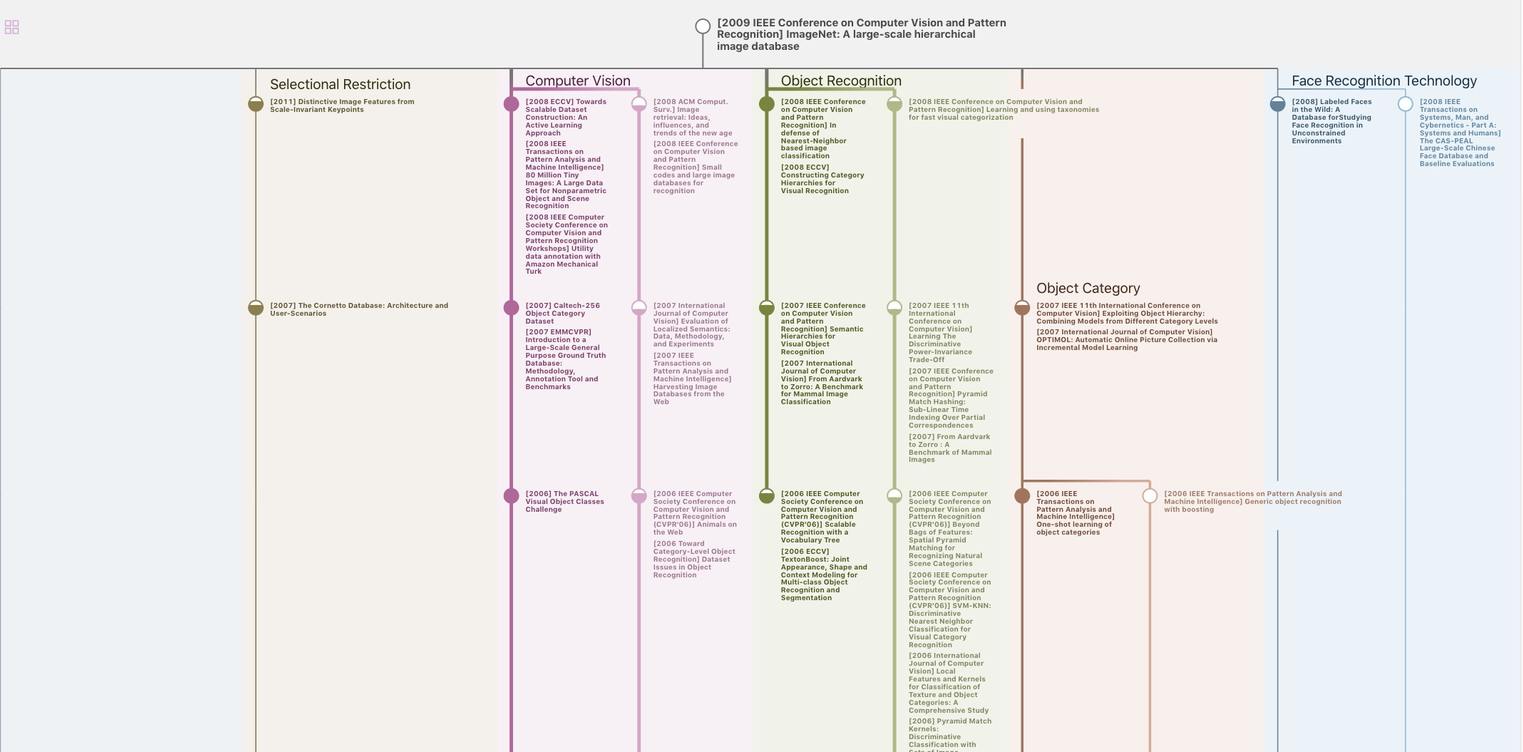
生成溯源树,研究论文发展脉络
Chat Paper
正在生成论文摘要