Cross-Task Multimodal Reinforcement for Long Tail Next POI Recommendation
IEEE TRANSACTIONS ON MULTIMEDIA(2024)
摘要
Next Point-of-Interest (POI) recommendation seeks to recommend locations that users are most likely to visit next based on their historical trajectories, providing both users and service providers with substantial benefits. However, most next POI recommendation methods calculate the distances between POIs when mining spatial information and adjust their weights accordingly, ignoring the characteristics and multimedia content features of the regions in which POIs are located. In addition, the next POI recommendations suffer from the long tail effect, in which only a small portion of POIs appear frequently in users' recommendation lists due to their high popularity, while remainders maintain a low presence. To this end, we propose the cross-task multimodal reinforcement method which enriches the representations of regions by incorporating information from auxiliary domains. Moreover, we devise a cross-task reinforcement module to effectively integrate the local representations with pre-trained encoders from auxiliary domains. Actually, the enhanced region representations contain constructive district properties which are helpful to find proper POIs that suit users' tastes and thus alleviate the long tail effect. Experiments conducted on two real-world datasets indicate that our proposed method outperforms the state-of-the-art models in terms of both general performance and that of niche POIs.
更多查看译文
关键词
Next POI recommendation,multimodal,long tail,cross-task
AI 理解论文
溯源树
样例
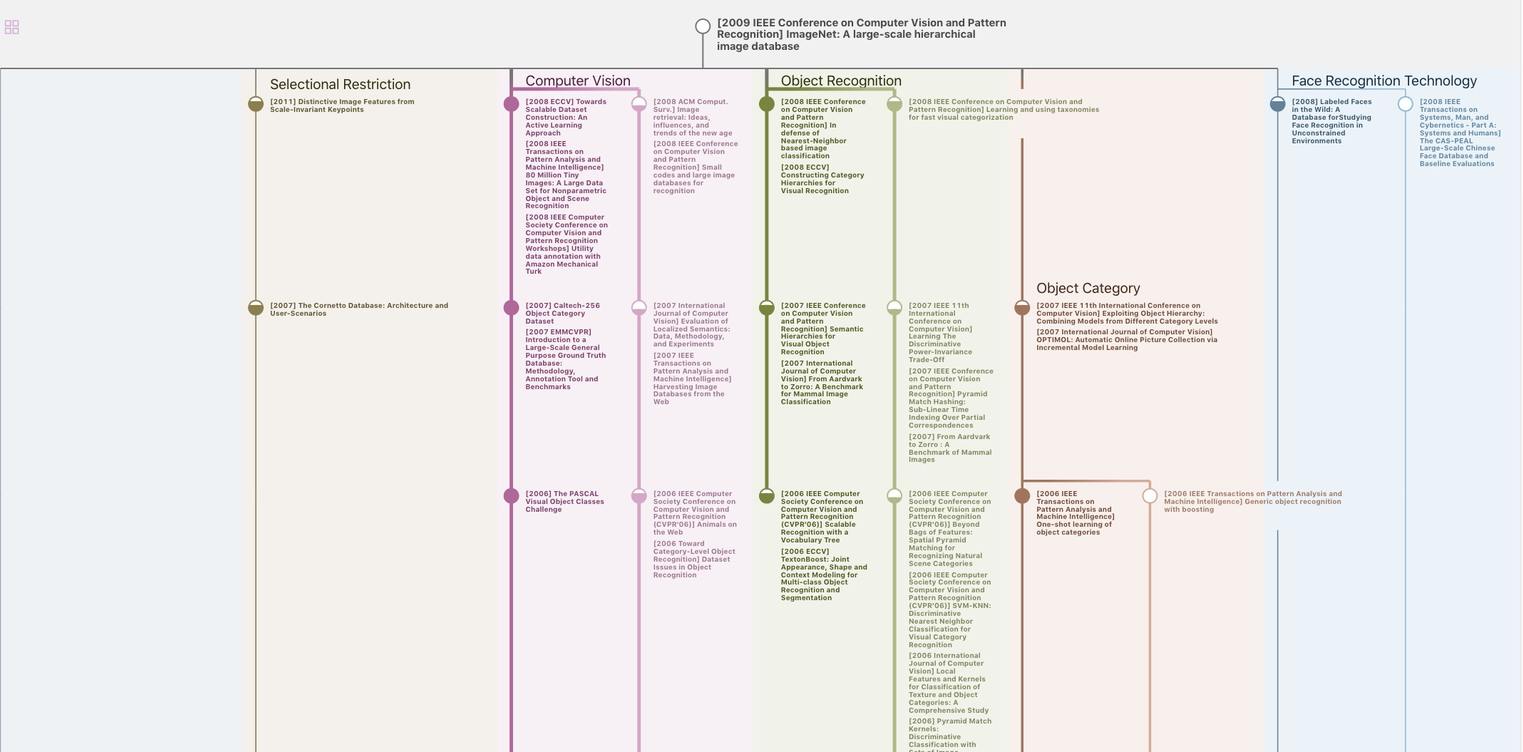
生成溯源树,研究论文发展脉络
Chat Paper
正在生成论文摘要