Improving CXR Self-Supervised Representation by Pretext Task and Cross-Domain Synthetic Data
Smart innovation, systems and technologies(2023)
摘要
Supervised deep learning techniques have facilitated Chest X-ray (CXR) classification. Transfer learning from ImageNet pre-trained weights has become a common practice in medical image analysis. However, it may be suboptimal in the CXR setting because of distribution disparity, reducing the quality of the learned representations. On the other hand, mining deep features from the non-annotated image using self-supervised learning has great potential for medical tasks. This paper studies the influence of the self-supervised pretext task and non-annotated data amount for CXR self-supervised representation learning. We design a domain-specific self-supervised pretext task in the form of a data augmentation pipeline and explore the feasibility of using CXR and even computerized tomography (CT) volumes to expand the non-annotated CXR database. We verify our method on two state-of-the-art (SOTA) self-supervised architectures, BYOL and SimSiam, and report the results on two public datasets, Xray14 and COVID-QU-Ex. Our main findings are (1) XR-Augment, the proposed data augmentation, outperforms its counterparts in SOTA architectures on the CXR datasets; (2) based on cross-domain ImageNet pre-trained weights, self-supervised learning with XR-Augment further improves the discriminability of model weights on the CXR, and more non-annotated CXR enlarges this advantage; and (3) the synthesized pseudo-CXR, generated from CT, also helps in the context of CXR self-supervised learning.
更多查看译文
关键词
pretext task,data,representation,self-supervised,cross-domain
AI 理解论文
溯源树
样例
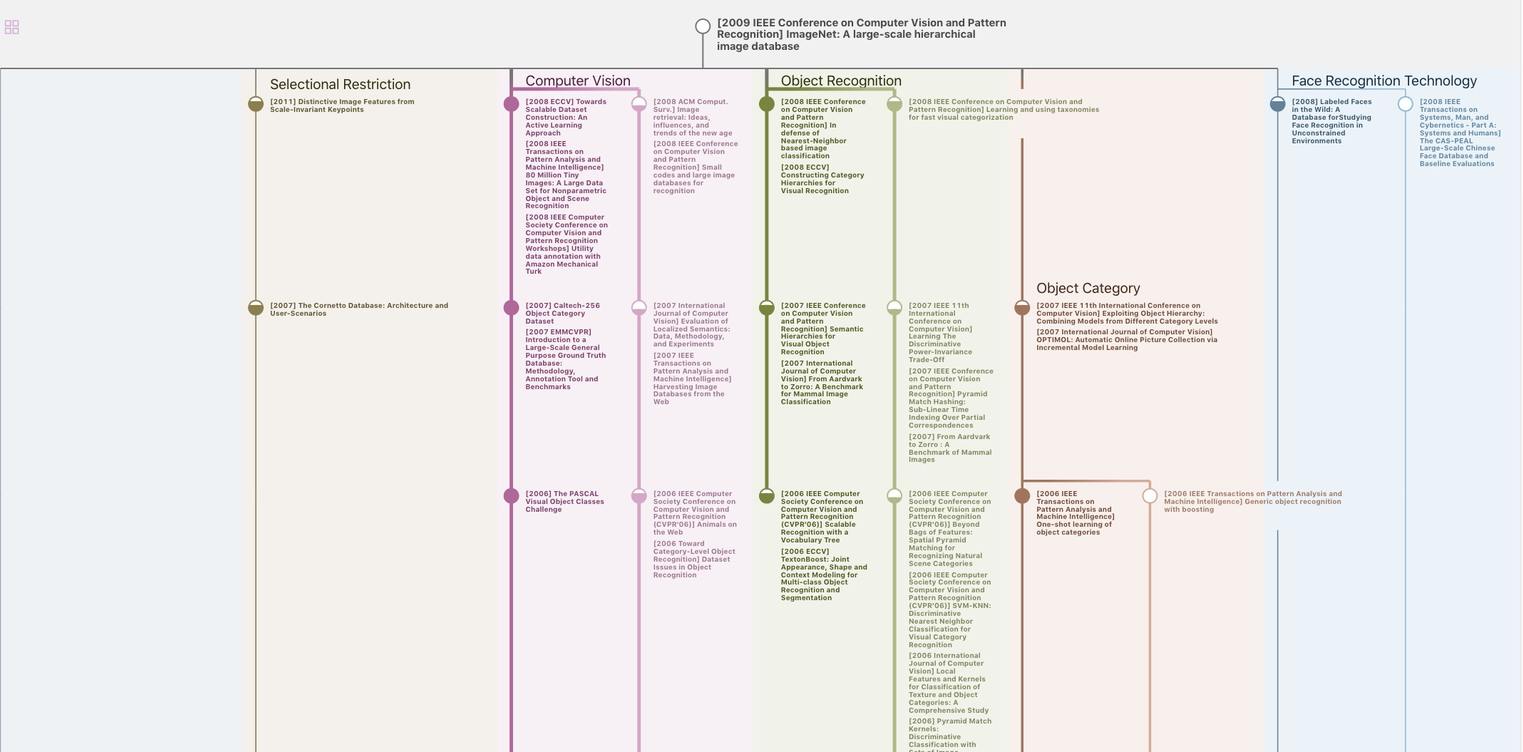
生成溯源树,研究论文发展脉络
Chat Paper
正在生成论文摘要