Safety Assurance of Autonomous Systems using Machine Learning: An Industrial Case Study and Lessons Learnt
INCOSE International Symposium(2023)
摘要
Abstract In order to assess AI/ML‐based autonomous systems in terms of safety, it is not sufficient to assess the system w.r.t. potential failures that could lead to hazards (e.g., as proposed by standards such as IEC 61508, ARP 4761, etc.). Also, functional weaknesses/insufficiencies of the used algorithms according to Safety Of The Intended Functionality (SOTIF) standard ISO 21448 must be considered. In this paper, we present an approach for the safety assessment of systems incorporating AI/ML models using a Model‐based Systems Engineering (MBSE) and a Model‐based Safety Assurance (MBSA) approach. Therefore, we introduce with Component Fault and Deficiency Trees (CFDTs) an extension of the model‐based Component Fault Tree (CFT) methodology. Thereby, we are able to describe cause‐effect relationships between individual failures and functional insufficiencies as well as system hazards and assess if all risks are mitigated. In this paper, we apply our approach to an industrial case study of a self‐driving toy vehicle (the PANORover) and present our lessons learnt.
更多查看译文
关键词
autonomous systems,safety,machine learning,industrial case study
AI 理解论文
溯源树
样例
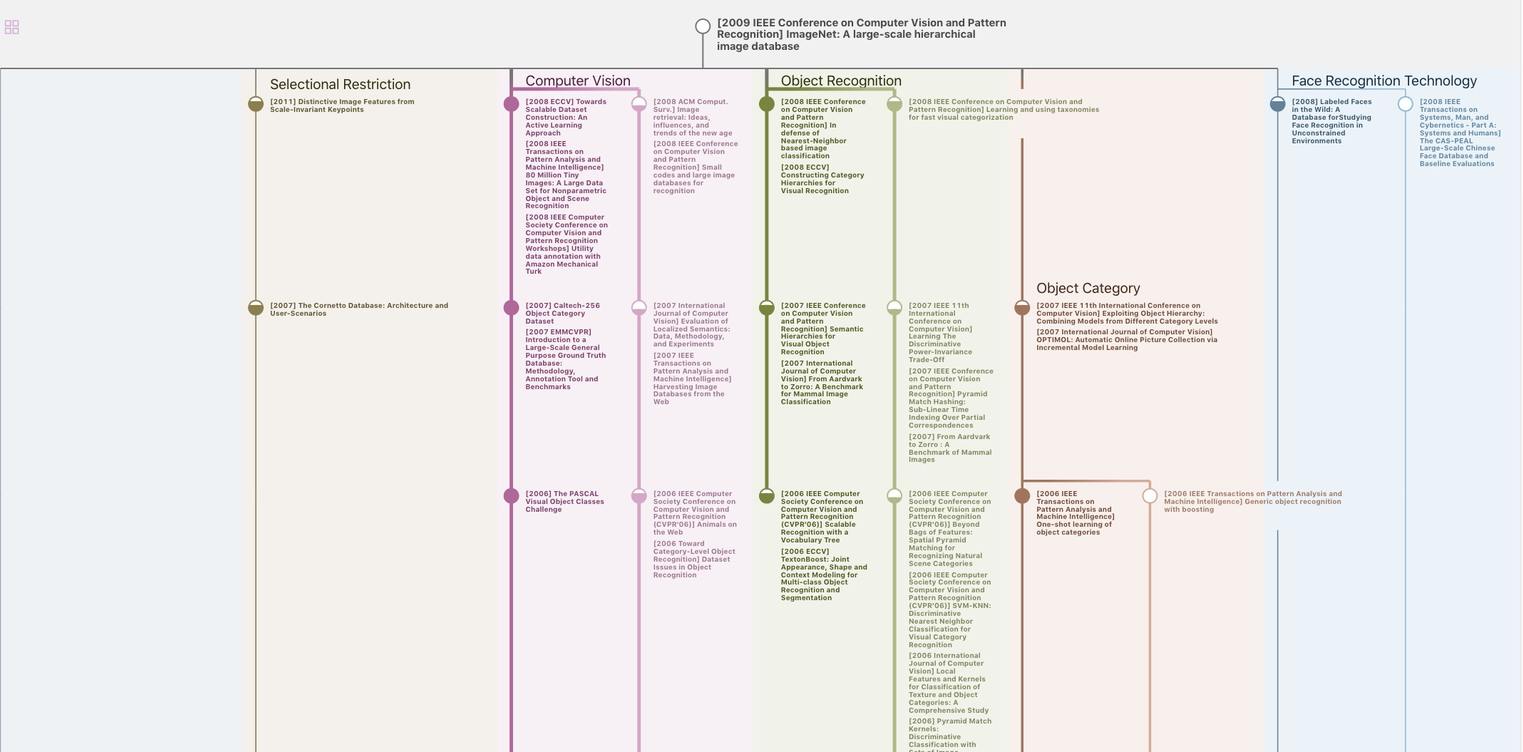
生成溯源树,研究论文发展脉络
Chat Paper
正在生成论文摘要