A Data Model for Predictive Supply Chain Risk Management
Lecture notes in mechanical engineering(2023)
摘要
Nowadays, supply chainsSupply Chain have recently shown to be more prone than ever to disruption. Predicting possible future risks has thus become necessary. In this context, artificial intelligenceArtificial Intelligence as a pillar of the Industry 4.0Industry 4.0 paradigm has proven to deal well with supply chainSupply Chain-related problems. In particular, supervised machine learningMachine learning tools have shown good predictive capabilities, but they require structured data to work well. While several data models have been proposed in the literature, no data model for supply chain risk managementSupply chain risk management has been found. This paper thus aims to propose a new data model to support supply chainSupply Chain risk-related predictions and evaluate this data model's contribution to enhancing models prediction performance. Following a dimensional fact model formalism (DFM), a conceptual model has been first developed and then has been translated to its respective logic version. Once the data model has been built, the problem of predicting the transport risk for a mechanical component delivered to an automotive OEM has been investigated. The predictive performance of a naïve forecasting model and of two long short-term memory (LSTM) models trained with different data have been compared. Results have shown the better performance of LSTM models against the traditional ones. In addition, the LSTM model built with the support of our data model has shown greater forecasting capabilities compared to the ones relying only on past observation of the variable to predict.
更多查看译文
关键词
risk,data model,chain,management
AI 理解论文
溯源树
样例
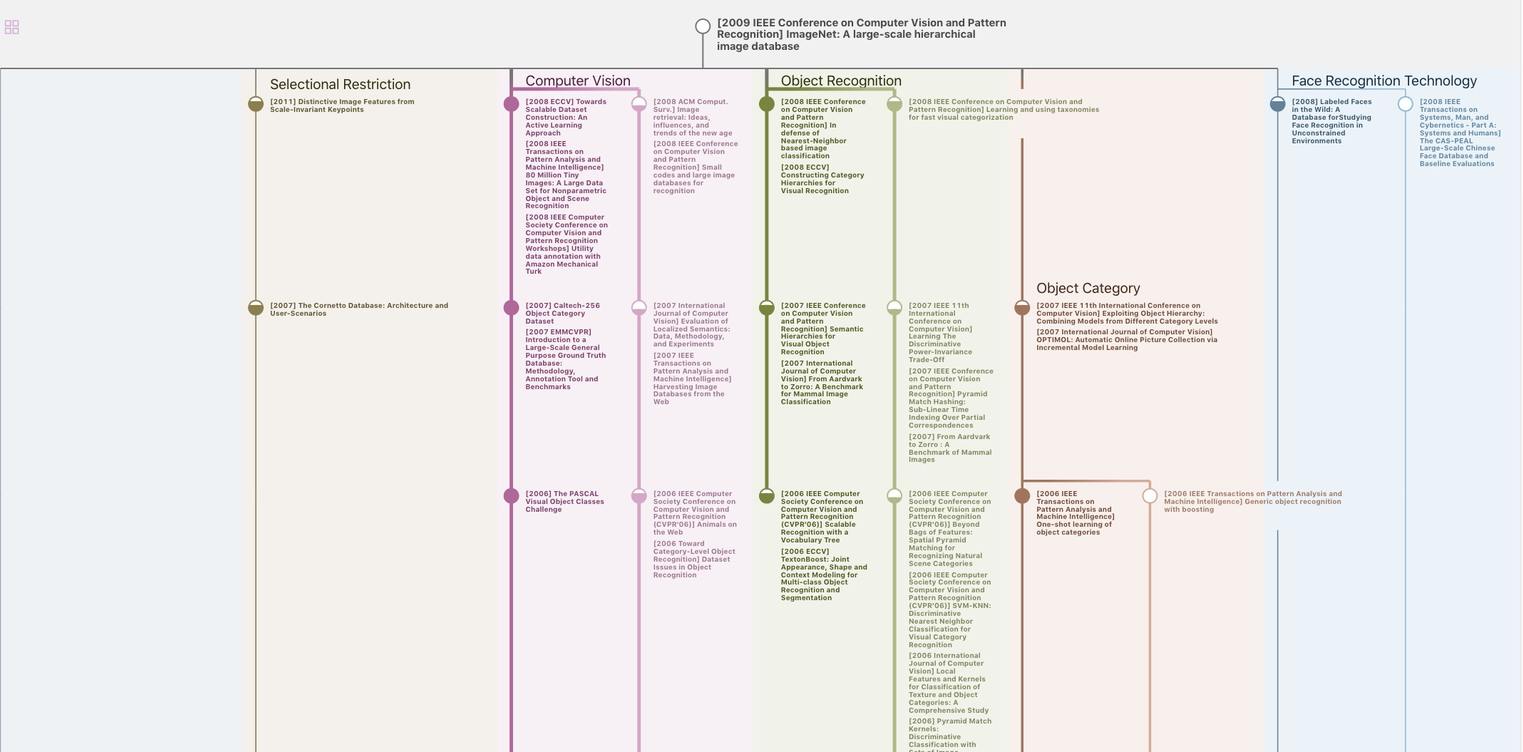
生成溯源树,研究论文发展脉络
Chat Paper
正在生成论文摘要