A Bregman Proximal Perspective on Classical and Quantum Blahut-Arimoto Algorithms
arXiv (Cornell University)(2023)
摘要
The Blahut-Arimoto algorithm is a well-known method to compute classical channel capacities and rate-distortion functions. Recent works have extended this algorithm to compute various quantum analogs of these quantities. In this paper, we show how these Blahut-Arimoto algorithms are special instances of mirror descent, which is a type of Bregman proximal method, and a well-studied generalization of gradient descent for constrained convex optimization. Using recently developed convex analysis tools, we show how analysis based on relative smoothness and strong convexity recovers known sublinear and linear convergence rates for Blahut-Arimoto algorithms. This Bregman proximal viewpoint allows us to derive related algorithms with similar convergence guarantees to solve problems in information theory for which Blahut-Arimoto-type algorithms are not directly applicable. We apply this framework to compute energy-constrained classical and quantum channel capacities, classical and quantum rate-distortion functions, and approximations of the relative entropy of entanglement, all with provable convergence guarantees.
更多查看译文
关键词
mirror descent perspective,quantum,algorithms,blahut-arimoto
AI 理解论文
溯源树
样例
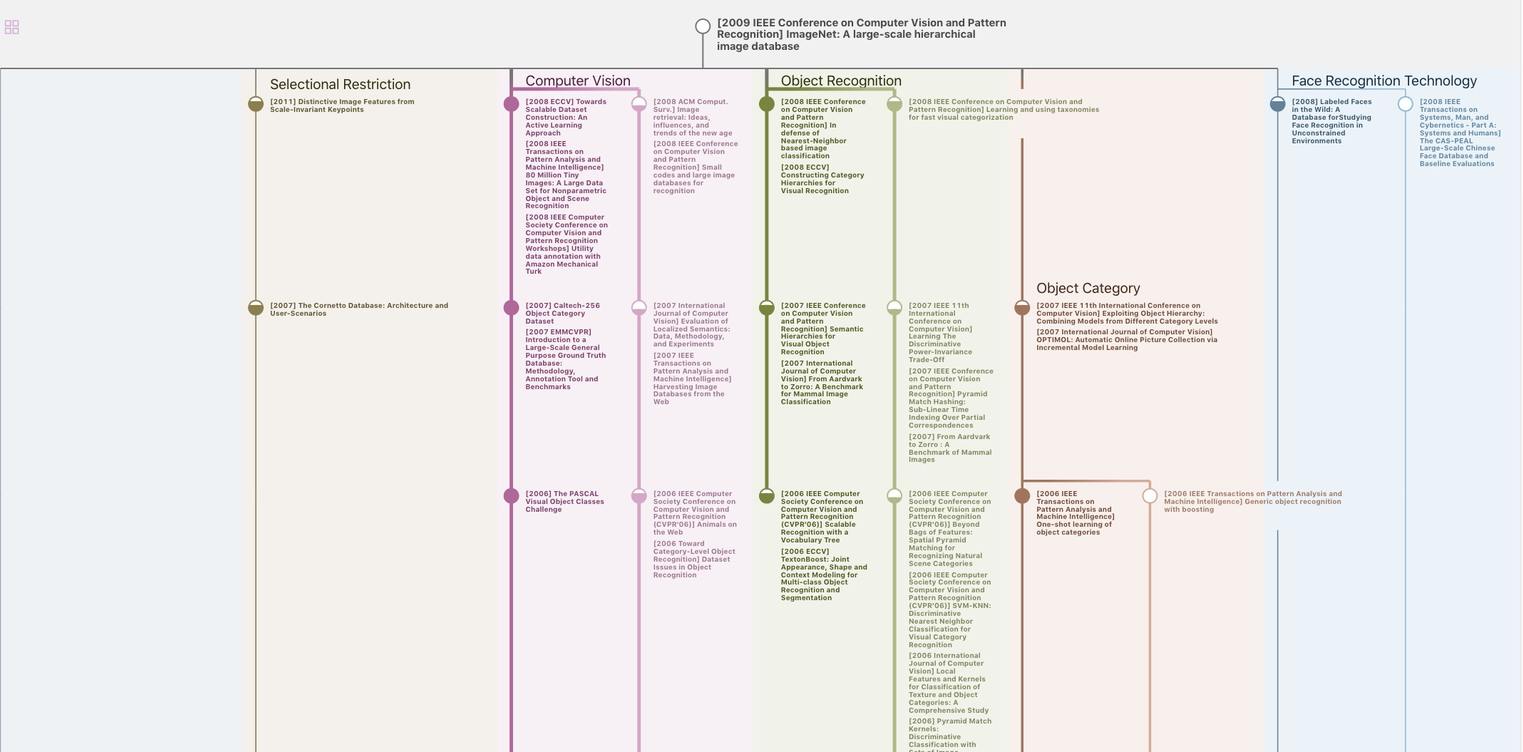
生成溯源树,研究论文发展脉络
Chat Paper
正在生成论文摘要