Recovering Generalization via Pre-Training-Like Knowledge Distillation for Out-of-Distribution Visual Question Answering
IEEE TRANSACTIONS ON MULTIMEDIA(2024)
摘要
With the emergence of large-scale multi-modal foundation models, significant improvements have been made towards Visual Question Answering (VQA) in recent years via the "Pre-training and Fine-tuning" paradigm. However, the fine-tuned VQA model, which is more specialized for the downstream training data, may fail to generalize well when there is a distribution shift between the training and test data, which is defined as the Out-of-Distribution (OOD) problem. An intuitive way to solve this problem is to transfer the common knowledge from the foundation model to the fine-tuned VQA model via knowledge distillation for better generalization. However, the generality of distilled knowledge based on the task-specific training data is questionable due to the bias between the training and test data. An ideal way is to adopt the pre-training data to distill the common knowledge shared by the training and OOD test samples, which however is impracticable due to the huge size of pre-training data. Based on the above considerations, in this article, we propose a method, named Pre-training-like Knowledge Distillation (PKD), to imitate the pre-training feature distribution and leverage it to distill the common knowledge, which can improve the generalization performance of the fine-tuned model for OOD VQA. Specifically, we first leverage the in-domain VQA data as guidance and adopt two cross-modal feature prediction networks, which are learned under the supervision of image-text matching loss and feature divergence loss, to estimate pre-training-like vision and text features. Next, we conduct feature-level distillation by explicitly integrating the downstream VQA input features with the predicted pre-training-like features through a memory mechanism. In the meantime, we also conduct model-level distillation by constraining the image-text matching output of the downstream VQA model and the output of the foundation model for the pre-training-like image and text features. Extensive experiments on the VQA-CP v2 and VQA v2 datasets demonstrate the effectiveness of our method.
更多查看译文
关键词
Multi-modal Foundation Model,Out-of-Distribution Generalization,Visual Question Answering,Knowledge Distillation
AI 理解论文
溯源树
样例
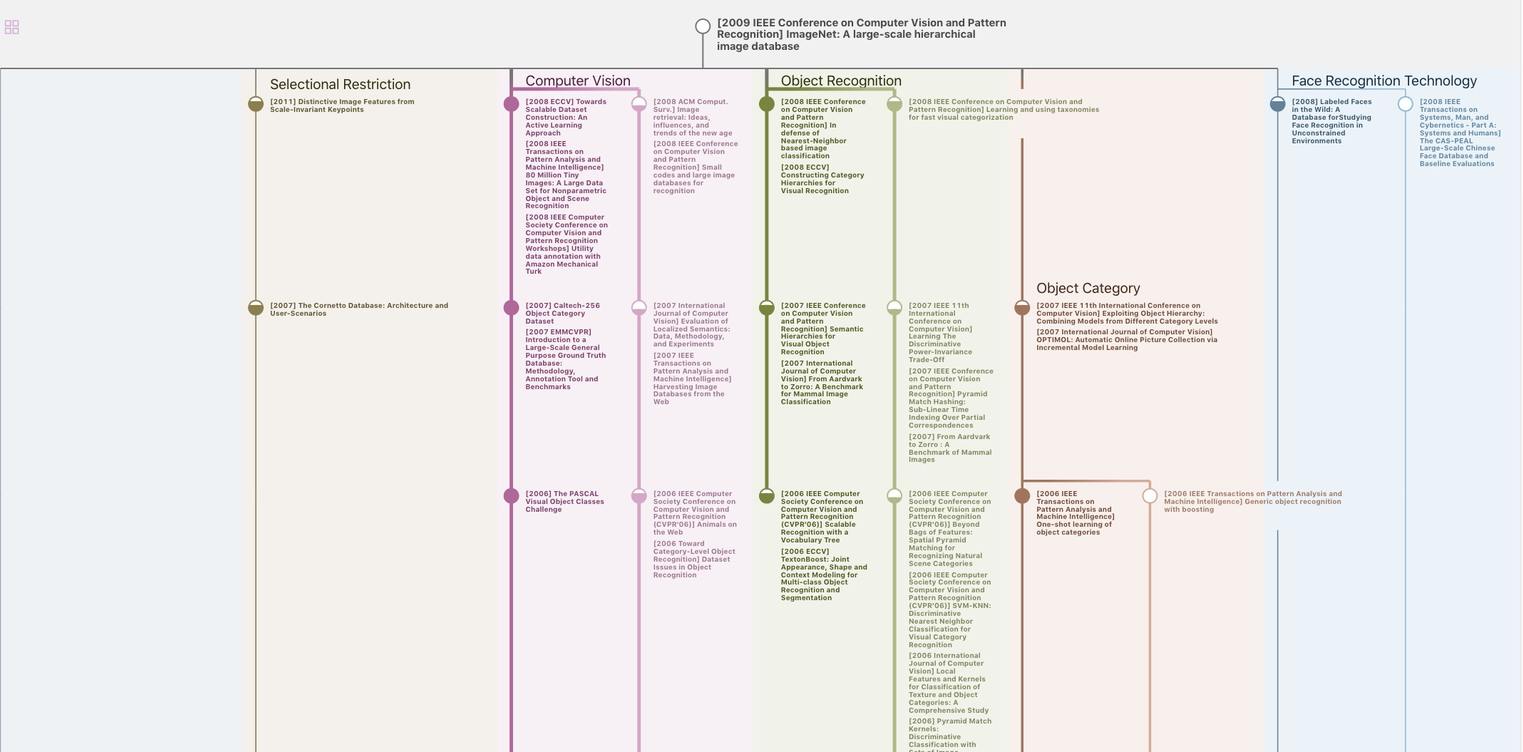
生成溯源树,研究论文发展脉络
Chat Paper
正在生成论文摘要