113 The Use of Machine Learning of Carotid Web Angioarchitecture for Predicting Stroke Risk
Neurosurgery(2023)
摘要
INTRODUCTION: Carotid webs (CWs) are a known mechanism for stroke in young adults without other vascular risk factors. Altered hemodynamics distal to the web may cause flow stagnation with the potential for distal embolization of clots. Although there are some computational flow models studies of CW, currently there are no well-described CT angiographic features to predict stroke risk. METHODS: Anatomical data of the internal carotid artery (ICA), common carotid artery (CCA), the CW and its “distal pouch” angioarchitecture was gathered as part of a retrospective cohort study from symptomatic (stroke) and asymptomatic (non-stroke) patients. Principal component analysis was applied to anatomical and angioarchitectural features, and the two components were plotted to investigate whether the stroke and non-stroke patients were easily separable. We built a predictive model of stroke risk using these features with Firth regression to reduce bias related to small datasets. RESULTS: The first two principal components of the anatomical and angioarchitectural features clearly distinguish between stroke patients and non-stroke patients. The firth regression and 5-fold cross validation revealed that CCA web “pouch” angle (p=.014), ICA web “pouch” tip angle (p=.023), CCA web “pouch” tip angle (p = .017), and ICA web “pouch” angle (p = .016) are the most important features to model and assess stroke risk. Conversely, CCA and ICA anatomy (diameter and angle) on their own were not found to be risk factors for stroke. Overall, pouch angles in respect to flow trajectory in the CCA and in the ICA are significant risk factors as compared to vessel sizes and flow relationships between them. CONCLUSIONS: This study demonstrates that carotid web angioarchitecture and carotid anatomy data from diagnostic angiography may be used to assess stroke risk, allowing physicians to tailor care for each patient according to risk stratification.
更多查看译文
关键词
carotid web angioarchitecture,stroke,machine learning
AI 理解论文
溯源树
样例
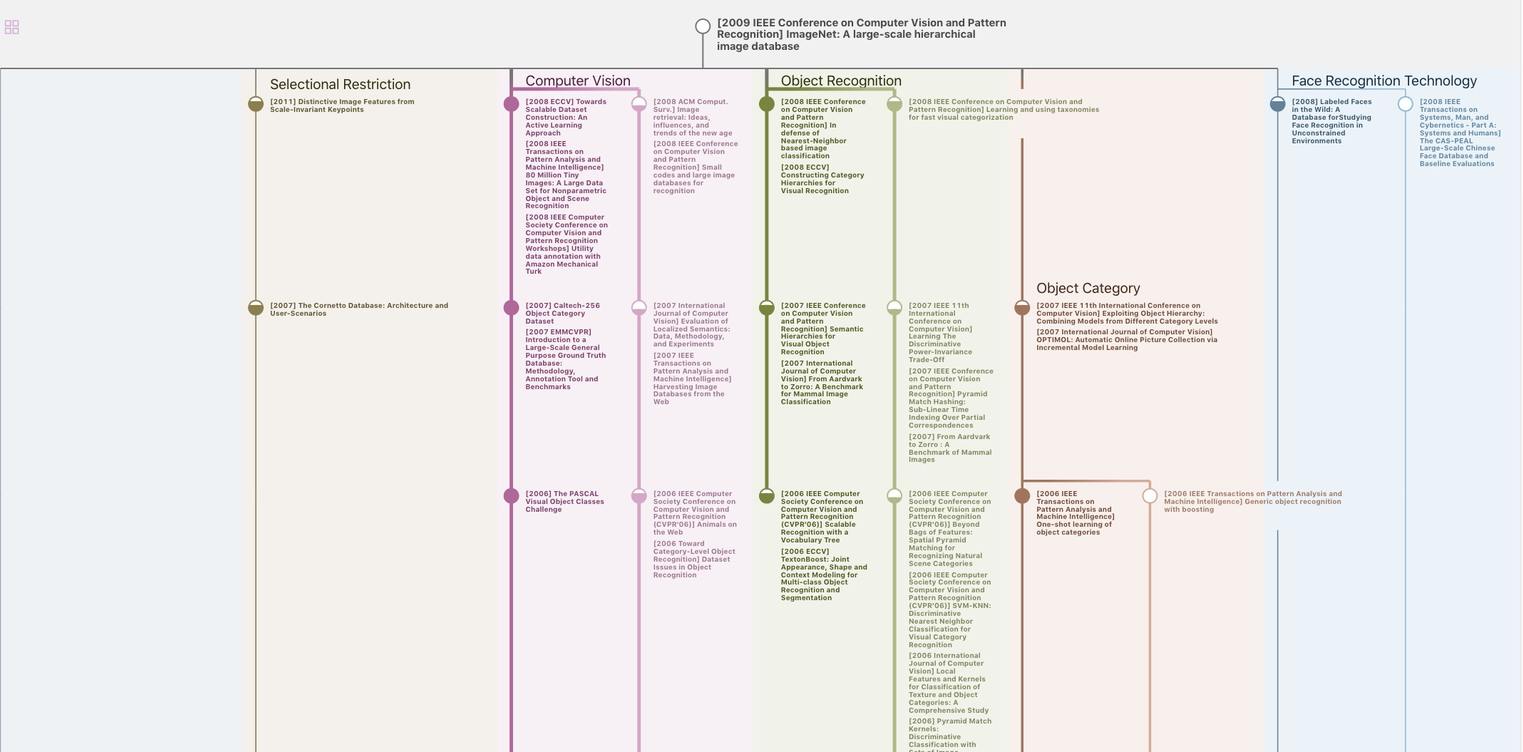
生成溯源树,研究论文发展脉络
Chat Paper
正在生成论文摘要