Specific Emitter Identification Based on ACO-XGBoost Feature Selection
Lecture Notes in Computer Science(2023)
摘要
In order to improve the performance of Specific Emitter Identification (SEI) in complex electromagnetic environment, a SEI model based on Ant Colony Optimization-eXtreme Gradient Boosting (ACO-XGBoost) feature selection is proposed. Firstly, lifting wavelet package transformation is introduced to extract features of original emitter signals and data normalization is carried out on the data. Secondly, ACO is used to optimize the main parameters for XGBoost and the optimized XGBoost algorithm is applied to score the importance of the features. Thirdly, the importance of each feature is used as threshold to determine the best feature subset. Finally, combined with XGBoost classifier, specific emitter has been correctly identified. The proposed model is investigated on three datasets and the experimental result shows that the optimal feature subset can be effectively acquired and reasonably classified by the proposed model. Comparing with Decision Tree (DT), Random Forest (RF), Gradient Boosting Decision Tree (GBDT) and XGBoost feature selection methods, the identification performance of the proposed model is effectively improved.
更多查看译文
关键词
specific emitter identification,selection,feature,aco-xgboost
AI 理解论文
溯源树
样例
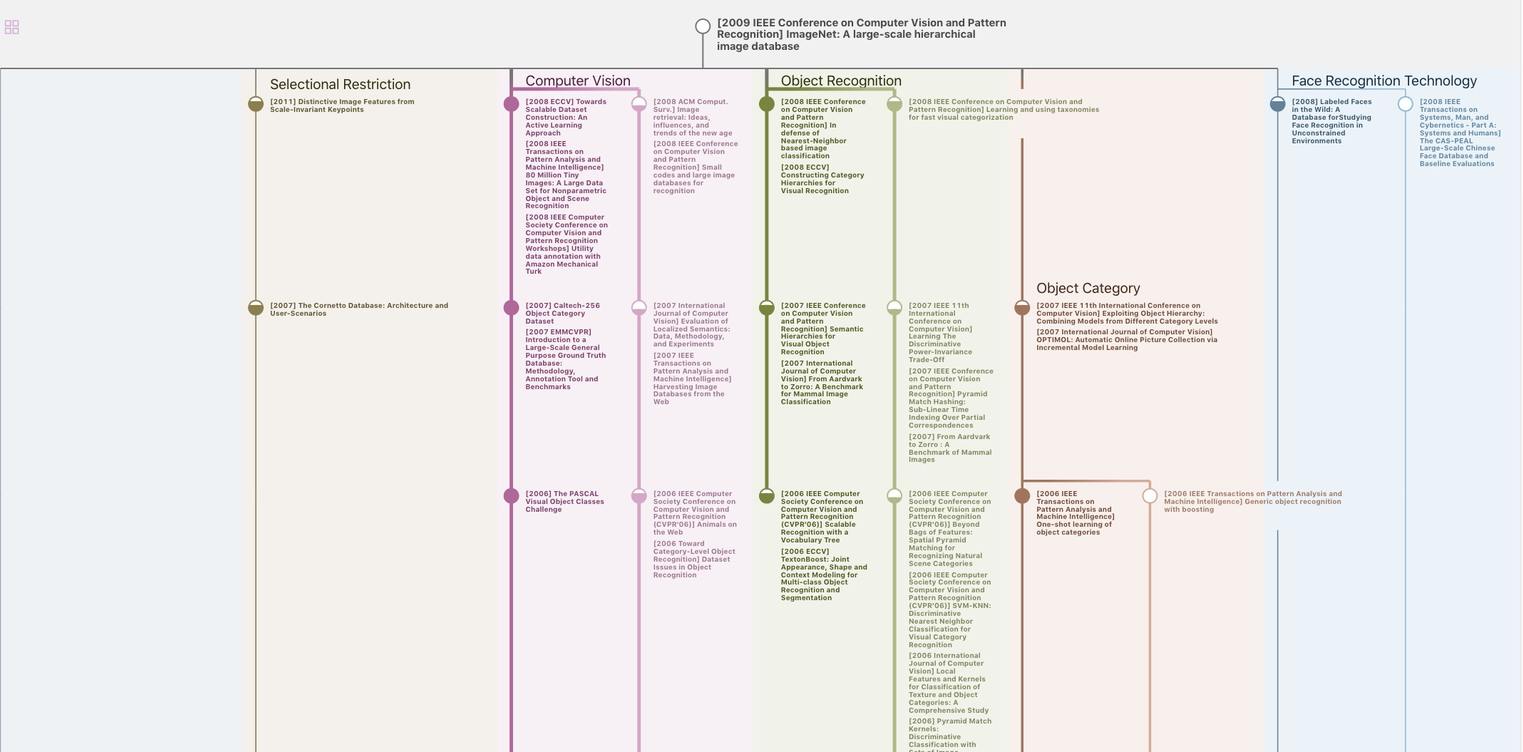
生成溯源树,研究论文发展脉络
Chat Paper
正在生成论文摘要