A Bayesian network learning method for sparse and unbalanced data with GNN-based multilabel classification application
APPLIED SOFT COMPUTING(2024)
摘要
Creating a well-defined Bayesian network (BN) is helpful for developing effective graph neural networks (GNNs) that exploit annotated labels in multilabel classification (MLC) tasks. Obtaining correct BNs can be challenging when the labels are highly unbalanced and sparse. This study proposes a novel scoring method to address data imbalance and sparsity by introducing an imbalanced weight term. Comparison results show that the BNs learned from the proposed scoring method are simpler and more accurate than the well-known methods. The obtained BNs based on the proposed method are used to construct GNNs for MLC tasks based on three datasets. The findings suggest that the adjacency matrices based on moral graphs of BNs exhibit more excellent stability than those derived from mathematical approaches. By selecting the parameter thoughtfully, GNNs based on the proposed BDsp scores showcase up to 12% classification performance improvement compared to GNNs based on existing Bayesian scores.
更多查看译文
关键词
Bayesian network,Structure learning,Imbalance data,Sparse data,Graph neural network
AI 理解论文
溯源树
样例
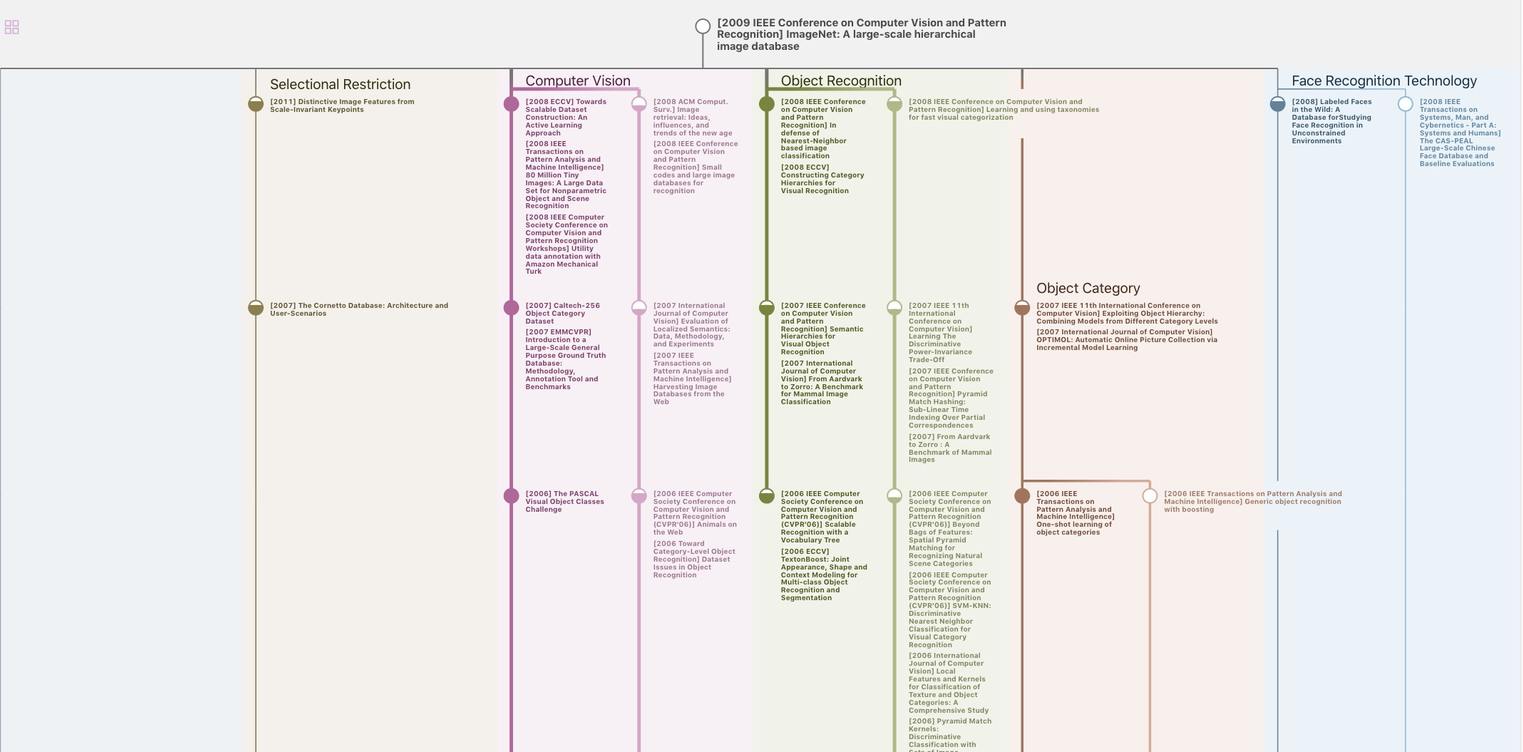
生成溯源树,研究论文发展脉络
Chat Paper
正在生成论文摘要