Dynamics and Inference for Voter Model Processes
arXiv (Cornell University)(2022)
摘要
We consider a discrete-time voter model process on a set of nodes, each being in one of two states, either 0 or 1. In each time step, each node adopts the state of a randomly sampled neighbor according to sampling probabilities, referred to as node interaction parameters. We study the maximum likelihood estimation of the node interaction parameters from observed node states for a given number of realizations of the voter model process. In contrast to previous work on parameter estimation of network autoregressive processes, whose long-run behavior is according to a stationary stochastic process, the voter model is an absorbing stochastic process that eventually reaches a consensus state. This requires developing a framework for deriving parameter estimation error bounds from observations consisting of several realizations of a voter model process. We present parameter estimation error bounds by interpreting the observation data as being generated according to an extended voter process that consists of cycles, each corresponding to a realization of the voter model process until absorption to a consensus state. In order to obtain these results, consensus time of a voter model process plays an important role. We present new bounds for all moments and a bound that holds with any given probability for consensus time, which may be of independent interest. In contrast to most existing work, our results yield a consensus time bound that holds with high probability. We also present a sampling complexity lower bound for parameter estimation within a prescribed error tolerance for the class of locally stable estimators.
更多查看译文
关键词
inference,model,dynamics,processes
AI 理解论文
溯源树
样例
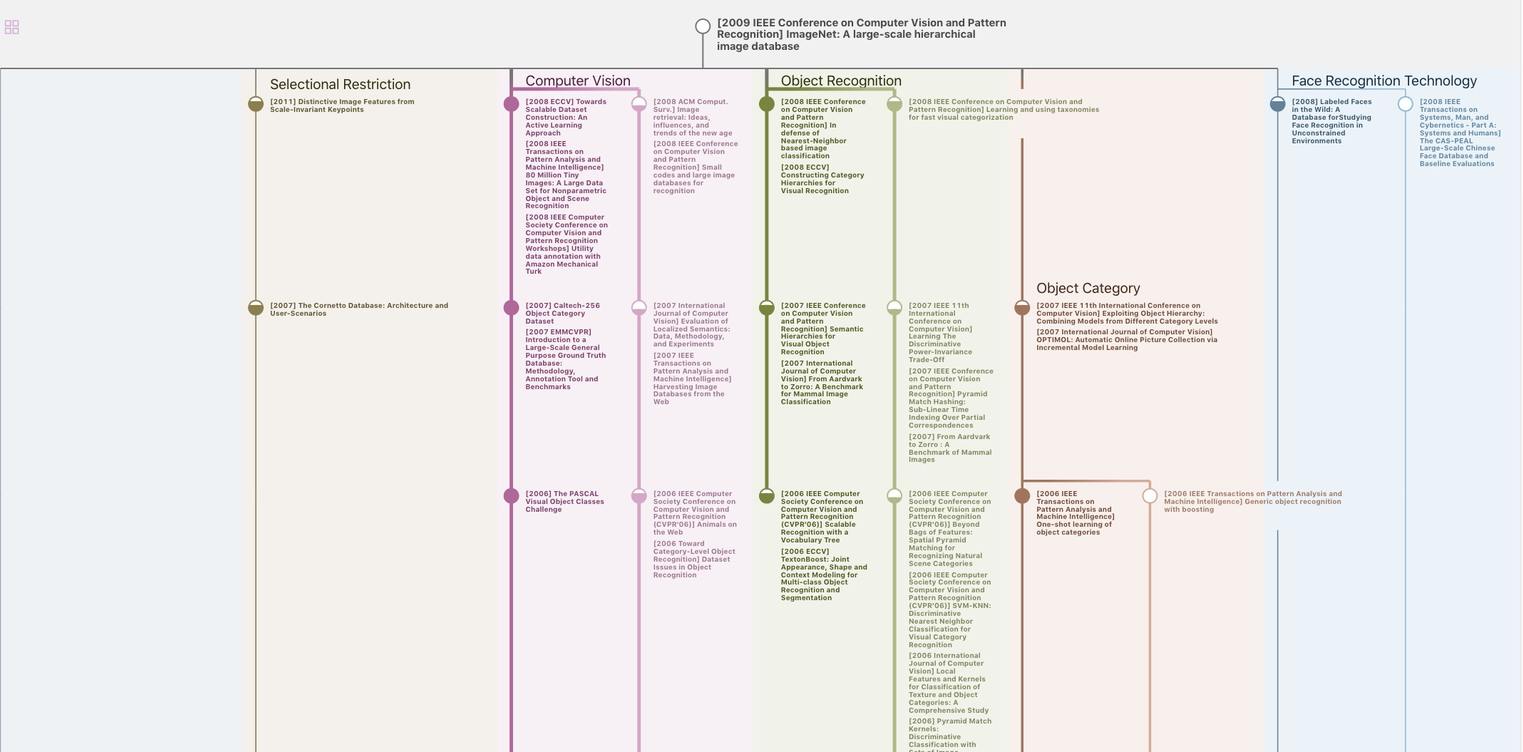
生成溯源树,研究论文发展脉络
Chat Paper
正在生成论文摘要