Not All Points Are Equal: Learning Highly Efficient Point-based Detectors for 3D LiDAR Point Clouds
arXiv (Cornell University)(2022)
摘要
We study the problem of efficient object detection of 3D LiDAR point clouds. To reduce the memory and computational cost, existing point-based pipelines usually adopt task-agnostic random sampling or farthest point sampling to progressively downsample input point clouds, despite the fact that not all points are equally important to the task of object detection. In particular, the foreground points are inherently more important than background points for object detectors. Motivated by this, we propose a highly-efficient single-stage point-based 3D detector in this paper, termed IA-SSD. The key of our approach is to exploit two learnable, task-oriented, instance-aware downsampling strategies to hierarchically select the foreground points belonging to objects of interest. Additionally, we also introduce a contextual centroid perception module to further estimate precise instance centers. Finally, we build our IA-SSD following the encoder-only architecture for efficiency. Extensive experiments conducted on several large-scale detection benchmarks demonstrate the competitive performance of our IA-SSD. Thanks to the low memory footprint and a high degree of parallelism, it achieves a superior speed of 80+ frames-per-second on the KITTI dataset with a single RTX2080Ti GPU. The code is available at \url{https://github.com/yifanzhang713/IA-SSD}.
更多查看译文
关键词
points,detectors,3d,point-based
AI 理解论文
溯源树
样例
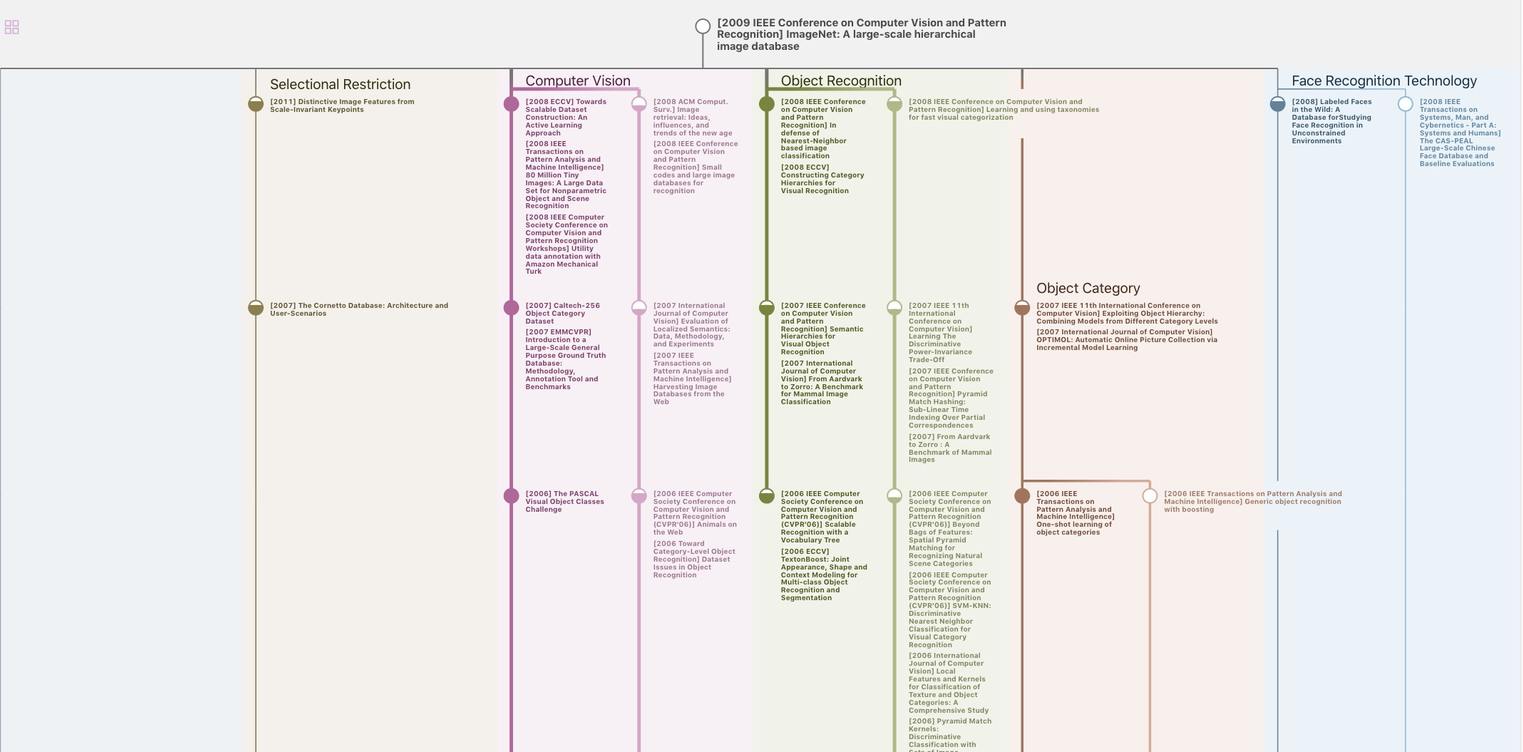
生成溯源树,研究论文发展脉络
Chat Paper
正在生成论文摘要